


With the blessing of drift-aware dynamic neural network, the new framework of time domain generalization far exceeds the domain generalization & adaptation method.
In the Domain Generalization (DG) task, when the distribution of the domain continuously changes with the environment, how to accurately capture the change and its impact on the model is a very important but also extremely challenging issue. To this end, Professor Zhao Liang's team from Emory University proposed a time domain generalization framework DRAIN based on Bayesian theory, which uses recursive networks to learn the drift of time dimension domain distribution, and combines dynamic neural networks and graph generation technology. Maximize the expression ability of the model and achieve model generalization and prediction in unknown fields in the future. This work has been selected into ICLR 2023 Oral (Top 5% among accepted papers).
The above is the detailed content of With the blessing of drift-aware dynamic neural network, the new framework of time domain generalization far exceeds the domain generalization & adaptation method.. For more information, please follow other related articles on the PHP Chinese website!

Hot AI Tools

Undresser.AI Undress
AI-powered app for creating realistic nude photos

AI Clothes Remover
Online AI tool for removing clothes from photos.

Undress AI Tool
Undress images for free

Clothoff.io
AI clothes remover

Video Face Swap
Swap faces in any video effortlessly with our completely free AI face swap tool!

Hot Article

Hot Tools

Notepad++7.3.1
Easy-to-use and free code editor

SublimeText3 Chinese version
Chinese version, very easy to use

Zend Studio 13.0.1
Powerful PHP integrated development environment

Dreamweaver CS6
Visual web development tools

SublimeText3 Mac version
God-level code editing software (SublimeText3)

Hot Topics
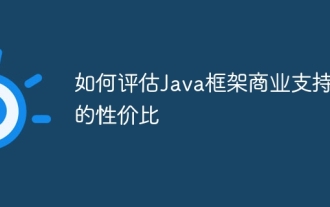
Evaluating the cost/performance of commercial support for a Java framework involves the following steps: Determine the required level of assurance and service level agreement (SLA) guarantees. The experience and expertise of the research support team. Consider additional services such as upgrades, troubleshooting, and performance optimization. Weigh business support costs against risk mitigation and increased efficiency.
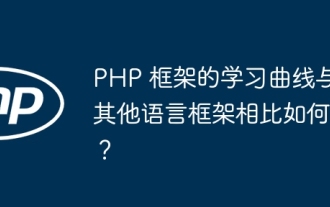
The learning curve of a PHP framework depends on language proficiency, framework complexity, documentation quality, and community support. The learning curve of PHP frameworks is higher when compared to Python frameworks and lower when compared to Ruby frameworks. Compared to Java frameworks, PHP frameworks have a moderate learning curve but a shorter time to get started.

The lightweight PHP framework improves application performance through small size and low resource consumption. Its features include: small size, fast startup, low memory usage, improved response speed and throughput, and reduced resource consumption. Practical case: SlimFramework creates REST API, only 500KB, high responsiveness and high throughput
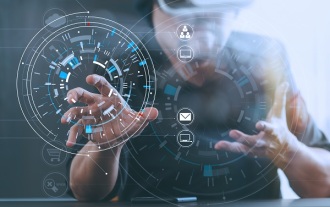
I believe that friends who love technology and have a strong interest in AI like the author must be familiar with convolutional neural networks, and must have been confused by such an "advanced" name for a long time. The author will enter the world of convolutional neural networks from scratch today ~ share it with everyone! Before we dive into convolutional neural networks, let’s take a look at how images work. Image Principle Images are represented in computers by numbers (0-255), and each number represents the brightness or color information of a pixel in the image. Among them: Black and white image: Each pixel has only one value, and this value varies between 0 (black) and 255 (white). Color image: Each pixel contains three values, the most common is the RGB (Red-Green-Blue) model, which is red, green and blue
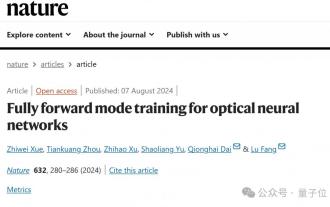
Using light to train neural networks, Tsinghua University results were recently published in Nature! What should I do if I cannot apply the backpropagation algorithm? They proposed a Fully Forward Mode (FFM) training method that directly performs the training process in the physical optical system, overcoming the limitations of traditional digital computer simulations. To put it simply, it used to be necessary to model the physical system in detail and then simulate these models on a computer to train the network. The FFM method eliminates the modeling process and allows the system to directly use experimental data for learning and optimization. This also means that training no longer needs to check each layer from back to front (backpropagation), but can directly update the parameters of the network from front to back. To use an analogy, like a puzzle, backpropagation

The AIxiv column is a column where this site publishes academic and technical content. In the past few years, the AIxiv column of this site has received more than 2,000 reports, covering top laboratories from major universities and companies around the world, effectively promoting academic exchanges and dissemination. If you have excellent work that you want to share, please feel free to contribute or contact us for reporting. Submission email: liyazhou@jiqizhixin.com; zhaoyunfeng@jiqizhixin.com The authors of this article are all from the team of Associate Professor Huang Lei, School of Artificial Intelligence, Beihang University and National Key Laboratory of Complex Critical Software Environment. The first author, Ni Yunhao, is a first-year graduate student, the second author, Guo Yuxin, is a third-year graduate student, the third author, Jia Junlong, is a second-year graduate student, and the corresponding author is Associate Professor Huang Lei
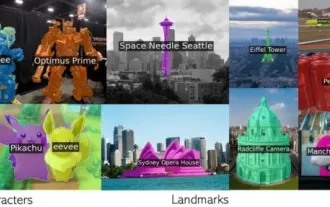
CLIP is called cyclically to effectively segment countless concepts without additional training. Any phrase including movie characters, landmarks, brands, and general categories. This new result of the joint team of Oxford University and Google Research has been accepted by CVPR2024 and the code has been open sourced. The team proposed a new technology called CLIPasRNN (CaR for short), which solves several key problems in the field of open vocabulary image segmentation: No training data is required: traditional methods require a large number of mask annotations or image-text datasets for fine-tuning, CaR The technology works without any additional training data. Open vocabulary limitations: Pre-trained visual-language models (VLMs) are limited in their ability to handle open vocabularies after fine-tuning. C
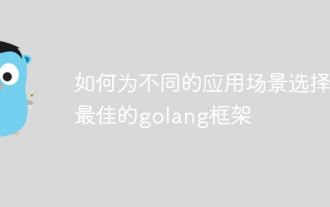
Choose the best Go framework based on application scenarios: consider application type, language features, performance requirements, and ecosystem. Common Go frameworks: Gin (Web application), Echo (Web service), Fiber (high throughput), gorm (ORM), fasthttp (speed). Practical case: building REST API (Fiber) and interacting with the database (gorm). Choose a framework: choose fasthttp for key performance, Gin/Echo for flexible web applications, and gorm for database interaction.
