


Everyone has a ChatGPT! Microsoft DeepSpeed Chat is shockingly released, one-click RLHF training for hundreds of billions of large models
The dream of having a ChatGPT for everyone is about to come true?
Just now, Microsoft has open sourced a system framework that can add a complete RLHF process to model training - DeepSpeed Chat.
In other words, high-quality ChatGPT-like models of all sizes are now within easy reach!
## Project address: https://github.com/microsoft/DeepSpeed
Unlock 100 billion-level ChatGPT with one click and easily save 15 timesAs we all know, because OpenAI is not Open, the open source community has successively launched LLaMa and Alpaca in order to allow more people to use ChatGPT-like models. , Vicuna, Databricks-Dolly and other models.
However, due to the lack of an end-to-end RLHF scale system, the current training of ChatGPT-like models is still very difficult. The emergence of DeepSpeed Chat just makes up for this "bug".
What’s even brighter is that DeepSpeed Chat has greatly reduced costs.
Previously, expensive multi-GPU setups were beyond the capabilities of many researchers, and even with access to multi-GPU clusters, existing methods cannot afford hundreds of billions of parameter ChatGPT models training.
Now, for only $1,620, you can train an OPT-66B model in 2.1 days through the hybrid engine DeepSpeed-HE.
If you use a multi-node, multi-GPU system, DeepSpeed-HE can spend 320 US dollars to train an OPT-13B model in 1.25 hours, and spend 5120 US dollars to train an OPT-13B model in less than 1.25 hours. Train an OPT-175B model in one day.
Former Meta AI expert Elvis forwarded it excitedly, saying this was a big deal and expressed curiosity about how DeepSpeed Chat compared with ColossalChat.
Next, let’s take a look at the effect.
After training by DeepSpeed-Chat, the 1.3 billion parameter version "ChatGPT" performed very well in the question and answer session. Not only can you get the context of the question, but the answers given are also relevant.
In multiple rounds of conversations, the performance demonstrated by this 1.3 billion parameter version of "ChatGPT" completely exceeded the inherent impression of this scale. .
Of course, before you experience it, you need to configure the environment:
git clone https://github.com/microsoft/DeepSpeed.git cd DeepSpeed pip install . git clone https://github.com/microsoft/DeepSpeedExamples.git cd DeepSpeedExamples/applications/DeepSpeed-Chat/ pip install -r requirements.txt
A cup of coffee, after training the 1.3 billion parameter version of ChatGPT
If you only have about 1-2 hours of coffee or lunch break, You can also try to use DeepSpeed-Chat to train a "little toy".
The team has specially prepared a training example for the 1.3B model, which can be tested on consumer-grade GPUs. The best part is, when you come back from your lunch break, everything is ready.
python train.py --actor-model facebook/opt-1.3b --reward-model facebook/opt-350m --num-gpus 1
Consumer-grade NVIDIA A6000 GPU with 48GB of video memory:
一个GPU Node,半天搞定130亿参数
如果你只有半天的时间,以及一台服务器节点,则可以通过预训练的OPT-13B作为actor模型,OPT-350M作为reward模型,来生成一个130亿参数的类ChatGPT模型:
python train.py --actor-model facebook/opt-13b --reward-model facebook/opt-350m --num-gpus 8
单DGX节点,搭载了8个NVIDIA A100-40G GPU:
超省钱云方案,训练660亿参数模型
如果你可以使用多节点集群或云资源,并希望训练一个更大、更高质量的模型。那么只需基于下面这行代码,输入你想要的模型大小(如66B)和GPU数量(如64):
python train.py --actor-model facebook/opt-66b --reward-model facebook/opt-350m --num-gpus 64
8个DGX节点,每个节点配备8个NVIDIA A100-80G GPU:
具体来说,针对不同规模的模型和硬件配置,DeepSpeed-RLHF系统所需的时间和成本如下:
DeepSpeed Chat是个啥?
DeepSpeed Chat是一种通用系统框架,能够实现类似ChatGPT模型的端到端RLHF训练,从而帮助我们生成自己的高质量类ChatGPT模型。
DeepSpeed Chat具有以下三大核心功能:
1. 简化ChatGPT类型模型的训练和强化推理体验
开发者只需一个脚本,就能实现多个训练步骤,并且在完成后还可以利用推理API进行对话式交互测试。
2. DeepSpeed-RLHF模块
DeepSpeed-RLHF复刻了InstructGPT论文中的训练模式,并提供了数据抽象和混合功能,支持开发者使用多个不同来源的数据源进行训练。
3. DeepSpeed-RLHF系统
团队将DeepSpeed的训练(training engine)和推理能力(inference engine) 整合成了一个统一的混合引擎(DeepSpeed Hybrid Engine or DeepSpeed-HE)中,用于RLHF训练。由于,DeepSpeed-HE能够无缝地在推理和训练模式之间切换,因此可以利用来自DeepSpeed-Inference的各种优化。
DeepSpeed-RLHF系统在大规模训练中具有无与伦比的效率,使复杂的RLHF训练变得快速、经济并且易于大规模推广:
- 高效且经济:
DeepSpeed-HE比现有系统快15倍以上,使RLHF训练快速且经济实惠。
例如,DeepSpeed-HE在Azure云上只需9小时即可训练一个OPT-13B模型,只需18小时即可训练一个OPT-30B模型。这两种训练分别花费不到300美元和600美元。
- Excellent scalability:
DeepSpeed-HE can support training models with hundreds of billions of parameters and run on multi-node multi-GPU systems Demonstrates excellent scalability.
Therefore, even a model with 13 billion parameters can be trained in only 1.25 hours. For a model with 175 billion parameters, training using DeepSpeed-HE only takes less than a day.
- Popularizing RLHF training:
Only a single GPU, DeepSpeed-HE can support training for more than A model with 13 billion parameters. This enables data scientists and researchers who do not have access to multi-GPU systems to easily create not only lightweight RLHF models, but also large and powerful models to cope with different usage scenarios.
Complete RLHF training process
In order to provide a seamless training experience, the researcher followed InstructGPT and used it in DeepSpeed-Chat It contains a complete end-to-end training process.
##DeepSpeed-Chat’s RLHF training process diagram, including some optional functions
The process consists of three main steps:
- Step 1:
Supervised fine-tuning (SFT), using Featured human answers to fine-tune pre-trained language models to respond to various queries.
- Step 2:
Reward model fine-tuning, trained with a dataset containing human ratings of multiple answers to the same query An independent (usually smaller than SFT) reward model (RW).
- Step 3:
RLHF training. In this step, the SFT model is trained from the RW model by using the approximate policy optimization (PPO) algorithm. The reward feedback is further fine-tuned.
In step 3, the researcher also provides two additional functions to help improve the quality of the model:
-Exponential Moving Average (EMA) collection, you can select an EMA-based checkpoint for final evaluation.
- Hybrid training, mixing the pre-training objective (i.e. next word prediction) with the PPO objective to prevent performance regression on public benchmarks (such as SQuAD2.0).
EMA and hybrid training are two training features that are often ignored by other open source frameworks because they do not hinder training.
However, according to InstructGPT, EMA checkpoints tend to provide better response quality than traditional final training models, and hybrid training can help the model maintain pre-training baseline solving capabilities.
Therefore, the researchers provide these functions for users so that they can fully obtain the training experience described in InstructGPT.
In addition to being highly consistent with the InstructGPT paper, the researcher also provides functions that allow developers to use a variety of data resources to train their own RLHF models:
- Data abstraction and hybrid capabilities:
DeepSpeed-Chat is equipped with (1) an abstract dataset layer to unify the format of different datasets; and (2) data splitting Split/Blend functionality, whereby multiple datasets are blended appropriately and then split in 3 training stages.
DeepSpeed Hybrid Engine
Indicates steps 1 and 2 of the guided RLHF pipeline, similar to conventional fine-tuning of large models, which are driven by ZeRO-based optimization and flexible parallelism in DeepSpeed training A combination of strategies to achieve scale and speed.
And step 3 of the pipeline is the most complex part in terms of performance impact.
Each iteration needs to effectively handle two stages: a) the inference stage, used for token/experience generation, to generate training input; b) the training stage, updating actors and The weights of the reward model, as well as the interaction and scheduling between them.
It introduces two major difficulties: (1) memory cost, since multiple SFT and RW models need to be run throughout the third stage; (2) speed of the answer generation stage Slower, if not accelerated correctly, will significantly slow down the entire third stage.
In addition, the two important functions added by the researchers in the third stage-exponential moving average (EMA) collection and hybrid training will incur additional memory and training costs.
#To address these challenges, researchers have combined all system capabilities of DeepSpeed training and inference into a unified infrastructure , that is, Hybrid Engine.
It utilizes the original DeepSpeed engine for fast training mode, while effortlessly applying the DeepSpeed inference engine for generation/evaluation mode, providing a better solution for the third stage of RLHF training. Fast training system.
As shown in the figure below, the transition between the DeepSpeed training and inference engines is seamless: by enabling the typical eval and train modes for the actor model, when running the inference and training processes , DeepSpeed has chosen different optimizations to run the model faster and improve the throughput of the overall system.
##DeepSpeed hybrid engine design to accelerate the most time-consuming part of the RLHF process
During the inference execution process of the experience generation phase of RLHF training, the DeepSpeed hybrid engine uses a lightweight memory management system to handle KV cache and intermediate results, while using highly optimized inference CUDA cores and tensor parallel computing , compared with existing solutions, the throughput (number of tokens per second) has been greatly improved.
During training, the hybrid engine enables memory optimization technologies such as DeepSpeed’s ZeRO family of technologies and Low-Order Adaptation (LoRA).
The way researchers design and implement optimization of these systems is to make them compatible with each other and can be combined together to provide the highest training efficiency under a unified hybrid engine.
The hybrid engine can seamlessly change model partitioning during training and inference to support tensor parallel-based inference and ZeRO-based training sharding mechanisms.
It can also reconfigure the memory system to maximize memory availability in every mode.
This avoids the memory allocation bottleneck and can support large batch sizes, greatly improving performance.
In summary, the Hybrid Engine pushes the boundaries of modern RLHF training, delivering unparalleled scale and system efficiency for RLHF workloads.
Compared with existing systems such as Colossal-AI or HuggingFace-DDP, DeepSpeed-Chat has over an order of magnitude throughput, enabling training larger actor models at the same latency budget or training similarly sized models at lower cost.
For example, DeepSpeed improves the throughput of RLHF training by more than 10 times on a single GPU. While both CAI-Coati and HF-DDP can run 1.3B models, DeepSpeed can run 6.5B models on the same hardware, which is directly 5 times higher.
On multiple GPUs on a single node, DeepSpeed-Chat is 6-19 times faster than CAI-Coati in terms of system throughput, HF- DDP is accelerated by 1.4-10.5 times.
The team stated that one of the key reasons why DeepSpeed-Chat can achieve such excellent results is the acceleration provided by the hybrid engine during the generation phase.
The above is the detailed content of Everyone has a ChatGPT! Microsoft DeepSpeed Chat is shockingly released, one-click RLHF training for hundreds of billions of large models. For more information, please follow other related articles on the PHP Chinese website!

Hot AI Tools

Undresser.AI Undress
AI-powered app for creating realistic nude photos

AI Clothes Remover
Online AI tool for removing clothes from photos.

Undress AI Tool
Undress images for free

Clothoff.io
AI clothes remover

Video Face Swap
Swap faces in any video effortlessly with our completely free AI face swap tool!

Hot Article

Hot Tools

Notepad++7.3.1
Easy-to-use and free code editor

SublimeText3 Chinese version
Chinese version, very easy to use

Zend Studio 13.0.1
Powerful PHP integrated development environment

Dreamweaver CS6
Visual web development tools

SublimeText3 Mac version
God-level code editing software (SublimeText3)

Hot Topics
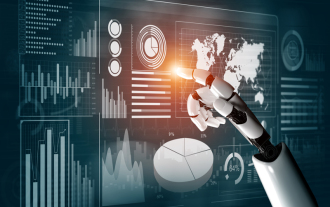
Text annotation is the work of corresponding labels or tags to specific content in text. Its main purpose is to provide additional information to the text for deeper analysis and processing, especially in the field of artificial intelligence. Text annotation is crucial for supervised machine learning tasks in artificial intelligence applications. It is used to train AI models to help more accurately understand natural language text information and improve the performance of tasks such as text classification, sentiment analysis, and language translation. Through text annotation, we can teach AI models to recognize entities in text, understand context, and make accurate predictions when new similar data appears. This article mainly recommends some better open source text annotation tools. 1.LabelStudiohttps://github.com/Hu
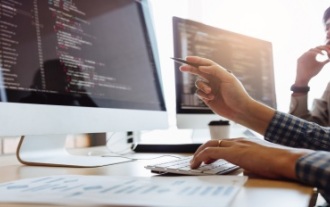
Image annotation is the process of associating labels or descriptive information with images to give deeper meaning and explanation to the image content. This process is critical to machine learning, which helps train vision models to more accurately identify individual elements in images. By adding annotations to images, the computer can understand the semantics and context behind the images, thereby improving the ability to understand and analyze the image content. Image annotation has a wide range of applications, covering many fields, such as computer vision, natural language processing, and graph vision models. It has a wide range of applications, such as assisting vehicles in identifying obstacles on the road, and helping in the detection and diagnosis of diseases through medical image recognition. . This article mainly recommends some better open source and free image annotation tools. 1.Makesens
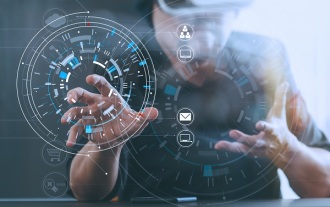
Face detection and recognition technology is already a relatively mature and widely used technology. Currently, the most widely used Internet application language is JS. Implementing face detection and recognition on the Web front-end has advantages and disadvantages compared to back-end face recognition. Advantages include reducing network interaction and real-time recognition, which greatly shortens user waiting time and improves user experience; disadvantages include: being limited by model size, the accuracy is also limited. How to use js to implement face detection on the web? In order to implement face recognition on the Web, you need to be familiar with related programming languages and technologies, such as JavaScript, HTML, CSS, WebRTC, etc. At the same time, you also need to master relevant computer vision and artificial intelligence technologies. It is worth noting that due to the design of the Web side
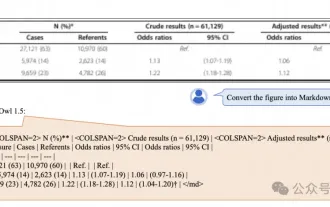
New SOTA for multimodal document understanding capabilities! Alibaba's mPLUG team released the latest open source work mPLUG-DocOwl1.5, which proposed a series of solutions to address the four major challenges of high-resolution image text recognition, general document structure understanding, instruction following, and introduction of external knowledge. Without further ado, let’s look at the effects first. One-click recognition and conversion of charts with complex structures into Markdown format: Charts of different styles are available: More detailed text recognition and positioning can also be easily handled: Detailed explanations of document understanding can also be given: You know, "Document Understanding" is currently An important scenario for the implementation of large language models. There are many products on the market to assist document reading. Some of them mainly use OCR systems for text recognition and cooperate with LLM for text processing.
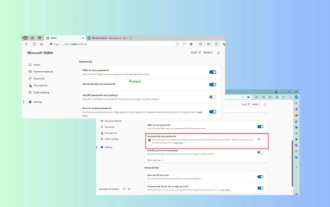
News on April 18th: Recently, some users of the Microsoft Edge browser using the Canary channel reported that after upgrading to the latest version, they found that the option to automatically save passwords was disabled. After investigation, it was found that this was a minor adjustment after the browser upgrade, rather than a cancellation of functionality. Before using the Edge browser to access a website, users reported that the browser would pop up a window asking if they wanted to save the login password for the website. After choosing to save, Edge will automatically fill in the saved account number and password the next time you log in, providing users with great convenience. But the latest update resembles a tweak, changing the default settings. Users need to choose to save the password and then manually turn on automatic filling of the saved account and password in the settings.
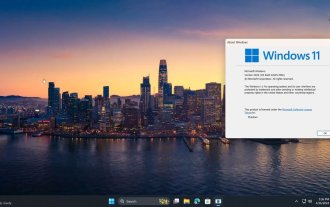
According to news from this site on April 27, Microsoft released the Windows 11 Build 26100 preview version update to the Canary and Dev channels earlier this month, which is expected to become a candidate RTM version of the Windows 1124H2 update. The main changes in the new version are the file explorer, Copilot integration, editing PNG file metadata, creating TAR and 7z compressed files, etc. @PhantomOfEarth discovered that Microsoft has devolved some functions of the 24H2 version (Germanium) to the 23H2/22H2 (Nickel) version, such as creating TAR and 7z compressed files. As shown in the diagram, Windows 11 will support native creation of TAR
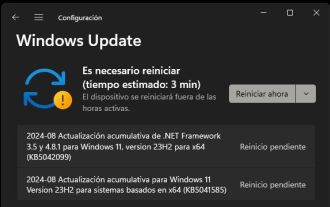
According to news from this site on August 14, during today’s August Patch Tuesday event day, Microsoft released cumulative updates for Windows 11 systems, including the KB5041585 update for 22H2 and 23H2, and the KB5041592 update for 21H2. After the above-mentioned equipment is installed with the August cumulative update, the version number changes attached to this site are as follows: After the installation of the 21H2 equipment, the version number increased to Build22000.314722H2. After the installation of the equipment, the version number increased to Build22621.403723H2. After the installation of the equipment, the version number increased to Build22631.4037. The main contents of the KB5041585 update for Windows 1121H2 are as follows: Improvement: Improved
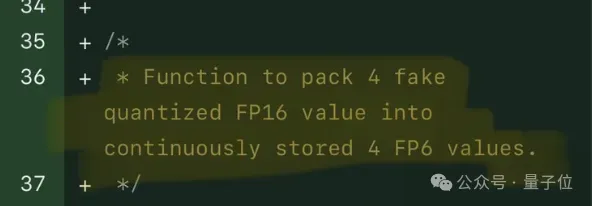
FP8 and lower floating point quantification precision are no longer the "patent" of H100! Lao Huang wanted everyone to use INT8/INT4, and the Microsoft DeepSpeed team started running FP6 on A100 without official support from NVIDIA. Test results show that the new method TC-FPx's FP6 quantization on A100 is close to or occasionally faster than INT4, and has higher accuracy than the latter. On top of this, there is also end-to-end large model support, which has been open sourced and integrated into deep learning inference frameworks such as DeepSpeed. This result also has an immediate effect on accelerating large models - under this framework, using a single card to run Llama, the throughput is 2.65 times higher than that of dual cards. one
