


Debugging Pytorch memory leak issues using context decorators
Decorators are specific implementations of python context managers. This article will illustrate how to use them through an example of pytorch GPU debugging. While it may not work in every situation, I found them to be very useful.
Debugging Memory Leak Issues
There are many ways to debug memory leaks. This article will demonstrate a useful method for identifying problematic lines in your code. This method can help to find the specific location in a concise way.
Manual debugging line by line
If you encounter a problem, a classic and commonly used method is to use a debugger to check line by line, such as the following example:
- Look for code snippets on how to calculate the total number of all tensors in pytorch in the search engine, such as: tensor-counter-snippet
- Set breakpoints in the code
- Use tensor-counter -snippet to get the total count of tensors
- Use the debugger to perform the next step
- Rerun tensor-counter-snippet and check if the tensor count is increased
- Duplicate The steps above
It works, but it sounds like a lot of trouble. We can encapsulate it into a function, which can be called when needed, so that there is almost no need to modify the existing code, which leads us to introduce the function of the decorator.
Python Decorator
Decorators can be wrapped in any part of the code. Here we use the decorator to check if there are additional tensors. In addition, we also need a counter because the number of tensors needs to be calculated before and after execution. The pattern looks like this:
def memleak_wrapper(func): def wrap(*args, **kwargs): print("num tensors start is ...") out = func(*args, **kwargs) print("num tensors end is ...") return out return wrap@memleak_wrapper def function_to_debug(x): print(f"put line(s) of code here. Input is {x}") out = x + 10 return outout = function_to_debug(x=1000) print(f"out is {out}") #输入类似这样 #num tensors start is ... #put line(s) of code here. Input is 1000 #num tensors end is ... #outis 1010
To run this code, we need to put the line of code we want to check into a function (function_to_debug). But this is not the best because we still need to insert a lot of code manually. The other thing is that if the code block generates more than one variable, you need to find additional solutions to use these downstream variables.
Context Decorator
In order to solve the above problem, we can use context manager instead of function decorator. The most widely used example of a context manager is instantiating a context using the with statement. The most common one used to be:
with open("file") as f: …
Using Python's contextlib library, Python users can easily create their own context managers. So in this article we will use ContextDecorator to complete the work we tried to use decorator above. Because it is easier to develop and easier to use:
from contextlib import ContextDecorator class check_memory_leak_context(ContextDecorator): def __enter__(self): print('Starting') return self def __exit__(self, *exc): print('Finishing') return False
ContextDecorator has 2 methods: enter() and exit() which are called when we enter or exit the context. The *exc parameter in __exit__ represents any incoming exception.
Now we use it to solve the problem mentioned above.
Use ContextDecorator to find memory leaks
Because we need to calculate the total number of tensors, we encapsulate the calculation process into a function get_n_tensors(), so that the tensors can be calculated at the beginning and end of the context Quantity:
class check_memory_leak_context(ContextDecorator): def __enter__(self): self.start = get_n_tensors() return self def __exit__(self, *exc): self.end = get_n_tensors() increase = self.end — self.start if increase > 0: print(f”num tensors increased with" f"{self.end — self.start} !”) else: print(”no added tensors”) return False
If there is an increase, print it to the console.
get_n_tensor() uses the garbage collector (gc) and is customized for pytorch, but can be easily modified for other libraries:
import gc def get_n_tensors(): tensors= [] for obj in gc.get_objects(): try: if (torch.is_tensor(obj) or (hasattr(obj, ‘data’) and torch.is_tensor(obj.data))): tensors.append(obj) except: pass return len(tensors)
It can be used now, and we can use it for any A line (or block) of code uses this context:
x = arbitrary_operation(x) ... with check_memory_leak_context(): y = x[0].permute(1, 2, 0).cpu().detach().numpy() x = some_harmless_operation() ... x = another_arbitrary_operation(x)
If a new tensor is created within the line wrapped by the context decorator, it will be printed.
Summary
This is a very good code snippet that you can put in a separate file during development. Here is the complete code for this article:
https://gist.github.com/MarkTension/4783697ebd5212ba500cdd829b364338
Finally I hope this small article can help you understand what a context manager is, how to use context decorators, and how to apply them to debugging pytorch .
The above is the detailed content of Debugging Pytorch memory leak issues using context decorators. For more information, please follow other related articles on the PHP Chinese website!

Hot AI Tools

Undresser.AI Undress
AI-powered app for creating realistic nude photos

AI Clothes Remover
Online AI tool for removing clothes from photos.

Undress AI Tool
Undress images for free

Clothoff.io
AI clothes remover

Video Face Swap
Swap faces in any video effortlessly with our completely free AI face swap tool!

Hot Article

Hot Tools

Notepad++7.3.1
Easy-to-use and free code editor

SublimeText3 Chinese version
Chinese version, very easy to use

Zend Studio 13.0.1
Powerful PHP integrated development environment

Dreamweaver CS6
Visual web development tools

SublimeText3 Mac version
God-level code editing software (SublimeText3)

Hot Topics
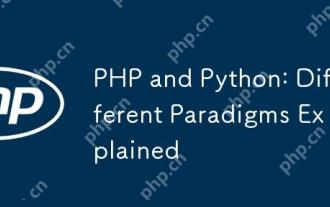
PHP is mainly procedural programming, but also supports object-oriented programming (OOP); Python supports a variety of paradigms, including OOP, functional and procedural programming. PHP is suitable for web development, and Python is suitable for a variety of applications such as data analysis and machine learning.
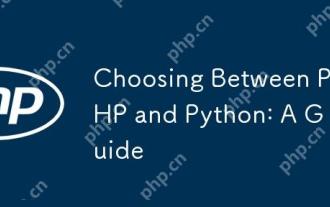
PHP is suitable for web development and rapid prototyping, and Python is suitable for data science and machine learning. 1.PHP is used for dynamic web development, with simple syntax and suitable for rapid development. 2. Python has concise syntax, is suitable for multiple fields, and has a strong library ecosystem.
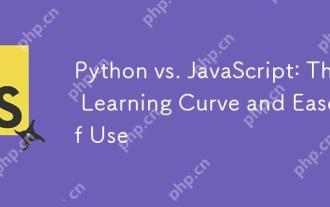
Python is more suitable for beginners, with a smooth learning curve and concise syntax; JavaScript is suitable for front-end development, with a steep learning curve and flexible syntax. 1. Python syntax is intuitive and suitable for data science and back-end development. 2. JavaScript is flexible and widely used in front-end and server-side programming.
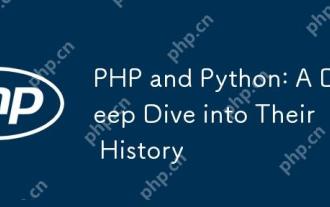
PHP originated in 1994 and was developed by RasmusLerdorf. It was originally used to track website visitors and gradually evolved into a server-side scripting language and was widely used in web development. Python was developed by Guidovan Rossum in the late 1980s and was first released in 1991. It emphasizes code readability and simplicity, and is suitable for scientific computing, data analysis and other fields.
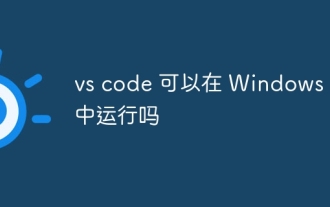
VS Code can run on Windows 8, but the experience may not be great. First make sure the system has been updated to the latest patch, then download the VS Code installation package that matches the system architecture and install it as prompted. After installation, be aware that some extensions may be incompatible with Windows 8 and need to look for alternative extensions or use newer Windows systems in a virtual machine. Install the necessary extensions to check whether they work properly. Although VS Code is feasible on Windows 8, it is recommended to upgrade to a newer Windows system for a better development experience and security.
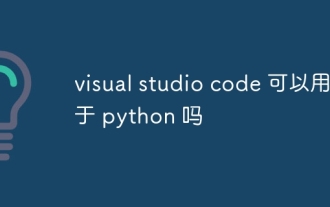
VS Code can be used to write Python and provides many features that make it an ideal tool for developing Python applications. It allows users to: install Python extensions to get functions such as code completion, syntax highlighting, and debugging. Use the debugger to track code step by step, find and fix errors. Integrate Git for version control. Use code formatting tools to maintain code consistency. Use the Linting tool to spot potential problems ahead of time.
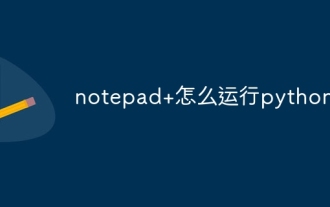
Running Python code in Notepad requires the Python executable and NppExec plug-in to be installed. After installing Python and adding PATH to it, configure the command "python" and the parameter "{CURRENT_DIRECTORY}{FILE_NAME}" in the NppExec plug-in to run Python code in Notepad through the shortcut key "F6".
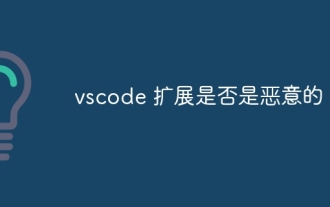
VS Code extensions pose malicious risks, such as hiding malicious code, exploiting vulnerabilities, and masturbating as legitimate extensions. Methods to identify malicious extensions include: checking publishers, reading comments, checking code, and installing with caution. Security measures also include: security awareness, good habits, regular updates and antivirus software.
