


BLOOM can create a new culture for AI research, but challenges remain
Translator|Li Rui
Reviewer|Sun Shujuan
BigScience research project recently released a large-scale language model BLOOM. At first glance, it looks like a copy of OpenAI Another attempt at GPT-3.
But what sets BLOOM apart from other large-scale natural language models (LLMs) is its efforts in researching, developing, training, and publishing machine learning models.
In recent years, large technology companies have hidden large-scale natural language models (LLMs) like strict trade secrets, but the BigScience team has put transparency and openness at the center of BLOOM from the beginning of the project.
The result is a large language model, ready for research and learning, and available to everyone. The example of open source and open collaboration established by BLOOM will be very beneficial to future research in large-scale natural language models (LLM) and other areas of artificial intelligence. But there are still some challenges inherent to large language models that need to be addressed.
What is BLOOM?
BLOOM is the abbreviation of "BigScience Large-Scale Open Science Open Access Multilingual Model". From the data point of view, it is not much different from GPT-3 and OPT-175B. It is a very large Transformer model, with 176 billion parameters, trained using 1.6TB of data, including natural language and software source code.
Like GPT-3, it can perform many tasks through zero-shot or few-shot learning, including text generation, summarization, question answering, and programming.
But the importance of BLOOM lies in the organization and construction process behind it.
BigScience is a research project launched in 2021 by the Machine Learning Model Center "Hugging Face". According to its website, the project "aims to demonstrate an alternative way to create, learn, and share large language models and large research artifacts within the AI/NLP research community."
In this regard , BigScience draws inspiration from science-making initiatives such as CERN and the Large Hadron Collider (LHC), where open scientific collaborations promote large-scale artifacts useful to the entire research community of creation.
In the past year since May 2021, more than 1,000 researchers from 60 countries and more than 250 institutions have co-created BLOOM in BigScience.
Transparency, Openness, and Inclusion
While most major large-scale natural language models (LLMs) are trained on English text only, BLOOM’s training corpus includes 46 natural languages and 13 programming languages. This is useful in many regions where the primary language is not English.
BLOOM also breaks the actual reliance on the models of large technology training companies. One of the main problems with large natural language models (LLMs) is the high cost of training and tuning. This barrier makes large natural language models (LLMs) with 100 billion parameters the exclusive domain of large tech companies with deep pockets. In recent years, artificial intelligence labs have been attracted by large technology companies to obtain subsidized cloud computing resources and fund their research.
In contrast, the BigScience research team received a 3 million euro grant from the French National Center for Scientific Research to train BLOOM on the supercomputer Jean Zay. There is no agreement granting an exclusive license to the technology to a commercial company, nor is there a commitment to commercialize the model and turn it into a profitable product.
In addition, the BigScience team is completely transparent about the entire model training process. They publish datasets, meeting transcripts, discussions, and code, as well as logs and technical details of training models.
Researchers are studying the model’s data and metadata and publishing interesting findings.
For example, researcher David McClure tweeted on July 12, 2022, “I’ve been looking at the training dataset behind the really cool BLOOM model from Bigscience and Hugging Face. There are 10 million samples from the English corpus, about 1.25% of the total, encoded with 'all-distilroberta-v1', and then UMAP to 2d."
Of course, the trained model itself can be used in Hugging Face's platform, which relieves researchers from the pain of spending millions of dollars to train.
Facebook open sourced one of its large-scale natural language models (LLM) under some restrictions last month. However, the transparency brought by BLOOM is unprecedented and promises to set a new standard for the industry.
BLOOM training co-lead Teven LeScao said, “In contrast to the secrecy of industrial AI research labs, BLOOM demonstrates that the most powerful AI models can be developed responsibly and openly by the broader research community. way to train and publish.”
Challenges remain
While BigScience’s efforts to bring openness and transparency to artificial intelligence research and large language models are laudable, there are challenges inherent in the field Still nothing has changed.
Large-scale natural language model (LLM) research is moving towards larger and larger models, which will further increase training and running costs. BLOOM uses 384 Nvidia Tesla A100 GPUs (priced at about $32,000 each) for training. And larger models will require larger computing clusters. The BigScience team has announced that it will continue to create other open source large natural language models (LLMs), but it remains to be seen how the team will fund its increasingly expensive research. For example, OpenAI started as a non-profit organization and later became a for-profit organization that sells products and relies on funding from Microsoft.
Another issue that remains to be solved is the huge cost of running these models. The compressed BLOOM model is 227GB in size, and running it requires specialized hardware with hundreds of GB of memory. For comparison, GPT-3 requires a computing cluster equivalent to an Nvidia DGX 2, which costs about $400,000. Hugging Face plans to launch an API platform that will allow researchers to use the model for about $40 per hour, which is a significant cost.
The cost of running BLOOM will also impact the applied machine learning community, startups, and organizations looking to build products powered by large-scale natural language models (LLMs). Currently, the GPT-3 API provided by OpenAI is more suitable for product development. It will be interesting to see which direction BigScience and Hugging Face take to enable developers to build products based on their valuable research.
In this regard, there is an expectation that BigScience will have smaller versions of its models in future releases. Contrary to what is often portrayed in the media, Large Natural Language Models (LLMs) still adhere to the "no free lunch" principle. This means that when applying machine learning, a more compact model that is fine-tuned for a specific task is more effective than a very large model with average performance on many tasks. For example, Codex is a modified version of GPT-3 that provides great assistance with programming at a fraction of the size and cost of GPT-3. GitHub currently offers a Codex-based product, Copilot, for $10 per month.
It will be interesting to examine where academic and applied AI goes in the future as BLOOM hopes to establish a new culture.
Original title: BLOOM can set a new culture for AI research—but challenges remain, author :Ben Dickson
The above is the detailed content of BLOOM can create a new culture for AI research, but challenges remain. For more information, please follow other related articles on the PHP Chinese website!

Hot AI Tools

Undresser.AI Undress
AI-powered app for creating realistic nude photos

AI Clothes Remover
Online AI tool for removing clothes from photos.

Undress AI Tool
Undress images for free

Clothoff.io
AI clothes remover

Video Face Swap
Swap faces in any video effortlessly with our completely free AI face swap tool!

Hot Article

Hot Tools

Notepad++7.3.1
Easy-to-use and free code editor

SublimeText3 Chinese version
Chinese version, very easy to use

Zend Studio 13.0.1
Powerful PHP integrated development environment

Dreamweaver CS6
Visual web development tools

SublimeText3 Mac version
God-level code editing software (SublimeText3)

Hot Topics
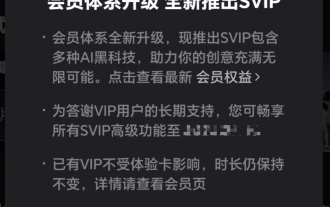
This site reported on June 27 that Jianying is a video editing software developed by FaceMeng Technology, a subsidiary of ByteDance. It relies on the Douyin platform and basically produces short video content for users of the platform. It is compatible with iOS, Android, and Windows. , MacOS and other operating systems. Jianying officially announced the upgrade of its membership system and launched a new SVIP, which includes a variety of AI black technologies, such as intelligent translation, intelligent highlighting, intelligent packaging, digital human synthesis, etc. In terms of price, the monthly fee for clipping SVIP is 79 yuan, the annual fee is 599 yuan (note on this site: equivalent to 49.9 yuan per month), the continuous monthly subscription is 59 yuan per month, and the continuous annual subscription is 499 yuan per year (equivalent to 41.6 yuan per month) . In addition, the cut official also stated that in order to improve the user experience, those who have subscribed to the original VIP
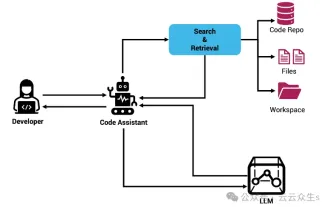
Improve developer productivity, efficiency, and accuracy by incorporating retrieval-enhanced generation and semantic memory into AI coding assistants. Translated from EnhancingAICodingAssistantswithContextUsingRAGandSEM-RAG, author JanakiramMSV. While basic AI programming assistants are naturally helpful, they often fail to provide the most relevant and correct code suggestions because they rely on a general understanding of the software language and the most common patterns of writing software. The code generated by these coding assistants is suitable for solving the problems they are responsible for solving, but often does not conform to the coding standards, conventions and styles of the individual teams. This often results in suggestions that need to be modified or refined in order for the code to be accepted into the application

To learn more about AIGC, please visit: 51CTOAI.x Community https://www.51cto.com/aigc/Translator|Jingyan Reviewer|Chonglou is different from the traditional question bank that can be seen everywhere on the Internet. These questions It requires thinking outside the box. Large Language Models (LLMs) are increasingly important in the fields of data science, generative artificial intelligence (GenAI), and artificial intelligence. These complex algorithms enhance human skills and drive efficiency and innovation in many industries, becoming the key for companies to remain competitive. LLM has a wide range of applications. It can be used in fields such as natural language processing, text generation, speech recognition and recommendation systems. By learning from large amounts of data, LLM is able to generate text
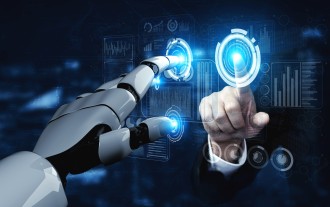
Large Language Models (LLMs) are trained on huge text databases, where they acquire large amounts of real-world knowledge. This knowledge is embedded into their parameters and can then be used when needed. The knowledge of these models is "reified" at the end of training. At the end of pre-training, the model actually stops learning. Align or fine-tune the model to learn how to leverage this knowledge and respond more naturally to user questions. But sometimes model knowledge is not enough, and although the model can access external content through RAG, it is considered beneficial to adapt the model to new domains through fine-tuning. This fine-tuning is performed using input from human annotators or other LLM creations, where the model encounters additional real-world knowledge and integrates it
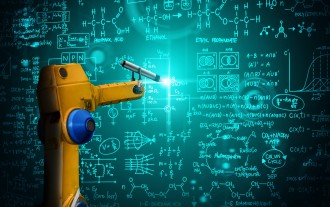
Machine learning is an important branch of artificial intelligence that gives computers the ability to learn from data and improve their capabilities without being explicitly programmed. Machine learning has a wide range of applications in various fields, from image recognition and natural language processing to recommendation systems and fraud detection, and it is changing the way we live. There are many different methods and theories in the field of machine learning, among which the five most influential methods are called the "Five Schools of Machine Learning". The five major schools are the symbolic school, the connectionist school, the evolutionary school, the Bayesian school and the analogy school. 1. Symbolism, also known as symbolism, emphasizes the use of symbols for logical reasoning and expression of knowledge. This school of thought believes that learning is a process of reverse deduction, through existing
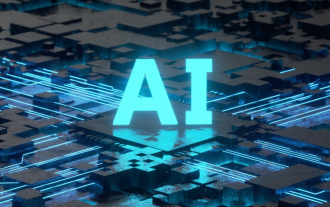
Editor |ScienceAI Question Answering (QA) data set plays a vital role in promoting natural language processing (NLP) research. High-quality QA data sets can not only be used to fine-tune models, but also effectively evaluate the capabilities of large language models (LLM), especially the ability to understand and reason about scientific knowledge. Although there are currently many scientific QA data sets covering medicine, chemistry, biology and other fields, these data sets still have some shortcomings. First, the data form is relatively simple, most of which are multiple-choice questions. They are easy to evaluate, but limit the model's answer selection range and cannot fully test the model's ability to answer scientific questions. In contrast, open-ended Q&A
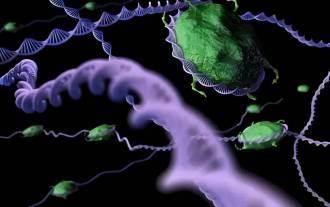
Editor | KX In the field of drug research and development, accurately and effectively predicting the binding affinity of proteins and ligands is crucial for drug screening and optimization. However, current studies do not take into account the important role of molecular surface information in protein-ligand interactions. Based on this, researchers from Xiamen University proposed a novel multi-modal feature extraction (MFE) framework, which for the first time combines information on protein surface, 3D structure and sequence, and uses a cross-attention mechanism to compare different modalities. feature alignment. Experimental results demonstrate that this method achieves state-of-the-art performance in predicting protein-ligand binding affinities. Furthermore, ablation studies demonstrate the effectiveness and necessity of protein surface information and multimodal feature alignment within this framework. Related research begins with "S
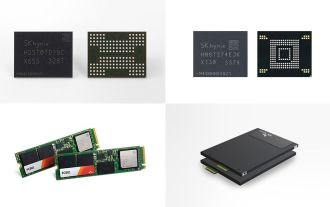
According to news from this site on August 1, SK Hynix released a blog post today (August 1), announcing that it will attend the Global Semiconductor Memory Summit FMS2024 to be held in Santa Clara, California, USA from August 6 to 8, showcasing many new technologies. generation product. Introduction to the Future Memory and Storage Summit (FutureMemoryandStorage), formerly the Flash Memory Summit (FlashMemorySummit) mainly for NAND suppliers, in the context of increasing attention to artificial intelligence technology, this year was renamed the Future Memory and Storage Summit (FutureMemoryandStorage) to invite DRAM and storage vendors and many more players. New product SK hynix launched last year
