


Learning ChatGPT, what will happen if human feedback is introduced into AI painting?
Recently, deep generative models have achieved remarkable success in generating high-quality images from text prompts, in part due to the scaling of deep generative models to large-scale web datasets such as LAION. However, some significant challenges remain, preventing large-scale text-to-image models from generating images that are perfectly aligned with text prompts. For example, current text-to-image models often fail to generate reliable visual text and have difficulty with combined image generation.
Back in the field of language modeling, learning from human feedback has become a powerful solution for “aligning model behavior with human intent.” This type of method first learns a reward function designed to reflect what humans care about in the task through human feedback on the model output, and then uses the learned reward function through a reinforcement learning algorithm (such as proximal policy optimization PPO) to Optimize language models. This reinforcement learning with human feedback framework (RLHF) has successfully combined large-scale language models (such as GPT-3) with sophisticated human quality assessment.
Recently, inspired by the success of RLHF in the language field, researchers from Google Research and Berkeley, California, proposed a fine-tuning method that uses human feedback to align text to image models.
##Paper address: https://arxiv.org/pdf/2302.12192v1.pdf
The method in this article is shown in Figure 1 below, which is mainly divided into 3 steps.
Step one: First generate different images from a set of text prompts "designed to test the alignment of text to image model output". Specifically, examine the pretrained model's more error-prone prompts—generating objects with a specific color, number, and background, and then collecting binary human feedback used to evaluate the model's output.
Step 2: Using a human-labeled dataset, train a reward function to predict human feedback given image and text prompts. We propose an auxiliary task to identify original text prompts among a set of perturbed text prompts to more effectively use human feedback for reward learning. This technique improves the generalization of the reward function to unseen images and text prompts.
Step 3: Update the text-to-image model via reward-weighted likelihood maximization to better align it with human feedback. Unlike previous work that used reinforcement learning for optimization, the researchers used semi-supervised learning to update the model to measure the quality of the model output, which is the learned reward function.
Researchers used 27,000 image-text pairs with human feedback to fine-tune the Stable Diffusion model, and the results show fine-tuning The latter model achieves significant improvements in generating objects with specific colors, quantities, and backgrounds. Achieved up to 47% improvement in image-text alignment at a slight loss in image fidelity.
Additionally, combined generation results have been improved to better generate unseen objects given a combination of unseen color, quantity, and background prompts. They also observed that the learned reward function matched human assessments of alignment better than CLIP scores on test text prompts.
However, Kimin Lee, the first author of the paper, also said that the results of this paper did not solve all the failure models in the existing text-to-image model, and there are still many challenges. They hope this work will highlight the potential of learning from human feedback in aligning Vincent graph models.
Method introduction
In order to align the generated image with the text prompt, this study performed a series of fine-tuning on the pre-trained model, and the process is shown in Figure 1 above. First, corresponding images were generated from a set of text prompts, a process designed to test various performances of the Vincentian graph model; then human raters provided binary feedback on these generated images; next, the study trained a reward model to predict human feedback with text prompts and images as input; finally, the study uses reward-weighted log-likelihood to fine-tune the Vincent graph model to improve text-image alignment.
Human Data Collection
To test the functionality of the Vincent graph model, the study considered three categories of text prompts: Specified count, color, background. For each category, the study generated prompts by pairing each word or phrase that described the object, such as green (color) with a dog (quantity). Additionally, the study considered combinations of three categories (e.g., two dogs dyed green in a city). Table 1 below better illustrates the dataset classification. Each prompt will be used to generate 60 images, and the model is mainly Stable Diffusion v1.5.
Human Feedback
Next comments Generated images for human feedback. Three images generated by the same prompt are presented to the labelers, and they are asked to evaluate whether each generated image is consistent with the prompt, and the evaluation criteria are good or bad. Since this task is relatively simple, binary feedback will suffice.
Reward Learning
To better evaluate image-text alignment, this study uses a reward function To measure, this function can map the CLIP embedding of image x and text prompt z to scalar values. It is then used to predict human feedback k_y ∈ {0, 1} (1 = good, 0 = bad).
Formally speaking, given the human feedback data set D^human = {(x, z, y)}, the reward functionTrain by minimizing the mean square error (MSE):
Previously, it has been Studies have shown that data augmentation methods can significantly improve data efficiency and model learning performance. In order to effectively utilize the feedback data set, this study designed a simple data augmentation scheme and an auxiliary loss that rewards learning. This study uses augmented prompts in an auxiliary task, that is, classification reward learning is performed on the original prompts. The Prompt classifier uses a reward function as follows:
##The auxiliary loss is:
#The last step is to update the Vincent diagram model. Since the diversity of the data set generated by the model is limited, it may lead to overfitting. To mitigate this, the study also minimized the pre-training loss as follows:
Experimental results
The experimental part is designed to test the effectiveness of human feedback participating in model fine-tuning. The model used in the experiment is Stable Diffusion v1.5; the data set information is shown in Table 1 (see above) and Table 2. Table 2 shows the distribution of feedback provided by multiple human labelers.
Human ratings of text-image alignment (evaluation metrics are color, number of objects). As shown in Figure 4, our method significantly improved image-text alignment. Specifically, 50% of the samples generated by the model received at least two-thirds of the votes in favor (the number of votes was 7 or more votes in favor). votes), however, fine-tuning slightly reduces image fidelity (15% vs. 10%).
Figure 2 shows examples of images from the original model and our fine-tuned counterpart. It can be seen that the original model generated images that lacked details (such as color, background, or count) (Figure 2 (a)), and the image generated by our model conforms to the color, count, and background specified by prompt. It is worth noting that our model can also generate unseen text prompt images with very high quality (Figure 2 (b)).
Reward the results of learning. Figure 3(a) shows the model’s scores in seen text prompts and unseen text prompts. Having rewards (green) is more consistent with typical human intentions than CLIP scores (red).
The above is the detailed content of Learning ChatGPT, what will happen if human feedback is introduced into AI painting?. For more information, please follow other related articles on the PHP Chinese website!

Hot AI Tools

Undresser.AI Undress
AI-powered app for creating realistic nude photos

AI Clothes Remover
Online AI tool for removing clothes from photos.

Undress AI Tool
Undress images for free

Clothoff.io
AI clothes remover

Video Face Swap
Swap faces in any video effortlessly with our completely free AI face swap tool!

Hot Article

Hot Tools

Notepad++7.3.1
Easy-to-use and free code editor

SublimeText3 Chinese version
Chinese version, very easy to use

Zend Studio 13.0.1
Powerful PHP integrated development environment

Dreamweaver CS6
Visual web development tools

SublimeText3 Mac version
God-level code editing software (SublimeText3)

Hot Topics
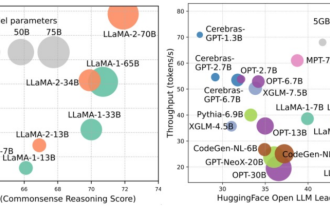
Large-scale language models (LLMs) have demonstrated compelling capabilities in many important tasks, including natural language understanding, language generation, and complex reasoning, and have had a profound impact on society. However, these outstanding capabilities require significant training resources (shown in the left image) and long inference times (shown in the right image). Therefore, researchers need to develop effective technical means to solve their efficiency problems. In addition, as can be seen from the right side of the figure, some efficient LLMs (LanguageModels) such as Mistral-7B have been successfully used in the design and deployment of LLMs. These efficient LLMs can significantly reduce inference memory while maintaining similar accuracy to LLaMA1-33B
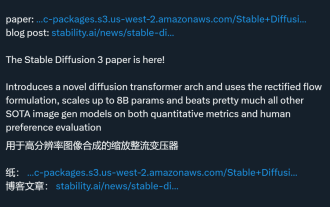
StableDiffusion3’s paper is finally here! This model was released two weeks ago and uses the same DiT (DiffusionTransformer) architecture as Sora. It caused quite a stir once it was released. Compared with the previous version, the quality of the images generated by StableDiffusion3 has been significantly improved. It now supports multi-theme prompts, and the text writing effect has also been improved, and garbled characters no longer appear. StabilityAI pointed out that StableDiffusion3 is a series of models with parameter sizes ranging from 800M to 8B. This parameter range means that the model can be run directly on many portable devices, significantly reducing the use of AI
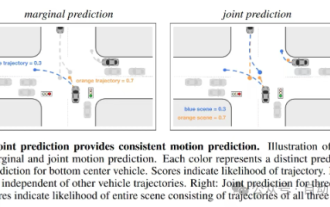
Trajectory prediction plays an important role in autonomous driving. Autonomous driving trajectory prediction refers to predicting the future driving trajectory of the vehicle by analyzing various data during the vehicle's driving process. As the core module of autonomous driving, the quality of trajectory prediction is crucial to downstream planning control. The trajectory prediction task has a rich technology stack and requires familiarity with autonomous driving dynamic/static perception, high-precision maps, lane lines, neural network architecture (CNN&GNN&Transformer) skills, etc. It is very difficult to get started! Many fans hope to get started with trajectory prediction as soon as possible and avoid pitfalls. Today I will take stock of some common problems and introductory learning methods for trajectory prediction! Introductory related knowledge 1. Are the preview papers in order? A: Look at the survey first, p
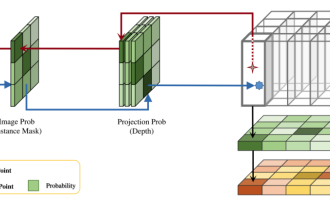
This paper explores the problem of accurately detecting objects from different viewing angles (such as perspective and bird's-eye view) in autonomous driving, especially how to effectively transform features from perspective (PV) to bird's-eye view (BEV) space. Transformation is implemented via the Visual Transformation (VT) module. Existing methods are broadly divided into two strategies: 2D to 3D and 3D to 2D conversion. 2D-to-3D methods improve dense 2D features by predicting depth probabilities, but the inherent uncertainty of depth predictions, especially in distant regions, may introduce inaccuracies. While 3D to 2D methods usually use 3D queries to sample 2D features and learn the attention weights of the correspondence between 3D and 2D features through a Transformer, which increases the computational and deployment time.
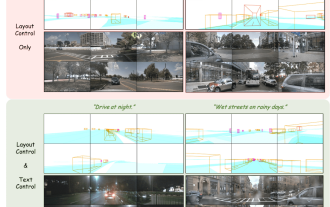
Some of the author’s personal thoughts In the field of autonomous driving, with the development of BEV-based sub-tasks/end-to-end solutions, high-quality multi-view training data and corresponding simulation scene construction have become increasingly important. In response to the pain points of current tasks, "high quality" can be decoupled into three aspects: long-tail scenarios in different dimensions: such as close-range vehicles in obstacle data and precise heading angles during car cutting, as well as lane line data. Scenes such as curves with different curvatures or ramps/mergings/mergings that are difficult to capture. These often rely on large amounts of data collection and complex data mining strategies, which are costly. 3D true value - highly consistent image: Current BEV data acquisition is often affected by errors in sensor installation/calibration, high-precision maps and the reconstruction algorithm itself. this led me to
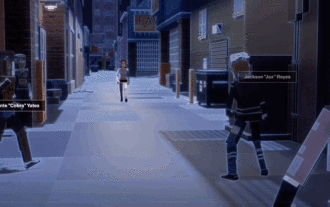
Please note that this square man is frowning, thinking about the identities of the "uninvited guests" in front of him. It turned out that she was in a dangerous situation, and once she realized this, she quickly began a mental search to find a strategy to solve the problem. Ultimately, she decided to flee the scene and then seek help as quickly as possible and take immediate action. At the same time, the person on the opposite side was thinking the same thing as her... There was such a scene in "Minecraft" where all the characters were controlled by artificial intelligence. Each of them has a unique identity setting. For example, the girl mentioned before is a 17-year-old but smart and brave courier. They have the ability to remember and think, and live like humans in this small town set in Minecraft. What drives them is a brand new,
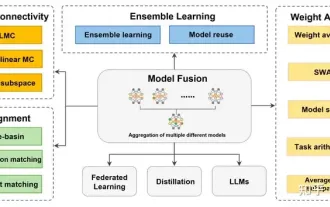
In September 23, the paper "DeepModelFusion:ASurvey" was published by the National University of Defense Technology, JD.com and Beijing Institute of Technology. Deep model fusion/merging is an emerging technology that combines the parameters or predictions of multiple deep learning models into a single model. It combines the capabilities of different models to compensate for the biases and errors of individual models for better performance. Deep model fusion on large-scale deep learning models (such as LLM and basic models) faces some challenges, including high computational cost, high-dimensional parameter space, interference between different heterogeneous models, etc. This article divides existing deep model fusion methods into four categories: (1) "Pattern connection", which connects solutions in the weight space through a loss-reducing path to obtain a better initial model fusion
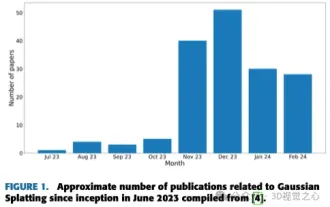
Written above & The author’s personal understanding is that image-based 3D reconstruction is a challenging task that involves inferring the 3D shape of an object or scene from a set of input images. Learning-based methods have attracted attention for their ability to directly estimate 3D shapes. This review paper focuses on state-of-the-art 3D reconstruction techniques, including generating novel, unseen views. An overview of recent developments in Gaussian splash methods is provided, including input types, model structures, output representations, and training strategies. Unresolved challenges and future directions are also discussed. Given the rapid progress in this field and the numerous opportunities to enhance 3D reconstruction methods, a thorough examination of the algorithm seems crucial. Therefore, this study provides a comprehensive overview of recent advances in Gaussian scattering. (Swipe your thumb up
