


Mid-year review: Ten hot data science and machine learning startups in 2022
Enterprises are dealing with an increasing amount of data, whether generated within the organization or collected from external sources. How to find effective ways to analyze and "operate" all this data to gain a competitive advantage is changing. Becoming more and more challenging.
This has also driven the demand for new tools and information technologies in the field of data science and machine learning. According to the Fortune Business Insights Report, the global machine learning market size will reach US$15.44 billion in 2021 alone, and is expected to reach US$21.17 billion this year and grow to US$209.91 billion by 2029, with a compound annual growth rate of 38.8%.
At the same time, according to a joint market research report, the global data science platform market size was US$4.7 billion in 2020 and is expected to reach US$79.7 billion by 2030, with a compound annual growth rate of 33.6%.
The concepts of "data science" and "machine learning" are sometimes easily confused or even used interchangeably, but in fact they are two different concepts and there is a correlation between them because data science Practice is key to machine learning projects.
According to the Master's in Data Science website, data science is a field of study that uses scientific methods to extract meaning and insights from data, including developing data analysis strategies, preparing data for analysis, developing data visualizations, and constructing data model, etc.
According to the Fortune Business Insights Report, machine learning is the next sub-section of the broader field of artificial intelligence. It uses data analysis to teach computers how to learn using models based on algorithms and data. That is Mimic the way humans learn.
The market demand for data science and machine learning tools has spawned a large number of start-up companies developing cutting-edge technologies in the field of data science or machine learning, including the following 10 companies:
1, Aporia
- Founder, CEO: Liran Hason
- Headquarters: Tel Aviv, Israel
- Website: https://www. aporia.com/
Founded in 2020, Aporia has developed a full-stack, highly customizable machine learning observability platform that data science and machine learning teams can use to monitor and debug , interpret and improve machine learning models and data.
Aporia raised $5 million in seed funding, followed by $25 million in Series A funding in March 2022.
Aporia will use the funds to triple its employee size by 2023, as well as expand its business scale in the United States and the range of use scenarios covered by its technology.
2. Black Crow AI
- Founder and CEO: Richard Harris
- Headquarters: New York, USA
- Website: https://www.blackcrow.ai/
Black Crow AI has developed a machine learning platform for e-commerce applications for online direct-to-consumer merchants. Their model predicts visitor behavior and future value when shopping. The software analyzes billions of data points in real time to enhance customer experience, manage churn, and optimize marketing spend.
Black Crow AI was founded in 2020 and received US$25 million in Series A financing in March this year, bringing the total financing amount to US$30 million. Black Crow AI will use the funds to accelerate the discovery of new, usable machine learning scenarios in digital commerce and adjacent verticals.
3、 Comet
- Co-founder, CEO: Gideon Mendels
- Headquarters: New York, USA
- Website: https://www.comet.ml/site/
Comet’s platform provides data scientists and data science teams with the ability to manage and optimize the entire machine learning lifecycle, including building and training models, experiment tracking, and model production monitoring to increase visibility, collaboration, and productivity.
Comet, founded in 2017, raised $50 million in Series B funding in November last year, but the company said it has grown its annual recurring revenue fivefold and its global workforce by threefold. Customers include Ancestry, Etsy, Uber and Zappos.
4, dotData
- Founder and CEO: Ryohei Fujimaki
- Headquarters: San Mateo, California, USA
- Website: https://dotdata.com/
dotData’s software provides automated feature engineering and enterprise AI automation functions for building artificial intelligence or machine learning models. In the machine learning development process, feature engineering is a critical step in finding important hidden patterns in the data used to develop and train machine learning models).
dotData’s flagship product is dotData Enterprise predictive analytics automation software, and it also offers related products including dotData Cloud AI automation platform, dotData Py and dotData Py Lite tools, and dotData Stream for real-time AI models.
DotData was founded in 2018 as a company spun off from NEC. It received US$31.6 million in Series B financing in April this year, bringing the total financing amount to US$74.6 million. dotData has been using these external funds to accelerate its own product development.
5, Neuton
- CEO: Andrey Korobitsyn
- Headquarters: San Jose, California, USA
- Website: https://neuton.ai/
Neuton, founded in 2021, develops an automated, no-code “tinyML” platform and other tools for developing microcontroller-embeddable Tiny machine learning models to make edge devices smart.
Neuton’s technology is entering a wide range of applications, including predictive maintenance of compressor water pumps, preventing grid overload, room occupancy detection, handwriting recognition on handheld devices, gearbox failure prediction and water pollution monitoring equipment, etc. .
6, Pinecone
- Founder and CEO: Edo Liberty
- Headquarters: San Francisco, USA
- Website: https://www.pinecone.io/
Pinecone develops a vector database and search technology to power artificial intelligence and machine learning applications. Last October, Pinecone launched Pinecone 2.0, taking the software from research labs to production applications.
Pinecone was founded in 2019. It came out of stealth mode last year and received US$10 million in seed round financing in January 2021 and US$28 million in Series A financing in March this year.
Gartner named Pinecone a "Cool Vendor" in artificial intelligence and machine learning data in 2021.
7、Snorkel AI
- Co-founder, CEO: Alex Ratner
- Headquarters: Redwood City, California, USA
- Website: https://snorkel.ai/
Snorkel was founded in 2019 and originated from the Artificial Intelligence Laboratory of Stanford University. The five founders of the company were working in this laboratory to solve the problem of lack of labeled training data in machine learning development.
Snorkel launched Snorkel Flow in March this year, a data-centric system that accelerates the development of artificial intelligence and machine learning through the use of programmatic tags, which are also used for data preparation and machine learning model development and A critical step in the training process.
Snorkel’s valuation topped $1 billion in August 2021, when the startup secured $85 million in Series C funding to expand its engineering and sales teams and accelerate platform development.
8, Striveworks
- CEO: James Rebesco
- Headquarters: Austin, Texas, USA
- Website: https://striveworks.us/
Striveworks was founded in 2018 and mainly develops MLOps technology for those highly regulated industries.
Striveworks’ flagship product, Chariot Platform, is primarily designed for operational data science, easing the burden of creating artificial intelligence or machine learning solutions. The system oversees the process of data acquisition and preparation, as well as the training, validation, deployment, and monitoring of machine learning models—all in the cloud, on-premises, or at the network edge.
9, Tecton
- Co-founder, CEO: Mike Del Balso
- Headquarters: San Francisco, USA
- Website: https://www.tecton.ai/
Tecton has developed a machine learning function library platform that can shorten the deployment speed of machine learning applications from months to a few minute. Tecton's technology can automatically convert raw data, generate training data sets, and provide large-scale online inference capabilities.
Tecton was founded in 2019. The founder developed the Uber Michelangelo machine learning platform. Later, the company came out of stealth mode in April 2020.
10, Verta
- CEO: Manasi Vartak
- Headquarters: Menlo Park, California, USA
- Website: https://www.verta.ai/
Verta’s platform can be used by data science and machine learning teams to deploy and operate throughout the entire artificial intelligence and machine learning model lifecycle , management and monitoring models.
Verta was named a “Cool Vendor” for core AI technology by Gartner this month.
The above is the detailed content of Mid-year review: Ten hot data science and machine learning startups in 2022. For more information, please follow other related articles on the PHP Chinese website!

Hot AI Tools

Undresser.AI Undress
AI-powered app for creating realistic nude photos

AI Clothes Remover
Online AI tool for removing clothes from photos.

Undress AI Tool
Undress images for free

Clothoff.io
AI clothes remover

Video Face Swap
Swap faces in any video effortlessly with our completely free AI face swap tool!

Hot Article

Hot Tools

Notepad++7.3.1
Easy-to-use and free code editor

SublimeText3 Chinese version
Chinese version, very easy to use

Zend Studio 13.0.1
Powerful PHP integrated development environment

Dreamweaver CS6
Visual web development tools

SublimeText3 Mac version
God-level code editing software (SublimeText3)

Hot Topics
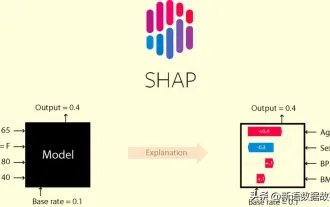
In the fields of machine learning and data science, model interpretability has always been a focus of researchers and practitioners. With the widespread application of complex models such as deep learning and ensemble methods, understanding the model's decision-making process has become particularly important. Explainable AI|XAI helps build trust and confidence in machine learning models by increasing the transparency of the model. Improving model transparency can be achieved through methods such as the widespread use of multiple complex models, as well as the decision-making processes used to explain the models. These methods include feature importance analysis, model prediction interval estimation, local interpretability algorithms, etc. Feature importance analysis can explain the decision-making process of a model by evaluating the degree of influence of the model on the input features. Model prediction interval estimate
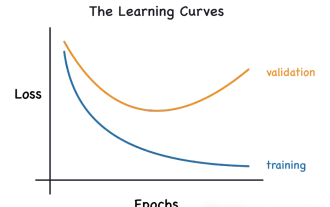
This article will introduce how to effectively identify overfitting and underfitting in machine learning models through learning curves. Underfitting and overfitting 1. Overfitting If a model is overtrained on the data so that it learns noise from it, then the model is said to be overfitting. An overfitted model learns every example so perfectly that it will misclassify an unseen/new example. For an overfitted model, we will get a perfect/near-perfect training set score and a terrible validation set/test score. Slightly modified: "Cause of overfitting: Use a complex model to solve a simple problem and extract noise from the data. Because a small data set as a training set may not represent the correct representation of all data." 2. Underfitting Heru
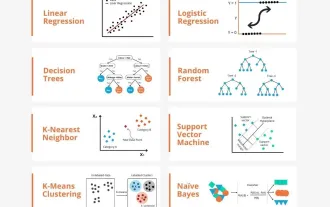
In layman’s terms, a machine learning model is a mathematical function that maps input data to a predicted output. More specifically, a machine learning model is a mathematical function that adjusts model parameters by learning from training data to minimize the error between the predicted output and the true label. There are many models in machine learning, such as logistic regression models, decision tree models, support vector machine models, etc. Each model has its applicable data types and problem types. At the same time, there are many commonalities between different models, or there is a hidden path for model evolution. Taking the connectionist perceptron as an example, by increasing the number of hidden layers of the perceptron, we can transform it into a deep neural network. If a kernel function is added to the perceptron, it can be converted into an SVM. this one
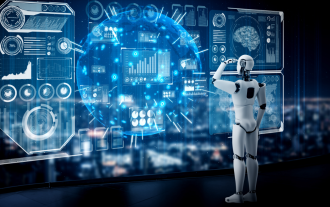
In the 1950s, artificial intelligence (AI) was born. That's when researchers discovered that machines could perform human-like tasks, such as thinking. Later, in the 1960s, the U.S. Department of Defense funded artificial intelligence and established laboratories for further development. Researchers are finding applications for artificial intelligence in many areas, such as space exploration and survival in extreme environments. Space exploration is the study of the universe, which covers the entire universe beyond the earth. Space is classified as an extreme environment because its conditions are different from those on Earth. To survive in space, many factors must be considered and precautions must be taken. Scientists and researchers believe that exploring space and understanding the current state of everything can help understand how the universe works and prepare for potential environmental crises
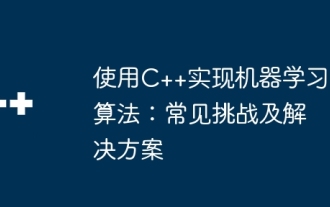
Common challenges faced by machine learning algorithms in C++ include memory management, multi-threading, performance optimization, and maintainability. Solutions include using smart pointers, modern threading libraries, SIMD instructions and third-party libraries, as well as following coding style guidelines and using automation tools. Practical cases show how to use the Eigen library to implement linear regression algorithms, effectively manage memory and use high-performance matrix operations.
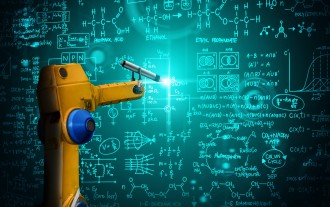
Machine learning is an important branch of artificial intelligence that gives computers the ability to learn from data and improve their capabilities without being explicitly programmed. Machine learning has a wide range of applications in various fields, from image recognition and natural language processing to recommendation systems and fraud detection, and it is changing the way we live. There are many different methods and theories in the field of machine learning, among which the five most influential methods are called the "Five Schools of Machine Learning". The five major schools are the symbolic school, the connectionist school, the evolutionary school, the Bayesian school and the analogy school. 1. Symbolism, also known as symbolism, emphasizes the use of symbols for logical reasoning and expression of knowledge. This school of thought believes that learning is a process of reverse deduction, through existing
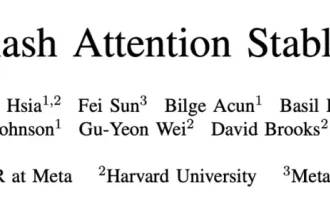
MetaFAIR teamed up with Harvard to provide a new research framework for optimizing the data bias generated when large-scale machine learning is performed. It is known that the training of large language models often takes months and uses hundreds or even thousands of GPUs. Taking the LLaMA270B model as an example, its training requires a total of 1,720,320 GPU hours. Training large models presents unique systemic challenges due to the scale and complexity of these workloads. Recently, many institutions have reported instability in the training process when training SOTA generative AI models. They usually appear in the form of loss spikes. For example, Google's PaLM model experienced up to 20 loss spikes during the training process. Numerical bias is the root cause of this training inaccuracy,
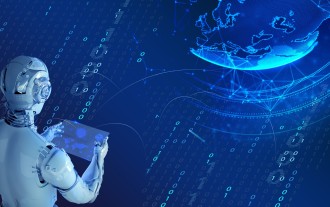
Translator | Reviewed by Li Rui | Chonglou Artificial intelligence (AI) and machine learning (ML) models are becoming increasingly complex today, and the output produced by these models is a black box – unable to be explained to stakeholders. Explainable AI (XAI) aims to solve this problem by enabling stakeholders to understand how these models work, ensuring they understand how these models actually make decisions, and ensuring transparency in AI systems, Trust and accountability to address this issue. This article explores various explainable artificial intelligence (XAI) techniques to illustrate their underlying principles. Several reasons why explainable AI is crucial Trust and transparency: For AI systems to be widely accepted and trusted, users need to understand how decisions are made
