


An article briefly analyzing multi-sensor fusion for autonomous driving
What does intelligent connected cars have to do with autonomous driving?
The core of autonomous driving lies in the car, so what is the intelligent network connection system? The carrier of intelligent network connection is also the car, but the core is the network that needs to be connected. One is a network composed of sensors and intelligent control systems inside the car, and the other is a network connected and shared by all cars. Network connection is to put a car into a large network to exchange important information, such as location, route, speed and other information. The development goal of the intelligent network system is to improve the safety and comfort of the car through the design optimization of the car's internal sensors and control systems, making the car more humane. Of course, the ultimate goal is to achieve driverless driving.
The three core auxiliary systems of autonomous vehicles: environment perception system, decision-making and planning system, and control and execution system. These are also the three key technologies that the intelligent networked vehicle itself must solve. question.
What role does the environment awareness system play in the intelligent network connection system?
What is environment awareness technology and what does it mainly include?
Environment perception mainly includes three aspects: sensors, perception and positioning. Sensors include cameras, millimeter-wave radar, lidar, and ultrasonic waves. Different sensors are placed on the vehicle to collect data, identify colors, and measure distances.
If a smart car wants to use the data obtained by the sensor to achieve intelligent driving, the data obtained through the sensor must be processed by a (perception) algorithm and calculated Data results are generated to realize the exchange of information about vehicles, roads, people, etc., so that vehicles can automatically analyze whether the vehicle is driving safely or dangerously, so that vehicles can achieve intelligent driving according to people's wishes, and ultimately replace people in making decisions and autonomous driving goals. .
Then there will be a key technical issue here. Different sensors play different roles. How can the data scanned by multiple sensors form a complete object image data?
Multi-sensor fusion technology
The main function of the camera is to identify the color of objects, but it will be affected by rainy weather ; Millimeter wave radar can make up for the disadvantages of cameras being affected by rainy days, and can identify relatively distant obstacles, such as pedestrians, roadblocks, etc., but cannot identify the specific shape of obstacles; lidar can make up for the inability of millimeter wave radar to identify obstacles. Disadvantages of the specific shape; Ultrasonic radar mainly identifies short-range obstacles on the vehicle body, and is often used in the vehicle parking process. In order to fuse the external data collected from different sensors to provide a basis for the controller to make decisions, it is necessary to process the multi-sensor fusion algorithm to form a panoramic perception.
What is multi-sensor fusion (fusion algorithm processing), and what are the main fusion algorithms?
The basic principle of multi-sensor fusion is just like the process of comprehensive information processing by the human brain. Various sensors are used to complement and optimize the combination of information at multiple levels and in multiple spaces, and finally produce a pair of observations. Consistent interpretation of the environment. In this process, multi-source data must be fully utilized for reasonable control and use, and the ultimate goal of information fusion is to derive more useful information through multi-level and multi-aspect combinations of information based on the separated observation information obtained by each sensor. This not only takes advantage of the cooperative operation of multiple sensors, but also comprehensively processes data from other information sources to improve the intelligence of the entire sensor system.
The concept of multi-sensor data fusion was first used in the military field. In recent years, with the development of autonomous driving, various radars have been used to target vehicles. detection. Because different sensors have data accuracy issues, how to determine the final fused data? For example, if the lidar reports that the distance to the vehicle in front is 5m, the millimeter-wave radar reports that the distance to the vehicle in front is 5.5m, and the camera determines that the distance to the vehicle in front is 4m, how should the central processor make the final judgment? Then a set of multi-data fusion algorithms are needed to solve this problem.
Commonly used methods of multi-sensor fusion are divided into two categories: random and artificial intelligence. The AI category mainly includes fuzzy logic reasoning and artificial neural network methods; the stochastic methods mainly include Bayesian filtering, Kalman filtering and other algorithms. At present, automotive fusion sensing mainly uses random fusion algorithms.
The fusion perception algorithm of autonomous vehicles mainly uses the Kalman filter algorithm, which uses linear system state equations to optimally estimate the system state through system input and output observation data. It is an algorithm that currently solves most problems. They are all the best and most efficient methods.
Multiple sensors need to be processed by fusion algorithms. Enterprises will need algorithm engineers in the fusion sensing category to solve the problem of multi-sensor fusion. Most of the job requirements in the fusion sensing category are It is necessary to be able to master the working principles of various sensors and the data characteristics of signals, to be able to master fusion algorithms for software development and sensor calibration algorithm capabilities, as well as point cloud data processing, deep learning detection algorithms, etc.
The third part of environment awareness - positioning (slam)
Slam is called simultaneous positioning and mapping, which assumes that the scene is static In this case, the image sequence is obtained through the movement of the camera and the design of the 3-D structure of the scene is obtained. This is an important task of computer vision. The data obtained by the camera is processed by an algorithm, which is visual slam.
In addition to visual slam, environment-aware positioning methods also include lidar slam, GPS/IMU and high-precision maps. The data obtained by these sensors need to be processed by algorithms to form data results that provide location information basis for autonomous driving decisions. Therefore, if you want to work in the field of environmental perception, you can not only choose the fusion sensing algorithm position, but also choose the slam field.
The above is the detailed content of An article briefly analyzing multi-sensor fusion for autonomous driving. For more information, please follow other related articles on the PHP Chinese website!

Hot AI Tools

Undresser.AI Undress
AI-powered app for creating realistic nude photos

AI Clothes Remover
Online AI tool for removing clothes from photos.

Undress AI Tool
Undress images for free

Clothoff.io
AI clothes remover

Video Face Swap
Swap faces in any video effortlessly with our completely free AI face swap tool!

Hot Article

Hot Tools

Notepad++7.3.1
Easy-to-use and free code editor

SublimeText3 Chinese version
Chinese version, very easy to use

Zend Studio 13.0.1
Powerful PHP integrated development environment

Dreamweaver CS6
Visual web development tools

SublimeText3 Mac version
God-level code editing software (SublimeText3)

Hot Topics










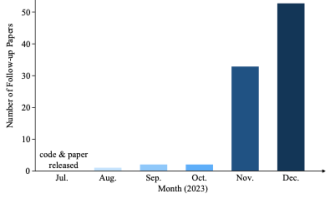
Written above & the author’s personal understanding Three-dimensional Gaussiansplatting (3DGS) is a transformative technology that has emerged in the fields of explicit radiation fields and computer graphics in recent years. This innovative method is characterized by the use of millions of 3D Gaussians, which is very different from the neural radiation field (NeRF) method, which mainly uses an implicit coordinate-based model to map spatial coordinates to pixel values. With its explicit scene representation and differentiable rendering algorithms, 3DGS not only guarantees real-time rendering capabilities, but also introduces an unprecedented level of control and scene editing. This positions 3DGS as a potential game-changer for next-generation 3D reconstruction and representation. To this end, we provide a systematic overview of the latest developments and concerns in the field of 3DGS for the first time.
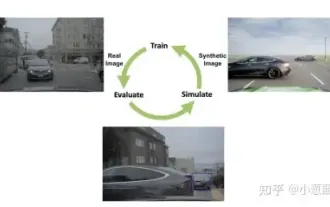
Yesterday during the interview, I was asked whether I had done any long-tail related questions, so I thought I would give a brief summary. The long-tail problem of autonomous driving refers to edge cases in autonomous vehicles, that is, possible scenarios with a low probability of occurrence. The perceived long-tail problem is one of the main reasons currently limiting the operational design domain of single-vehicle intelligent autonomous vehicles. The underlying architecture and most technical issues of autonomous driving have been solved, and the remaining 5% of long-tail problems have gradually become the key to restricting the development of autonomous driving. These problems include a variety of fragmented scenarios, extreme situations, and unpredictable human behavior. The "long tail" of edge scenarios in autonomous driving refers to edge cases in autonomous vehicles (AVs). Edge cases are possible scenarios with a low probability of occurrence. these rare events
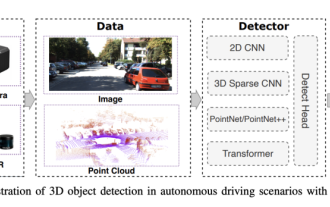
0.Written in front&& Personal understanding that autonomous driving systems rely on advanced perception, decision-making and control technologies, by using various sensors (such as cameras, lidar, radar, etc.) to perceive the surrounding environment, and using algorithms and models for real-time analysis and decision-making. This enables vehicles to recognize road signs, detect and track other vehicles, predict pedestrian behavior, etc., thereby safely operating and adapting to complex traffic environments. This technology is currently attracting widespread attention and is considered an important development area in the future of transportation. one. But what makes autonomous driving difficult is figuring out how to make the car understand what's going on around it. This requires that the three-dimensional object detection algorithm in the autonomous driving system can accurately perceive and describe objects in the surrounding environment, including their locations,
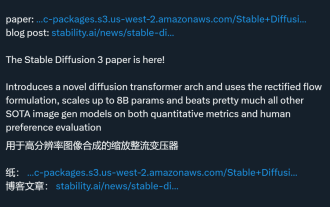
StableDiffusion3’s paper is finally here! This model was released two weeks ago and uses the same DiT (DiffusionTransformer) architecture as Sora. It caused quite a stir once it was released. Compared with the previous version, the quality of the images generated by StableDiffusion3 has been significantly improved. It now supports multi-theme prompts, and the text writing effect has also been improved, and garbled characters no longer appear. StabilityAI pointed out that StableDiffusion3 is a series of models with parameter sizes ranging from 800M to 8B. This parameter range means that the model can be run directly on many portable devices, significantly reducing the use of AI
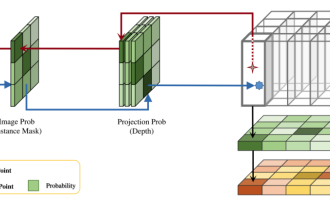
This paper explores the problem of accurately detecting objects from different viewing angles (such as perspective and bird's-eye view) in autonomous driving, especially how to effectively transform features from perspective (PV) to bird's-eye view (BEV) space. Transformation is implemented via the Visual Transformation (VT) module. Existing methods are broadly divided into two strategies: 2D to 3D and 3D to 2D conversion. 2D-to-3D methods improve dense 2D features by predicting depth probabilities, but the inherent uncertainty of depth predictions, especially in distant regions, may introduce inaccuracies. While 3D to 2D methods usually use 3D queries to sample 2D features and learn the attention weights of the correspondence between 3D and 2D features through a Transformer, which increases the computational and deployment time.

Original title: SIMPL: ASimpleandEfficientMulti-agentMotionPredictionBaselineforAutonomousDriving Paper link: https://arxiv.org/pdf/2402.02519.pdf Code link: https://github.com/HKUST-Aerial-Robotics/SIMPL Author unit: Hong Kong University of Science and Technology DJI Paper idea: This paper proposes a simple and efficient motion prediction baseline (SIMPL) for autonomous vehicles. Compared with traditional agent-cent
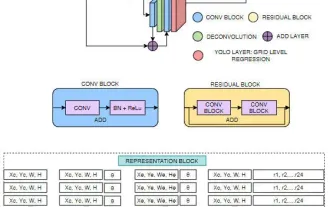
Target detection is a relatively mature problem in autonomous driving systems, among which pedestrian detection is one of the earliest algorithms to be deployed. Very comprehensive research has been carried out in most papers. However, distance perception using fisheye cameras for surround view is relatively less studied. Due to large radial distortion, standard bounding box representation is difficult to implement in fisheye cameras. To alleviate the above description, we explore extended bounding box, ellipse, and general polygon designs into polar/angular representations and define an instance segmentation mIOU metric to analyze these representations. The proposed model fisheyeDetNet with polygonal shape outperforms other models and simultaneously achieves 49.5% mAP on the Valeo fisheye camera dataset for autonomous driving
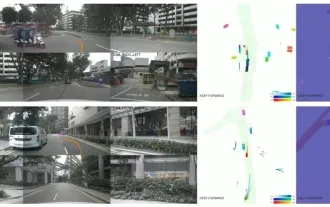
In the past month, due to some well-known reasons, I have had very intensive exchanges with various teachers and classmates in the industry. An inevitable topic in the exchange is naturally end-to-end and the popular Tesla FSDV12. I would like to take this opportunity to sort out some of my thoughts and opinions at this moment for your reference and discussion. How to define an end-to-end autonomous driving system, and what problems should be expected to be solved end-to-end? According to the most traditional definition, an end-to-end system refers to a system that inputs raw information from sensors and directly outputs variables of concern to the task. For example, in image recognition, CNN can be called end-to-end compared to the traditional feature extractor + classifier method. In autonomous driving tasks, input data from various sensors (camera/LiDAR
