


Stanford, Meta AI Research: On the road to AGI, data pruning is more important than we think
In many fields of machine learning, including vision, language, and speech, neural scaling states that test error generally decreases with training data, model size, or number of calculations. This proportional improvement has driven deep learning to achieve substantial performance gains. However, these improvements achieved through scaling alone come at a considerable cost in terms of computation and energy.
This proportional scaling is unsustainable. For example, the amount of data, computation, or energy required to reduce the error from 3% to 2% increases exponentially. Some previous research has shown that reducing the cross-entropy loss from 3.4 to 2.8 in language modeling with large Transformers requires 10 times more training data. Furthermore, for large visual Transformers, an additional 2 billion pre-trained data points (starting from 1 billion) only resulted in an accuracy gain of a few percentage points on ImageNet.
All these results shed light on the nature of data in deep learning, while showing that the practice of collecting huge data sets can be inefficient. The discussion here is whether we can do better. For example, can we achieve exponential scaling with a good strategy for selecting training samples?
In a recent article, researchers found that only adding a few carefully selected training samples can reduce the error from 3% to 2% without having to collect 10 times more random samples. sample. In short, "Sale is not all you need".
Paper link: https://arxiv.org/pdf/2206.14486.pdf
Overall, the contribution of this research is:
1. Using statistical mechanics, a new data pruning analysis theory is developed. In the teacher-student perceptron learning environment, samples are pruned according to their teacher margins, and the large (small) margins each correspond to a simple ( Difficulty) sample. The theory agrees quantitatively with numerical experiments and reveals two surprising predictions:
a. The optimal pruning strategy changes with the amount of initial data; if the initial data is abundant (scarce), then Only difficult (easy) samples should be retained.
b. If an increasing Pareto-optimal pruning score is chosen as a function of the initial dataset size, then exponential scaling is possible for the pruned dataset size.
#2. Research shows that these two predictions hold true in practice in more general settings. They validate the error exponential scaling characteristics with respect to pruned dataset size for ResNets trained from scratch on SVHN, CIFAR-10, and ImageNet, and for a visual Transformer fine-tuned on CIFAR-10.
3. Conducted a large-scale benchmarking study on 10 different data pruning metrics on ImageNet and found that most of the metrics performed poorly except for the most computationally intensive ones.
4. A new low-cost unsupervised pruning metric is developed using self-supervised learning, which, unlike previous metrics, does not require labels. We demonstrate that this unsupervised measure is comparable to the best supervised pruning measures, which require labels and more computation. This result reveals the possibility of using a pre-trained base model to prune new datasets.
Is scale all you need?
The researcher's perceptron data pruning theory proposes three surprising predictions, which can be tested in a more general environment, such as deep neural networks trained on benchmarks:
( 1) Compared with random data pruning, when the initial data set is relatively large, it is beneficial to retain only the most difficult samples, but when the initial data set is relatively small, this is harmful;
(2) With As the initial data set size increases, data pruning by retaining a fixed fraction f of the hardest samples should produce a power-law scaling with an exponent equal to random pruning;
(3) When the initial data set size and all Optimizing the test error on the fraction of retained data can be achieved by performing more aggressive pruning on a larger initial data set, tracking a Pareto-optimal lower envelope, breaking the relationship between test error and pruned data set size. The power law scaling function relationship between
The researchers used different numbers of initial data set sizes and data fractions saved under data pruning (the theory in Figure 3A vs. the deep learning experiment in Figure 3BCD), ResNets trained on SVHN, CIFAR-10 and ImageNet validate the above three predictions. In each experimental setting, it can be seen that larger initial dataset size and more aggressive pruning perform better than power-law scaling. Additionally, a larger initial data set may see better scaling (Figure 3A).
In addition, researchers have found that data pruning can improve the performance of transfer learning. They first analyzed ViT pretrained on ImageNet21K and then fine-tuned on different pruned subsets of CIFAR-10. Interestingly, the pretrained model allowed for more aggressive data pruning; only 10% of the CIFAR-10 fine-tuning matched or exceeded the performance achieved by all CIFAR-10 fine-tuning (Figure 4A). Additionally, Figure 4A provides a sample of power-law scaling breaking in a fine-tuned setting.
By pre-training ResNet50 on different pruned subsets of ImageNet1K (shown in Figure 3D), the researchers examined the efficacy of pruning the pre-trained data, and then used CIFAR Fine-tune them on -10. As shown in Figure 4B, pretraining on a minimum of 50% of ImageNet is able to match or exceed the CIFAR-10 performance achieved by pretraining on all ImageNet.
Therefore, pruning the pre-training data of upstream tasks can still maintain high performance on different downstream tasks. Overall, these results show the promise of pruning in transfer learning during the pre-training and fine-tuning stages.
Benchmarking supervised pruning metrics on ImageNet
The researchers noticed that most of the data pruning experiments were on small-scale data sets (i.e., variants of MNIST and CIFAR ) on. Therefore, the few pruning metrics proposed for ImageNet are rarely compared with baselines designed on smaller datasets.
Therefore, it is unclear how most pruning methods scale to ImageNet and which method is best. To investigate the theoretical impact of pruning metric quality on performance, we decided to fill this knowledge gap by conducting a systematic evaluation of 8 different supervised pruning metrics on ImageNet.
They observed significant performance differences between the metrics: Figure 5BC shows the test performance when a portion of the hardest samples under each metric was retained in the training set. Many metrics achieve success on smaller datasets, but when choosing a significantly smaller training subset (such as 80% of Imagenet), only a few still achieve comparable performance when trained on the full dataset.
Nonetheless, most measures still outperform random pruning (Figure 5C). Researchers found that all pruning metrics amplify class imbalance, resulting in performance degradation. To address this issue, the authors used a simple 50% class balance rate in all ImageNet experiments.
Self-supervised data pruning via prototype metrics
As shown in Figure 5, many data pruning metrics do not scale well to ImageNet, and some of them are indeed computationally intensive. Furthermore, all these metrics require annotations, which limits their data pruning capabilities for training large-scale base models on massive unlabeled datasets. Therefore, we clearly need simple, scalable, self-supervised pruning metrics.
To evaluate whether the clusters discovered by the metric are consistent with ImageNet classes, we compared their overlap in Figure 6A. The performance of self-supervised and supervised measures is similar when retaining more than 70% of the data, which shows the promise of self-supervised pruning.
For more research details, please refer to the original paper.
The above is the detailed content of Stanford, Meta AI Research: On the road to AGI, data pruning is more important than we think. For more information, please follow other related articles on the PHP Chinese website!

Hot AI Tools

Undresser.AI Undress
AI-powered app for creating realistic nude photos

AI Clothes Remover
Online AI tool for removing clothes from photos.

Undress AI Tool
Undress images for free

Clothoff.io
AI clothes remover

Video Face Swap
Swap faces in any video effortlessly with our completely free AI face swap tool!

Hot Article

Hot Tools

Notepad++7.3.1
Easy-to-use and free code editor

SublimeText3 Chinese version
Chinese version, very easy to use

Zend Studio 13.0.1
Powerful PHP integrated development environment

Dreamweaver CS6
Visual web development tools

SublimeText3 Mac version
God-level code editing software (SublimeText3)

Hot Topics
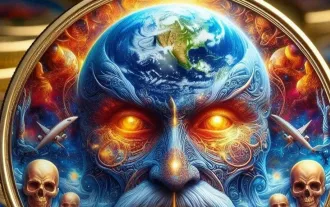
WorldCoin (WLD) stands out in the cryptocurrency market with its unique biometric verification and privacy protection mechanisms, attracting the attention of many investors. WLD has performed outstandingly among altcoins with its innovative technologies, especially in combination with OpenAI artificial intelligence technology. But how will the digital assets behave in the next few years? Let's predict the future price of WLD together. The 2025 WLD price forecast is expected to achieve significant growth in WLD in 2025. Market analysis shows that the average WLD price may reach $1.31, with a maximum of $1.36. However, in a bear market, the price may fall to around $0.55. This growth expectation is mainly due to WorldCoin2.
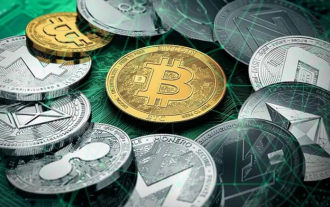
Factors of rising virtual currency prices include: 1. Increased market demand, 2. Decreased supply, 3. Stimulated positive news, 4. Optimistic market sentiment, 5. Macroeconomic environment; Decline factors include: 1. Decreased market demand, 2. Increased supply, 3. Strike of negative news, 4. Pessimistic market sentiment, 5. Macroeconomic environment.
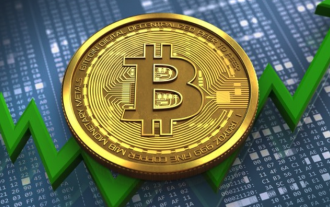
Exchanges that support cross-chain transactions: 1. Binance, 2. Uniswap, 3. SushiSwap, 4. Curve Finance, 5. Thorchain, 6. 1inch Exchange, 7. DLN Trade, these platforms support multi-chain asset transactions through various technologies.

Aavenomics is a proposal to modify the AAVE protocol token and introduce token repos, which has implemented a quorum for AAVEDAO. Marc Zeller, founder of the AAVE Project Chain (ACI), announced this on X, noting that it marks a new era for the agreement. Marc Zeller, founder of the AAVE Chain Initiative (ACI), announced on X that the Aavenomics proposal includes modifying the AAVE protocol token and introducing token repos, has achieved a quorum for AAVEDAO. According to Zeller, this marks a new era for the agreement. AaveDao members voted overwhelmingly to support the proposal, which was 100 per week on Wednesday
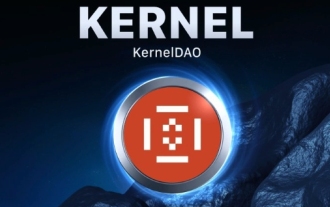
In the bustling world of cryptocurrencies, new opportunities always emerge. At present, KernelDAO (KERNEL) airdrop activity is attracting much attention and attracting the attention of many investors. So, what is the origin of this project? What benefits can BNB Holder get from it? Don't worry, the following will reveal it one by one for you.
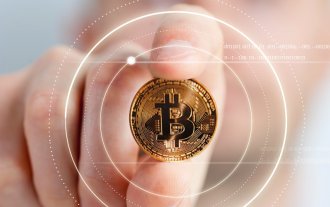
The steps to draw a Bitcoin structure analysis chart include: 1. Determine the purpose and audience of the drawing, 2. Select the right tool, 3. Design the framework and fill in the core components, 4. Refer to the existing template. Complete steps ensure that the chart is accurate and easy to understand.
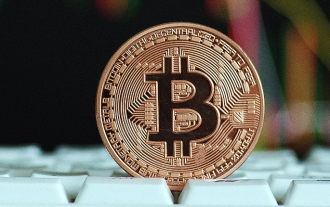
Suggestions for choosing a cryptocurrency exchange: 1. For liquidity requirements, priority is Binance, Gate.io or OKX, because of its order depth and strong volatility resistance. 2. Compliance and security, Coinbase, Kraken and Gemini have strict regulatory endorsement. 3. Innovative functions, KuCoin's soft staking and Bybit's derivative design are suitable for advanced users.
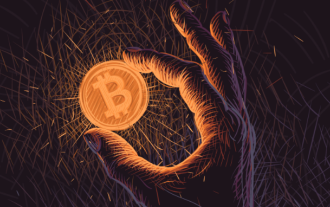
The platforms that have outstanding performance in leveraged trading, security and user experience in 2025 are: 1. OKX, suitable for high-frequency traders, providing up to 100 times leverage; 2. Binance, suitable for multi-currency traders around the world, providing 125 times high leverage; 3. Gate.io, suitable for professional derivatives players, providing 100 times leverage; 4. Bitget, suitable for novices and social traders, providing up to 100 times leverage; 5. Kraken, suitable for steady investors, providing 5 times leverage; 6. Bybit, suitable for altcoin explorers, providing 20 times leverage; 7. KuCoin, suitable for low-cost traders, providing 10 times leverage; 8. Bitfinex, suitable for senior play
