


ChatGLM, the Tsinghua-based Gigabit base conversation model, has launched internal testing and is an open source single-card version of the model.
The release of ChatGPT has stirred up the entire AI field, and major technology companies, startups, and university teams are following suit. Recently, Heart of the Machine has reported on the research results of many startup companies and university teams.
Yesterday, another large-scale domestic AI dialogue model made its grand debut: ChatGLM, a company’s smart spectrum AI transformed from Tsinghua’s technological achievements and based on the GLM-130B 100 billion base model, is now open for invitations Internal testing.
It is worth mentioning that Zhipu AI has also open sourced the Chinese-English bilingual dialogue model ChatGLM-6B, which supports inference on a single consumer-grade graphics card.
#Internal beta application website: chatglm.cn
It is understood that the capability improvement of the current version of ChatGLM model mainly comes from the unique 100 billion base model GLM-130B. It is an architecture different from BERT, GPT-3 and T5. It is an autoregressive pre-training model containing multi-objective functions.
In August 2022, Tsinghua University and Zhipu AI opened the Chinese-English bilingual dense model GLM-130B with 130 billion parameters to the research community and industry. This model has some unique features Advantages:
- Bilingual: Supports both Chinese and English;
- High precision (English): in the public English natural language Better than GPT-3 175B (API: davinci, base model), OPT-175B and BLOOM-176B on the list of LAMBADA, MMLU and Big-bench-lite;
- High accuracy (Chinese): Significantly better than ERNIE TITAN 3.0 260B and YUAN 1.0-245B on 7 zero-sample CLUE data sets and 5 zero-sample FewCLUE data sets;
- Fast inference: First A 100 billion model that implements INT4 quantification, supporting fast and basically lossless inference with a 4-card 3090 or 8-card 2080Ti server;
- Reproducibility: all results (more than 30 Each task) can be reproduced through our open source code and model parameters;
- Cross-platform: supports domestic Haiguang DCU, Huawei Ascend 910 and Sunway processors and the United States Training and inference on NVIDIA chips.
Now, referring to the design ideas of ChatGPT, ChatGLM has injected code pre-training into the GLM-130B base model, through supervised fine-tuning (Supervised Fine-Tuning) and other technologies to achieve alignment of human intentions.
Heart of the Machine has obtained the internal beta invitation code. Here is a simple conversation with ChatGLM. The effect is as follows:
It can understand the actual meaning of "station CP":
Give ChatGLM a math problem to try:
Since I learned the linear equation of two variables, basic "chicken and rabbit in the same cage" problems like this can no longer trouble it:
Open Source ChatGLM-6B
ChatGLM-6B is an open source dialogue language model that supports bilingual question and answer in Chinese and English and is optimized for Chinese. The model is based on the General Language Model (GLM) architecture and has 6.2 billion parameters. Combined with model quantization technology, users can deploy it locally on consumer-grade graphics cards (a minimum of 6GB of video memory is required at the INT4 quantization level). ChatGLM-6B uses the same technology as ChatGLM and is optimized for Chinese Q&A and dialogue. After bilingual training in Chinese and English with about 1T identifiers, supplemented by supervised fine-tuning, feedback self-service, human feedback reinforcement learning and other technologies, the 6.2 billion parameter ChatGLM-6B, although not as large as the 100 billion model, has greatly reduced the inference cost and improved It has improved efficiency and can already generate answers that are quite consistent with human preferences.
Model open source address: https://github.com/THUDM/ChatGLM-6B
Specifically , ChatGLM-6B has the following characteristics:
- # Sufficient bilingual pre-training in Chinese and English: ChatGLM-6B has trained a token amount of 1T on Chinese and English materials in a 1:1 ratio. , both bilingual.
- Optimized model architecture and size: Drawing on GLM-130B training experience, the two-dimensional RoPE position encoding implementation is revised, using the traditional FFN structure. The parameter size of 6B (6.2 billion) also makes it possible for researchers and individual developers to fine-tune and deploy ChatGLM-6B themselves.
- Lower deployment threshold: At FP16 half precision, ChatGLM-6B requires at least 13 GB of video memory for inference. Combined with model quantization technology, this requirement can be further reduced to 10GB (INT8 ) and 6GB (INT4), allowing ChatGLM-6B to be deployed on consumer-grade graphics cards.
- Longer sequence length: Compared with GLM-10B (sequence length 1024), ChatGLM-6B has a sequence length of 2048, supporting longer conversations and applications.
- Human intention alignment training: Supervised Fine-Tuning, Feedback Bootstrap, Reinforcement Learning from Human Feedback and other methods are used to make The model is beginning to have the ability to understand the intent of human instructions. The output format is markdown for easy display.
However, due to the small capacity of the ChatGLM-6B model, there are inevitably some limitations and shortcomings, including:
- Relatively weak model memory and language capabilities. ChatGLM-6B may generate incorrect information when faced with many factual knowledge tasks, and it is not very good at solving logical problems (such as mathematics, programming).
- May generate harmful illustrations or biased content: ChatGLM-6B is only a preliminary language model aligned with human intent and may generate harmful or biased content.
- Weak multi-turn dialogue capability: ChatGLM-6B’s context understanding capability is not sufficient, and context loss may occur when faced with long answer generation and multi-turn dialogue scenarios. and misunderstanding situations.
#The GLM team stated that ChatGLM is still far behind the world's top large model research and products. In the future, it will continue to develop and open source updated versions of ChatGLM and related models. The GLM team also welcomes everyone to download ChatGLM-6B and conduct research and (non-commercial) application development based on it.
The above is the detailed content of ChatGLM, the Tsinghua-based Gigabit base conversation model, has launched internal testing and is an open source single-card version of the model.. For more information, please follow other related articles on the PHP Chinese website!

Hot AI Tools

Undresser.AI Undress
AI-powered app for creating realistic nude photos

AI Clothes Remover
Online AI tool for removing clothes from photos.

Undress AI Tool
Undress images for free

Clothoff.io
AI clothes remover

Video Face Swap
Swap faces in any video effortlessly with our completely free AI face swap tool!

Hot Article

Hot Tools

Notepad++7.3.1
Easy-to-use and free code editor

SublimeText3 Chinese version
Chinese version, very easy to use

Zend Studio 13.0.1
Powerful PHP integrated development environment

Dreamweaver CS6
Visual web development tools

SublimeText3 Mac version
God-level code editing software (SublimeText3)

Hot Topics
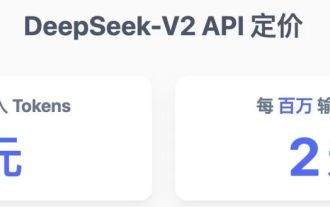
Imagine an artificial intelligence model that not only has the ability to surpass traditional computing, but also achieves more efficient performance at a lower cost. This is not science fiction, DeepSeek-V2[1], the world’s most powerful open source MoE model is here. DeepSeek-V2 is a powerful mixture of experts (MoE) language model with the characteristics of economical training and efficient inference. It consists of 236B parameters, 21B of which are used to activate each marker. Compared with DeepSeek67B, DeepSeek-V2 has stronger performance, while saving 42.5% of training costs, reducing KV cache by 93.3%, and increasing the maximum generation throughput to 5.76 times. DeepSeek is a company exploring general artificial intelligence
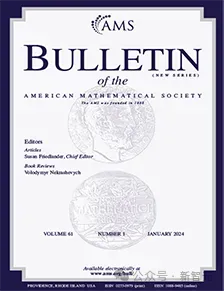
AI is indeed changing mathematics. Recently, Tao Zhexuan, who has been paying close attention to this issue, forwarded the latest issue of "Bulletin of the American Mathematical Society" (Bulletin of the American Mathematical Society). Focusing on the topic "Will machines change mathematics?", many mathematicians expressed their opinions. The whole process was full of sparks, hardcore and exciting. The author has a strong lineup, including Fields Medal winner Akshay Venkatesh, Chinese mathematician Zheng Lejun, NYU computer scientist Ernest Davis and many other well-known scholars in the industry. The world of AI has changed dramatically. You know, many of these articles were submitted a year ago.
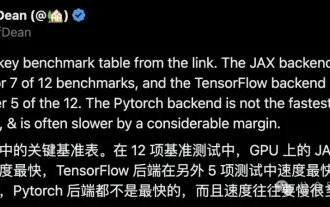
The performance of JAX, promoted by Google, has surpassed that of Pytorch and TensorFlow in recent benchmark tests, ranking first in 7 indicators. And the test was not done on the TPU with the best JAX performance. Although among developers, Pytorch is still more popular than Tensorflow. But in the future, perhaps more large models will be trained and run based on the JAX platform. Models Recently, the Keras team benchmarked three backends (TensorFlow, JAX, PyTorch) with the native PyTorch implementation and Keras2 with TensorFlow. First, they select a set of mainstream
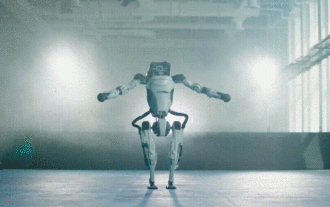
Boston Dynamics Atlas officially enters the era of electric robots! Yesterday, the hydraulic Atlas just "tearfully" withdrew from the stage of history. Today, Boston Dynamics announced that the electric Atlas is on the job. It seems that in the field of commercial humanoid robots, Boston Dynamics is determined to compete with Tesla. After the new video was released, it had already been viewed by more than one million people in just ten hours. The old people leave and new roles appear. This is a historical necessity. There is no doubt that this year is the explosive year of humanoid robots. Netizens commented: The advancement of robots has made this year's opening ceremony look like a human, and the degree of freedom is far greater than that of humans. But is this really not a horror movie? At the beginning of the video, Atlas is lying calmly on the ground, seemingly on his back. What follows is jaw-dropping
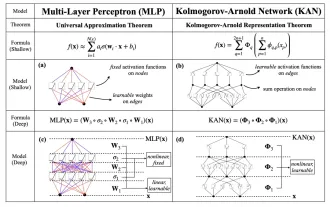
Earlier this month, researchers from MIT and other institutions proposed a very promising alternative to MLP - KAN. KAN outperforms MLP in terms of accuracy and interpretability. And it can outperform MLP running with a larger number of parameters with a very small number of parameters. For example, the authors stated that they used KAN to reproduce DeepMind's results with a smaller network and a higher degree of automation. Specifically, DeepMind's MLP has about 300,000 parameters, while KAN only has about 200 parameters. KAN has a strong mathematical foundation like MLP. MLP is based on the universal approximation theorem, while KAN is based on the Kolmogorov-Arnold representation theorem. As shown in the figure below, KAN has
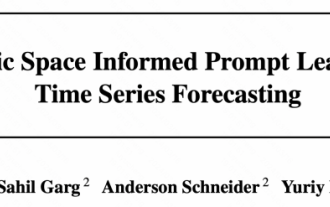
Today I would like to share a recent research work from the University of Connecticut that proposes a method to align time series data with large natural language processing (NLP) models on the latent space to improve the performance of time series forecasting. The key to this method is to use latent spatial hints (prompts) to enhance the accuracy of time series predictions. Paper title: S2IP-LLM: SemanticSpaceInformedPromptLearningwithLLMforTimeSeriesForecasting Download address: https://arxiv.org/pdf/2403.05798v1.pdf 1. Large problem background model
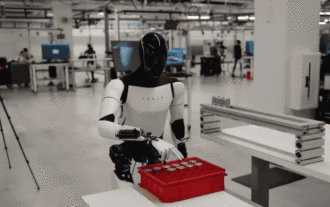
The latest video of Tesla's robot Optimus is released, and it can already work in the factory. At normal speed, it sorts batteries (Tesla's 4680 batteries) like this: The official also released what it looks like at 20x speed - on a small "workstation", picking and picking and picking: This time it is released One of the highlights of the video is that Optimus completes this work in the factory, completely autonomously, without human intervention throughout the process. And from the perspective of Optimus, it can also pick up and place the crooked battery, focusing on automatic error correction: Regarding Optimus's hand, NVIDIA scientist Jim Fan gave a high evaluation: Optimus's hand is the world's five-fingered robot. One of the most dexterous. Its hands are not only tactile
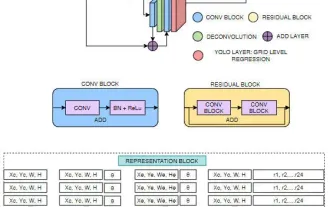
Target detection is a relatively mature problem in autonomous driving systems, among which pedestrian detection is one of the earliest algorithms to be deployed. Very comprehensive research has been carried out in most papers. However, distance perception using fisheye cameras for surround view is relatively less studied. Due to large radial distortion, standard bounding box representation is difficult to implement in fisheye cameras. To alleviate the above description, we explore extended bounding box, ellipse, and general polygon designs into polar/angular representations and define an instance segmentation mIOU metric to analyze these representations. The proposed model fisheyeDetNet with polygonal shape outperforms other models and simultaneously achieves 49.5% mAP on the Valeo fisheye camera dataset for autonomous driving
