Behind the large AI model, there are staggering carbon emissions
Since large-scale language models like ChatGPT became a global sensation, few people have noticed that training and running large-scale language models is generating staggering carbon emissions.
Although neither OpenAI nor Google have said how much their respective products’ computing costs are, according to third-party researchers According to the analysis, the ChatGPT part of the training consumed 1,287 megawatt hours and resulted in more than 550 tons of carbon dioxide emissions, which is equivalent to a person traveling back and forth between New York and San Francisco 550 times.
In fact, this is only the emission during training. More carbon dioxide will be emitted when the AI large model is running.
Martin Bouchard, co-founder of QScale, a Canadian data center company, believes that in order to meet the growing needs of search engine users, Microsoft and Google have added generative AI such as ChatGPT to searches, which will lead to Each search increases the computational effort by at least 4 to 5 times.
If you have to retrain the model frequently and add more parameters, the scale of calculation is completely different.
According to the International Energy Agency (International Energy Agency), greenhouse gas emissions from data centers already account for about 1% of global greenhouse gas emissions.
This number is expected to rise as large AI models and demand for cloud computing grow.
AI large models are becoming an important source of carbon emissions.
1. Reduce the carbon emissions of large AI models
The training and operation process of AI models consumes a lot of energy, but the key issue is how to know and measure a single machine How many greenhouse gas emissions are learning experiments generating, and how much can be reduced?
Currently, data scientists still cannot easily and reliably obtain measurement results in this field, which also hinders the further development of feasible response strategies.
In response to this problem, Google published a study detailing the energy costs of state-of-the-art language models, including early and larger versions of LaMDA.
Research results show that combining efficient models, processors and data centers with clean energy can reduce the carbon footprint of machine learning systems by up to 1,000 times.
The team proposed four basic methods to significantly reduce the carbon (and energy) footprint of machine learning workloads, which are currently used at Google and anyone using Google Cloud services Can be used by anyone.
Google energy and carbon footprint reduction best practices (4Ms) are as follows:
- Model: Choose efficient ones, researchers say ML model architecture is crucial as it has the potential to improve ML quality while cutting computation time in half.
- Machine: Using processors and systems specifically designed for ML training can increase performance and energy efficiency by 2x to 5x compared to general-purpose processors.
- Mechanization: In most cases, on-premises data centers are older and smaller. Therefore, the cost of new energy-efficient cooling and power distribution systems cannot be amortized.
The cloud-based data center is a new, custom-designed warehouse with energy-efficient features that can accommodate 50,000 servers. They provide exceptionally efficient power utilization (PUE).
Therefore, computing in the cloud instead of locally can save 1.4-2 times energy and reduce pollution.
- Optimization: The cloud allows customers to select areas with the cleanest energy, thereby reducing their total carbon footprint by 5 to 10 times. This load increase is largely offset by improved models based on 4Ms, machine learning-specific hardware and efficient data centers.
Google data shows that machine learning training and inference accounted for only 10% to 15% of Google’s overall energy use over the past three years, with 35% used annually. For inference, 25% is used for training.
To find improved machine learning models, Google uses Neural Architecture Search (NAS).
NAS is typically performed only once per problem domain/search space combination, and the resulting model can then be reused for hundreds of applications. The one-time cost of NAS is typically used on an ongoing basis. offset by emission reductions.
Researchers conducted a study to train the Transformer model.
To do this, they used Nvidia P100 GPUs in a typical data center with an energy mix similar to the global average, while using next-generation ML hardware such as TPUv4, performance improved over the P100 14 times.
At the same time, efficient cloud data centers save 1.4 times more energy than ordinary data centers, thus reducing total energy consumption by 83 times.
Additionally, data centers powered by low-carbon energy can reduce carbon emissions by a further nine times, for a total reduction of 747 times over four years.
The Google team believes that in the field of information technology, the life cycle costs of manufacturing computing devices of all types and sizes are much higher than the operating costs of machine learning.
Emissions Estimated manufacturing costs include the embedded carbon emitted by manufacturing all relevant components, from chips to data center buildings.
Of course, in addition to using the 4Ms approach, service providers and users can also take simple steps to improve their carbon footprint performance, such as:
Customers should analyze and reduce their energy use and carbon footprint by having data center providers report on data center efficiency and cleanliness of energy supply for each location.
Engineers should train models on the fastest processors in the greenest data centers, which are increasingly in the cloud.
Machine learning researchers should focus on designing more efficient models, such as taking advantage of sparsity or including retrieval to reduce the model.
Additionally, they should report their energy consumption and carbon impact. Not only will this encourage competition beyond model quality, but it will also ensure that their work is properly accounted for.
2. AI helps reduce carbon emissions
Although the large AI model is a major contributor to carbon emissions, cutting-edge technologies represented by AI are also making contributions to reducing carbon emissions. Make a contribution.
A study jointly conducted by Baidu and the consulting agency IDC (International Data Corporation) shows that the contribution of AI-related technologies to carbon reduction will increase year by year and will reach at least 70% by 2060. , the total amount of carbon reduction is expected to exceed 35 billion tons.
Taking the transportation industry as an example, the carbon emissions of China's transportation industry in 2020 are estimated to be 1.04 billion tons, accounting for 9% of the country's overall emissions.
In the process of driving the transportation industry to reduce carbon emissions, the use of congestion-relieving intelligent transportation technology based on intelligent information control can effectively improve the traffic efficiency of major urban road intersections. Therefore, cities with a population of tens of millions can reduce at least 41,600 tons of carbon emissions every year - which is equivalent to the carbon emissions of 14,000 private cars driving for one year.
Judging from current practice, the key to understanding and achieving emission reduction is to predict and monitor the effect of emission reduction, and AI has the function of predicting emissions and monitoring emissions in energy conservation and emission reduction. , three key applications to reduce emissions.
According to the "White Paper on Carbon Neutral Industry Development", in terms of predicting emissions, AI can predict future carbon emissions based on current emissions reduction efforts and needs, and at the same time determine carbon emissions. Lower emissions guidelines.
In terms of monitoring emissions, AI can track carbon footprint data in real time and collect data from all aspects of procurement, production, sales, operation and maintenance, logistics, etc., to improve monitoring accuracy.
In terms of reducing emissions, after AI collects data from each link, it can optimize and adjust the workflow of each link from a global perspective.
In fact, in terms of AI assisting carbon emission reduction, it has been applied in many domestic fields.
In the field of new energy, the prominent problem lies in its volatility, randomness and intermittent characteristics.
Use AI technology combined with simulation calculations to predict the instability of wind and photovoltaic power, such as: combining wind speed, wind direction, light intensity and other natural meteorological characteristics to reasonably predict future power generation forecast, output more accurate power generation plans to the power grid, and shield the uncertainty and instability of new energy sources under the technical layer.
For another example, the jurisdiction of the Water Group includes raw water, water production, water supply, drainage, sewage, water conservation, etc.
Take the water supply for residents as an example. If the water pressure is too high, it will require a lot of energy and the leakage rate of the pipe network will be high, which may cause pipe explosions; if the water pressure is too low, it may cause pipe explosions. It will cause inconvenience for residents to use water.
In order to solve this problem, the water group deployed hardware sensors underground to monitor water pressure and built a water brain. On the premise of ensuring safe and stable water supply, AI technology can be used to achieve intelligence. Voltage regulation control and energy consumption optimization.
Not only that, AI carbon reduction technology is also used in business scenarios with high energy consumption such as power plants, parks, and data centers to accurately predict and control their production electricity needs. Optimize power-consuming equipment and carbon footprint.
3. Conclusion
The advancement of AI technology has brought many conveniences to mankind, but we must also pay attention to environmental issues during development.
How AI can achieve sustainable development in the future and how AI can better support changes in the dual-carbon field are still issues that need to be solved by all industries.
The above is the detailed content of Behind the large AI model, there are staggering carbon emissions. For more information, please follow other related articles on the PHP Chinese website!

Hot AI Tools

Undresser.AI Undress
AI-powered app for creating realistic nude photos

AI Clothes Remover
Online AI tool for removing clothes from photos.

Undress AI Tool
Undress images for free

Clothoff.io
AI clothes remover

Video Face Swap
Swap faces in any video effortlessly with our completely free AI face swap tool!

Hot Article

Hot Tools

Notepad++7.3.1
Easy-to-use and free code editor

SublimeText3 Chinese version
Chinese version, very easy to use

Zend Studio 13.0.1
Powerful PHP integrated development environment

Dreamweaver CS6
Visual web development tools

SublimeText3 Mac version
God-level code editing software (SublimeText3)

Hot Topics
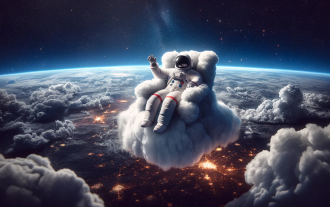
DALL-E 3 was officially introduced in September of 2023 as a vastly improved model than its predecessor. It is considered one of the best AI image generators to date, capable of creating images with intricate detail. However, at launch, it was exclus
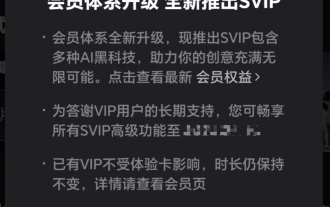
This site reported on June 27 that Jianying is a video editing software developed by FaceMeng Technology, a subsidiary of ByteDance. It relies on the Douyin platform and basically produces short video content for users of the platform. It is compatible with iOS, Android, and Windows. , MacOS and other operating systems. Jianying officially announced the upgrade of its membership system and launched a new SVIP, which includes a variety of AI black technologies, such as intelligent translation, intelligent highlighting, intelligent packaging, digital human synthesis, etc. In terms of price, the monthly fee for clipping SVIP is 79 yuan, the annual fee is 599 yuan (note on this site: equivalent to 49.9 yuan per month), the continuous monthly subscription is 59 yuan per month, and the continuous annual subscription is 499 yuan per year (equivalent to 41.6 yuan per month) . In addition, the cut official also stated that in order to improve the user experience, those who have subscribed to the original VIP
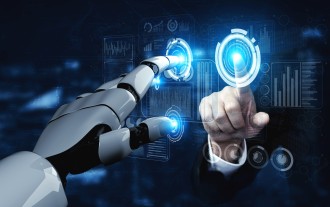
Large Language Models (LLMs) are trained on huge text databases, where they acquire large amounts of real-world knowledge. This knowledge is embedded into their parameters and can then be used when needed. The knowledge of these models is "reified" at the end of training. At the end of pre-training, the model actually stops learning. Align or fine-tune the model to learn how to leverage this knowledge and respond more naturally to user questions. But sometimes model knowledge is not enough, and although the model can access external content through RAG, it is considered beneficial to adapt the model to new domains through fine-tuning. This fine-tuning is performed using input from human annotators or other LLM creations, where the model encounters additional real-world knowledge and integrates it
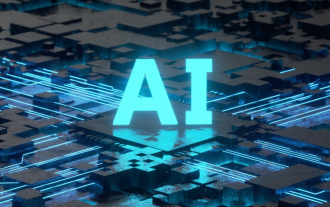
Editor |ScienceAI Question Answering (QA) data set plays a vital role in promoting natural language processing (NLP) research. High-quality QA data sets can not only be used to fine-tune models, but also effectively evaluate the capabilities of large language models (LLM), especially the ability to understand and reason about scientific knowledge. Although there are currently many scientific QA data sets covering medicine, chemistry, biology and other fields, these data sets still have some shortcomings. First, the data form is relatively simple, most of which are multiple-choice questions. They are easy to evaluate, but limit the model's answer selection range and cannot fully test the model's ability to answer scientific questions. In contrast, open-ended Q&A
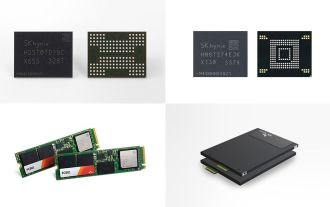
According to news from this site on August 1, SK Hynix released a blog post today (August 1), announcing that it will attend the Global Semiconductor Memory Summit FMS2024 to be held in Santa Clara, California, USA from August 6 to 8, showcasing many new technologies. generation product. Introduction to the Future Memory and Storage Summit (FutureMemoryandStorage), formerly the Flash Memory Summit (FlashMemorySummit) mainly for NAND suppliers, in the context of increasing attention to artificial intelligence technology, this year was renamed the Future Memory and Storage Summit (FutureMemoryandStorage) to invite DRAM and storage vendors and many more players. New product SK hynix launched last year
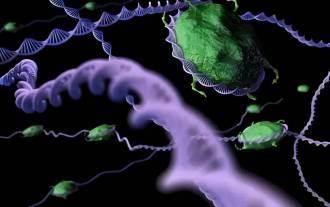
Editor | KX In the field of drug research and development, accurately and effectively predicting the binding affinity of proteins and ligands is crucial for drug screening and optimization. However, current studies do not take into account the important role of molecular surface information in protein-ligand interactions. Based on this, researchers from Xiamen University proposed a novel multi-modal feature extraction (MFE) framework, which for the first time combines information on protein surface, 3D structure and sequence, and uses a cross-attention mechanism to compare different modalities. feature alignment. Experimental results demonstrate that this method achieves state-of-the-art performance in predicting protein-ligand binding affinities. Furthermore, ablation studies demonstrate the effectiveness and necessity of protein surface information and multimodal feature alignment within this framework. Related research begins with "S
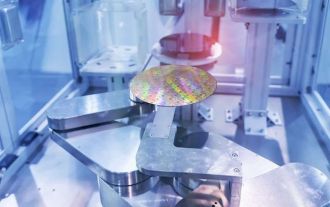
According to news from this website on July 5, GlobalFoundries issued a press release on July 1 this year, announcing the acquisition of Tagore Technology’s power gallium nitride (GaN) technology and intellectual property portfolio, hoping to expand its market share in automobiles and the Internet of Things. and artificial intelligence data center application areas to explore higher efficiency and better performance. As technologies such as generative AI continue to develop in the digital world, gallium nitride (GaN) has become a key solution for sustainable and efficient power management, especially in data centers. This website quoted the official announcement that during this acquisition, Tagore Technology’s engineering team will join GLOBALFOUNDRIES to further develop gallium nitride technology. G
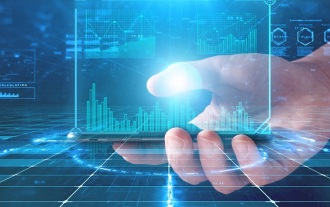
In the world of front-end development, VSCode has become the tool of choice for countless developers with its powerful functions and rich plug-in ecosystem. In recent years, with the rapid development of artificial intelligence technology, AI code assistants on VSCode have sprung up, greatly improving developers' coding efficiency. AI code assistants on VSCode have sprung up like mushrooms after a rain, greatly improving developers' coding efficiency. It uses artificial intelligence technology to intelligently analyze code and provide precise code completion, automatic error correction, grammar checking and other functions, which greatly reduces developers' errors and tedious manual work during the coding process. Today, I will recommend 12 VSCode front-end development AI code assistants to help you in your programming journey.
