


Nine common mistakes data scientists make when using Python
Best practices are learned from mistakes, so here we summarize some of the most common mistakes we encounter and provide methods, ideas and resources on how to best solve them.
1. Not using a virtual environment
This is not a coding problem in itself, but I still think it is a very good idea to isolate the environment for each type of project practice.
Why use a dedicated environment for each project?
The first reason is the problem of Python's own package management. We want to minimize conflicts between packages and versions.
Another reason is that our code and dependencies can be easily deployed to any location
Using a virtual environment can start from Anaconda or Pipenv. If you want to go deeper then Docker is the first choice.
2. Overuse of Jupyter Notebooks
Notebooks are great for educational purposes and doing some quick and complex analysis work, but they don’t serve as a good IDE.
A good IDE is a real weapon when dealing with data science tasks and can greatly improve your work efficiency.
Notebooks are great for doing experiments and making it easy to show the results to others. But it's error-prone, and when it comes to executing long-term, collaborative, and deployable projects, you're better off using an IDE like VScode, Pycharm, Spyder, etc.
3. Use absolute rather than relative paths
The biggest problem with absolute paths is that they cannot be deployed conveniently. The main way to solve this problem is to set the working directory to the project root directory and do not The project contains files outside the project directory and uses relative paths for all paths in the code.
import pandas as pd import numpy as np import os #### 错误的方式 ##### excel_path1 = "C:\Users\abdelilah\Desktop\mysheet1.xlsx" excel_path2 = "C:\Users\abdelilah\Desktop\mysheet2.xlsx" mydf1 = pd.read_excel(excel_path1) mydf2 = pd.read_excel(excel_path2) #### 正确的方式 #### DATA_DIR = "data" #将要读取的文件复制到data目录 crime06_filename = "CrimeOneYearofData_2006.xlsx" crime07_filename = "CrimeOneYearofData_2007.xlsx" crime06_df = pd.read_excel(os.path.join(DATA_DIR, crime06_filename)) crime07_df = pd.read_excel(os.path.join(DATA_DIR, crime07_filename))
4. Not handling warnings
When our code is able to run but produces strange warning messages, we are happy to finally get the code to run and receive meaningful output. But do we need to deal with these warnings?
First of all, warnings themselves are not errors, but they are reminders of potential errors or problems. Warnings appear when something in your code works successfully but maybe not the way it's intended.
The most common warnings I encounter are Pandas' "SettingwithCopyWarning" and "DeprecationWarning".
The biggest reason for SettingwithCopyWarning is the warning that occurs when Pandas detects chained assignment (Chained Assignment). We should avoid assigning values to the results of chained indexes, because this operation may or may not report a warning. .
DeprecationWarning usually indicates that Pandas has deprecated some functionality and that your code will break when using a later version.
The advice here is not to deal with all warnings, but you must understand the reasons for all warnings, know which warnings can be ignored in a specific project, and the occurrence of those warnings will affect the results. It has an impact and should be avoided.
5. List comprehension is not used (rarely used)
List comprehension is a very powerful feature of Python. Many for loops can be replaced with list comprehensions that are more readable, more Pythonic, and faster.
You can see below a sample code designed to read a CSV file in a directory. As you can see, Tim is easy to maintain when using list comprehensions.
import pandas as pd import os DATA_PATH = "data" filename_list = os.listdir(DATA_PATH) #### 不好的方法 ##### csv_list = [] for fileaname in filename_list: csv_list.append(pd.read_csv(os.path.join(DATA_PATH, filename))) #### 建议 #### csv_list = [pd.read_csv(os.path.join(DATA_PATH, filename)) for filename in filename_list] list comprehensions csv_list = [pd.read_csv(os.path.join(DATA_PATH, filename)) for filename in filename_list if filename.endswith(".csv")]
6. Do not use type annotations
Type annotations (or type hints) are ways to assign types to variables. When the IDE prompts IntelliSense, it can provide us with the type of the indicator variable/parameter. This can not only improve the speed of our development, but also be of great help to us in reading the code
def mystery_combine(a, b, times): return (a + b) * times
If written like this, we don’t know the types of a, b and times at all
def mystery_combine(a: str, b: str, times: int) -> str: return (a + b) * times
But With the addition of type annotations, we know that a and b are strings and times are integers
It should be noted that python introduced type annotations in version 3.5, and python will not check type annotations during execution. , it just provides a convenient static type checking tool for the IDE to perform static type checking on dynamic languages to avoid some potential errors.
7. Pandas code is not standardized
Method chaining is a great feature of pandas, but if there are many operations included in one line, the code may become unreadable.
A trick to make this approach even easier is to put the expression in parentheses, so you can use one line for each component of the expression.
var_list = ["clicks", "time_spent"] var_list_Q = [varname + "_Q" for varname in var_list] #不可读的方法 df_Q = df.groupby("id").rolling(window=3, min_periods=1, on="yearmonth[var_list].mean().reset_index().rename(columns=dict(zip(var_list, var_list_Q))) #可读性强的方法 df_Q = ( df .groupby("id") .rolling(window=3, min_periods=1, on="yearmonth")[var_list] .mean() .reset_index() .rename(columns=dict(zip(var_list, var_list_Q))))
8. Not complying with PEP conventions
When you first start programming in Python, the code may be crude and unreadable. This is because we do not have our own design rules to make our The code looks better. It would be laborious and laborious to design such rules ourselves and require a lot of practice. Fortunately, Python has officially specified rules: PEP, which is Python's official style guide.
Although PEP rules are many and cumbersome, we can ignore some PEP rules, but they can be used in 90% of the code.
9. You don’t use coding assistance tools
Do you want to significantly improve your productivity in coding? Start using Coding Assist, which helps with clever auto-completion, opening documents, and providing suggestions for improving your code.
pylance, Kite, tabnine, and copilot are all very good choices.
The above is the detailed content of Nine common mistakes data scientists make when using Python. For more information, please follow other related articles on the PHP Chinese website!

Hot AI Tools

Undresser.AI Undress
AI-powered app for creating realistic nude photos

AI Clothes Remover
Online AI tool for removing clothes from photos.

Undress AI Tool
Undress images for free

Clothoff.io
AI clothes remover

Video Face Swap
Swap faces in any video effortlessly with our completely free AI face swap tool!

Hot Article

Hot Tools

Notepad++7.3.1
Easy-to-use and free code editor

SublimeText3 Chinese version
Chinese version, very easy to use

Zend Studio 13.0.1
Powerful PHP integrated development environment

Dreamweaver CS6
Visual web development tools

SublimeText3 Mac version
God-level code editing software (SublimeText3)

Hot Topics
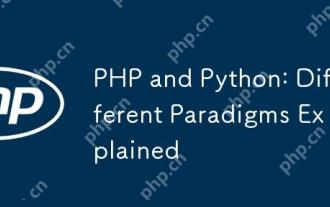
PHP is mainly procedural programming, but also supports object-oriented programming (OOP); Python supports a variety of paradigms, including OOP, functional and procedural programming. PHP is suitable for web development, and Python is suitable for a variety of applications such as data analysis and machine learning.
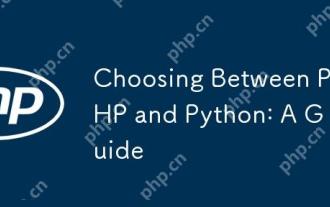
PHP is suitable for web development and rapid prototyping, and Python is suitable for data science and machine learning. 1.PHP is used for dynamic web development, with simple syntax and suitable for rapid development. 2. Python has concise syntax, is suitable for multiple fields, and has a strong library ecosystem.
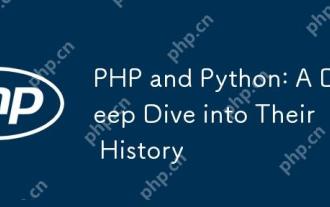
PHP originated in 1994 and was developed by RasmusLerdorf. It was originally used to track website visitors and gradually evolved into a server-side scripting language and was widely used in web development. Python was developed by Guidovan Rossum in the late 1980s and was first released in 1991. It emphasizes code readability and simplicity, and is suitable for scientific computing, data analysis and other fields.
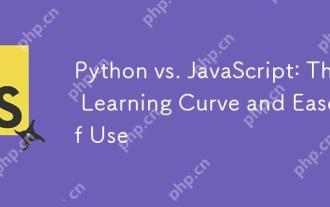
Python is more suitable for beginners, with a smooth learning curve and concise syntax; JavaScript is suitable for front-end development, with a steep learning curve and flexible syntax. 1. Python syntax is intuitive and suitable for data science and back-end development. 2. JavaScript is flexible and widely used in front-end and server-side programming.
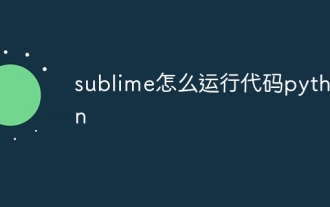
To run Python code in Sublime Text, you need to install the Python plug-in first, then create a .py file and write the code, and finally press Ctrl B to run the code, and the output will be displayed in the console.
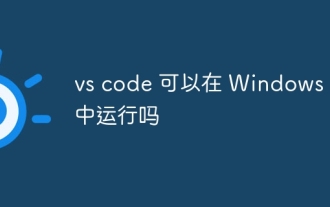
VS Code can run on Windows 8, but the experience may not be great. First make sure the system has been updated to the latest patch, then download the VS Code installation package that matches the system architecture and install it as prompted. After installation, be aware that some extensions may be incompatible with Windows 8 and need to look for alternative extensions or use newer Windows systems in a virtual machine. Install the necessary extensions to check whether they work properly. Although VS Code is feasible on Windows 8, it is recommended to upgrade to a newer Windows system for a better development experience and security.
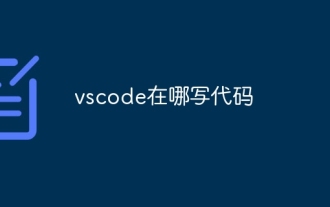
Writing code in Visual Studio Code (VSCode) is simple and easy to use. Just install VSCode, create a project, select a language, create a file, write code, save and run it. The advantages of VSCode include cross-platform, free and open source, powerful features, rich extensions, and lightweight and fast.
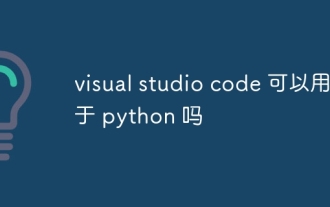
VS Code can be used to write Python and provides many features that make it an ideal tool for developing Python applications. It allows users to: install Python extensions to get functions such as code completion, syntax highlighting, and debugging. Use the debugger to track code step by step, find and fix errors. Integrate Git for version control. Use code formatting tools to maintain code consistency. Use the Linting tool to spot potential problems ahead of time.
