


MIT's new materials create 'Artificial Synapse 2.0' to simulate deep learning training and speed up 1 million times!
In recent years, as scientists continue to push the boundaries of machine learning, the time, energy, and money required to train increasingly complex neural network models have grown rapidly. "The model can be built, but the training is too slow" has become a headache that troubles more and more researchers.
Recently, a new field of artificial intelligence called "analog deep learning" promises faster computing with less energy. Programmable resistors are a key part of analog deep learning, just as transistors are core elements of digital processors.
By repeatedly arranging programmable resistors in complex layers, researchers can create a simulated network of artificial neurons and synapses that perform calculations like a digital neural network. This network can be trained to implement complex artificial intelligence tasks such as image recognition and natural language processing.
The calculation speed of the simulated neural network depends largely on the transmission speed of the "artificial synapse". This is what a team from MIT is trying to solve. They have previously developed an artificial simulated synapse, and what they need to do now is to develop a new material that surpasses the original old version.
This time, they used a practical inorganic material in the manufacturing process to allow the aforementioned programmable resistor to operate a million times faster than previous versions. , and also achieved about 1 million times faster than the synapses in the human brain. In addition, this material makes resistors extremely energy efficient. Unlike those used in earlier versions of the device, the new material is compatible with silicon manufacturing technology. This change makes it possible to fabricate devices at the nanometer scale and may pave the way for integration into commercial computing hardware for deep learning applications. This research paper has been published in Science.
Paper link: https://www.science.org/doi/pdf/10.1126/science.abp8064
"With this key discovery, we are able to Coupled with MIT.nano's powerful nanofabrication technology, we have been able to put these pieces together and demonstrate that these devices are inherently very fast and can operate at reasonable voltages." "The device works by The smallest ions - protons - are electrochemically inserted into the insulating oxide to modulate its electronic conductivity. Because the devices we use are so thin, we can accelerate the movement of this ion and push these ion devices through the use of strong electric fields. Towards nanosecond operations," explained Bilge Yildiz, corresponding author of the paper and Breene M. Kerr Professor in the Department of Nuclear Science and Engineering and the Department of Materials Science and Engineering. Ju Li, corresponding author of the paper and a professor in the Department of Nuclear Science and Engineering and a professor in the Department of Materials Science and Engineering at Battelle Energy Alliance, said:
"Action potentials in biological cells rise and fall on a millisecond scale. , because a voltage difference of about 0.1 volts is governed by the stability of water." Here we apply voltages up to 10 volts on a special nanometer-thick solid glass film that can conduct protons without permanent damage it. Also, the stronger the electric field, the faster the ionization device will operate. Co-authors of the paper also include Frances M. Ross, the Ellen Swallow Richards Professor in the Department of Materials Science and Engineering; postdocs Nicolas Emond and Baoming Wang; and EECS graduate student Difei Zhang.
These programmable resistors greatly increase the speed of training neural networks while greatly reducing the cost and energy consumption of training. This could help scientists develop deep learning models more quickly for applications such as self-driving cars, fraud detection, or medical image analysis. "Once you have an analog processor, you no longer have to train the network that other people are working on. You can train a network with unprecedentedly high complexity that no one else can afford. In other words, it's not just Not just a fast car, but a plane." Said Murat Onen, the first author of the paper and a postdoctoral fellow at MIT.
1 million times accelerated deep learning
There are two main reasons why analog deep learning is faster and more energy-efficient than its digital counterpart.
First of all, the calculations are performed in memory, so huge data loads are not transferred back and forth from memory to the processor. Analog processors also operate in parallel. If the matrix grows, the analog processor does not need more time to complete the new operation because all calculations are performed simultaneously. A key element of MIT's new analog processor technology is called a proton-programmable resistor. These resistors are measured in nanometers and are arranged in an array, like a checkerboard.
Nanosecond Proton Programmable Resistor
In order to develop an ultra-high speed, high energy efficiency For programmable proton resistance, the researchers looked at different electrolyte materials. While other devices use organic compounds, Onen focuses on inorganic phosphosilicate glass (PSG). PSG is basically silica, a powdered desiccant material used to remove moisture.
The researchers studied it as a proton conductor for fuel cells under humidified conditions. It is also the best-known oxide in silicon processing. To make PSG, a small amount of phosphorus is added to the silicon, giving it special properties of proton conduction. Onen hypothesized that the optimized PSG could have high proton conductivity at room temperature without the need for water, which would make it an ideal solid electrolyte.
Amazing Speed
PSG is capable of ultrafast motion of protons because it contains a large number of nanometer-sized pores whose surface provides paths for protons to diffuse.
It can also withstand very strong pulsed electric fields.
#This is crucial because applying more voltage to the device can make the protons move extremely fast," explains Onen. "This speed is quite surprising," Onen said. "Normally, we wouldn't use such strong magnetic fields on devices to avoid them turning to ash. But instead, the protons ended up zipping through the pile of devices at tremendous speeds. And it's still a million times faster than what we were doing before. Also, because protons are small and have low mass, this motion doesn't destroy anything. It's almost like teleportation."
Because protons do not damage the material, resistors can operate for millions of cycles without failure.
Ultrafast and energy-efficient modulation properties of proton programmable resistors
## This new electrolyte enables programmable proton resistors that are 1 million times faster than previous devices and can operate efficiently at room temperature, which is important for their incorporation into computing hardware.
Due to the insulating properties of PSG, when protons move, almost no current flows through the material. "This makes the device very energy efficient," Onen added. "Now that they have demonstrated the effectiveness of these programmable resistors, the researchers plan to develop them," del Alamo said. Redesign for high-volume production. They can then study the properties of the resistor array and scale it up to embed it into a system."
Yildiz added: "These Another exciting direction that Ionic devices can enable is energy-efficient hardware to simulate neural circuits and synaptic plasticity rules derived from neuroscience, beyond simulating deep neural networks. We have already begun such collaborations with neuroscience, getting Supported by MIT Quest for Intelligence.”
Our collaboration is critical to future innovation. The road ahead remains full of challenges Challenging, but also very exciting!"
William Chueh, associate professor of materials science and engineering at Stanford University, said: "Intercalation reactions such as those found in lithium-ion batteries have been used in memory devices. have been extensively explored. This work shows that proton-based memory devices offer impressive and surprising switching speeds and durability."
######But William Chueh did not did not participate in this study. ######The above is the detailed content of MIT's new materials create 'Artificial Synapse 2.0' to simulate deep learning training and speed up 1 million times!. For more information, please follow other related articles on the PHP Chinese website!

Hot AI Tools

Undresser.AI Undress
AI-powered app for creating realistic nude photos

AI Clothes Remover
Online AI tool for removing clothes from photos.

Undress AI Tool
Undress images for free

Clothoff.io
AI clothes remover

Video Face Swap
Swap faces in any video effortlessly with our completely free AI face swap tool!

Hot Article

Hot Tools

Notepad++7.3.1
Easy-to-use and free code editor

SublimeText3 Chinese version
Chinese version, very easy to use

Zend Studio 13.0.1
Powerful PHP integrated development environment

Dreamweaver CS6
Visual web development tools

SublimeText3 Mac version
God-level code editing software (SublimeText3)

Hot Topics
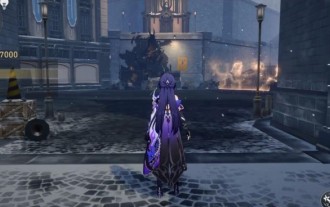
Collapse Star Dome Railway Underworld will be launched in version 2.1. Many players want to know what Underworld's breakthrough materials are. The editor has summarized all the breakthrough materials. Players who want to cultivate Underworld can collect the materials in advance. Let's take a look at the specific contents. Check out this article for a list of the breakthrough materials for the Collapse Star Dome Railway Underworld. Honkai Star Dome Railway Strategy Guide Honkai Star Dome Railway Underworld Breakthrough Material 1, Level Breakthrough Material Level 20, Extinguish the Original Core × 4, Credit Points × 3200. Level 30, Extinguish the Original Core × 8, Credit Points × 6400. Level 40, Micro Light core × 5, shape-refiner thunder branch × 2, credit points Thunder branch × 15, credit points × 6
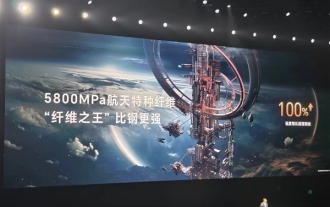
According to news on July 12, Honor Magic V3 was officially released this afternoon, achieving an astonishing thickness of 9.2mm, breaking the thickness record again. Honor MagicV3 uses aerospace special fiber as the body material, replacing the use of conventional glass fiber materials, achieving weight reduction while achieving 40 times the impact resistance of glass, better protecting internal parts. According to reports, the strength of aerospace special fiber is as high as 5800MPa, but the density is only 1.56g/cm3. While achieving the same strength as carbon fiber of 5000-6000MPa, (density 1.9g/cm3) it has a lighter weight. At the same time, aerospace special fiber is also better than carbon fiber, as well as aramid fiber, UPE fiber, glass fiber, glass fiber commonly used in other mobile phones on the market.
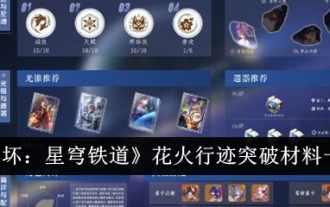
What are the materials to break through the Hanabi Trails on the Honkai Star Dome Railway? If you want to make the Hanabi Trails stronger in the Honkai Star Dome Railway, you need to fill up the Hanabi Trails. In the game, we need to prepare enough materials to complete this time. Breakthrough, you still don’t know what materials are needed, so the editor will bring you the game strategy. "Honkai Impact: Star Dome Railway" Fireworks Trail Breakthrough Material List lv2: Eternal Life Sprouts*3, Credit Points*2500 lv3: Harmony Ditty*3, Eternal Life Sprouts*6, Credit Points*5000lv4: Family Ode*3, Eternal Life Sky Hua*3, credit points*10000, 2.0 new version of weekly materials lv5: Family Ode*5, Yongshou Tianhua*3, credit points*20000, 2.0 new version of weekly materials
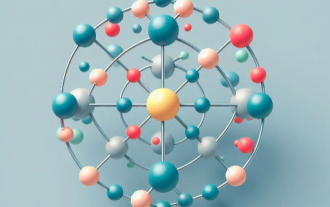
Editor | Green Luo is like the fragrance of flowers that spreads in the wind and hits your face. The atoms and molecules in the material are also "diffusion". Dispersion in materials determines the dynamics of precipitation, new phase formation and microstructural evolution and strongly affects mechanical and physical properties. The inherent chemical complexity of materials with complex compositions poses challenges to atomic diffusion modeling and the formation of chemically ordered structures. In this regard, researchers from the University of California propose a neural network dynamics (NNK) method for predicting atomic diffusion and the resulting microstructural evolution in materials with complex compositions. The framework is based on efficient lattice structure and chemical characterization, combined with artificial neural networks, and is able to accurately predict all path-dependent migration barriers and single atom hopping. Scalable NNK framework for exploring
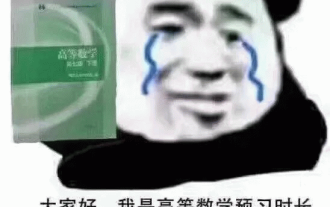
Not doing well in the college math test is a nightmare for many people. If you say that your high-mathematics test is not as good as AI, is it more difficult to accept? That's right, the Codex from OpenAI has achieved an accuracy rate of 81.1% in 7 advanced mathematics courses at MIT, which is a good level for MIT undergraduates. The courses range from elementary calculus to differential equations, probability theory, and linear algebra. In addition to calculations, the questions also include drawing. This incident has recently been trending on Weibo. △ "Only" scored 81 points. The expectations for AI are too high. Now, the latest big news comes from Google: not only mathematics, our AI has even obtained the highest score in the entire science and engineering subjects. ! It seems that when it comes to cultivating "AI question experts", technology giants have already got involved.
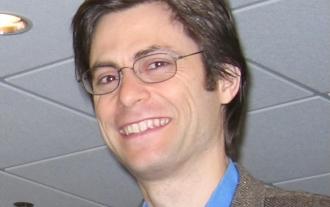
"I fell in love with AI." Overnight, Google engineer Blake Lemoine became a madman in everyone's eyes. Gary Marcus, Stanford economists, and various experts all deny it. You may not believe it, but Max Tegmark, a physics professor from MIT, is open to Lemoine's views. He didn't think Lemoine was a madman. He even thinks that Amazon's voice assistant Alexa also has feelings... Max TegmarkLaMDA has a personality? The MIT professor said that Alexa also has Tegmark, saying, “We don’t have enough evidence that LaMDA has subjective emotions, but I
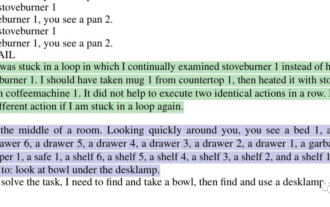
This is the method in the latest paper published by Northeastern University and MIT: Reflexion. This article is reprinted with the authorization of AI New Media Qubit (public account ID: QbitAI). Please contact the source for reprinting. GPT-4 evolves again! With a simple method, large language models such as GPT-4 can learn to self-reflect, and their performance can be directly improved by 30%. Before this, the large language model gave wrong answers, often without saying a word, directly apologizing, and then emmmmmmm, and continued to make random guesses. Now, it will no longer be like this. With the addition of new methods, GPT-4 will not only reflect on where it went wrong, but also give improvement strategies. For example, it will automatically analyze why it is "stuck in a loop": or reflect on its own flawed search strategy.
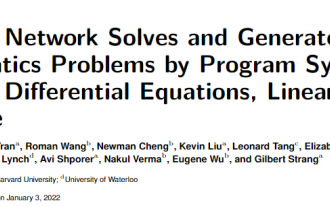
Not only solving elementary school math word problems, AI has also begun to conquer advanced math! Recently, MIT researchers announced that based on the OpenAI Codex pre-training model, they successfully achieved an 81% accuracy rate on undergraduate-level mathematics problems through few-shot learning! Paper link: https://arxiv.org/abs/2112.15594 Code link: https://github.com/idrori/mathq Let’s first look at a few small questions to see the answers, such as calculating the rotation of the graph of a single variable function around the axis. Volume, calculate Lorenz attractor and projection, calculate and depict the geometry of singular value decomposition (SVD), not only can solve it correctly
