


Merging two models with zero obstacles, linear connection of large ResNet models takes only seconds, inspiring new research on neural networks
Deep learning has been able to achieve such achievements thanks to its ability to solve large-scale non-convex optimization problems with relative ease. Although non-convex optimization is NP-hard, some simple algorithms, usually variants of stochastic gradient descent (SGD), have shown surprising effectiveness in actually fitting large neural networks.
In this article, several scholars from the University of Washington wrote "Git Re-Basin: Merging Models modulo Permutation Symmetries". They studied the SGD algorithm in high-dimensional non-convex optimization in deep learning. Unreasonable effectiveness on the issue. They were inspired by three questions:
1. Why does SGD perform well in the optimization of high-dimensional non-convex deep learning loss landscapes, while in other non-convex optimization settings, such as policy learning, The robustness of trajectory optimization and recommendation systems has significantly declined?
2. Where are the local minima? Why does the loss decrease smoothly and monotonically when linearly interpolating between initialization weights and final training weights?
3. Why do two independently trained models with different random initialization and data batching order achieve almost the same performance? Furthermore, why do their training loss curves look the same
Paper address: https://arxiv.org/pdf/2209.04836. pdf
This article believes that there is some invariance in model training, so that different trainings will show almost the same performance.
Why is this so? In 2019, Brea et al. noticed that hidden units in neural networks have arrangement symmetry. Simply put: we can swap any two units in the hidden layer of the network, and the network functionality will remain the same. Entezari et al. 2021 speculated that these permutation symmetries might allow us to linearly connect points in weight space without compromising losses.
Below we use an example from one of the authors of the paper to illustrate the main purpose of the article, so that everyone will have a clearer understanding.
Suppose you trained an A model and your friend trained a B model, the training data of the two models may be different. It doesn't matter, using the Git Re-Basin proposed in this article, you can merge the two models A B in the weight space without damaging the loss.
The authors of the paper stated that Git Re-Basin can be applied to any neural network (NN), and they demonstrated it for the first time A zero-barrier linear connection is possible between two independently trained (without pre-training) models (ResNets).
They found that merging ability is a property of SGD training, merging does not work at initialization, but a phase change occurs, so merging will become possible over time.
# They also found that model width is closely related to mergeability, that is, wider is better.
Also, not all architectures can be merged: VGG seems to be more difficult to merge than ResNets.
This merging method has other advantages, you can train the model on disjoint and biased data sets and then merge them together in the weight space. For example, you have some data in the US and some in the EU. For some reason the data cannot be mixed. You can train separate models first, then merge the weights, and finally generalize to the merged dataset.
Thus, trained models can be mixed without the need for pre-training or fine-tuning. The author expressed that he is interested in knowing the future development direction of linear mode connection and model repair, which may be applied to areas such as federated learning, distributed training, and deep learning optimization.
Finally, it is also mentioned that the weight matching algorithm in Chapter 3.2 only takes about 10 seconds to run, so a lot of time is saved. Chapter 3 of the paper also introduces three methods for matching model A and model B units. Friends who are not clear about the matching algorithm can check the original paper.
Netizen comments and author’s questions
This paper triggered a heated discussion on Twitter. Soumith Chintala, co-founder of PyTorch, said that if this research can be migrated to more The larger the setting, the better the direction it can take. Merging two models (including weights) can expand ML model development and may play a huge role in open source co-development of models.
Others believe that if permutation invariance can capture most equivalences so efficiently, it will provide inspiration for theoretical research on neural networks.
The first author of the paper, Dr. Samuel Ainsworth of the University of Washington, also answered some questions raised by netizens.
First someone asked, "Are there any hints in the paper about targeting unique basins in training? If there was a way to abstract the permutations, the training might be faster. Quick."
Ainsworth replied that he had not thought of this. He really hopes to be able to train faster somehow, but so far it has proven to be very difficult. The problem is that SGD is essentially a local search, so it's not that easy to exploit higher-order geometry. Maybe distributed training is the way to go.
Some people also ask whether it is applicable to RNN and Transformers? Ainsworth says it works in principle, but he hasn't experimented with it yet. Time will prove everything.
Finally someone asked, "This seems to be very important for distributed training to "come true"? Could it be that DDPM ( Denoising diffusion probability model) does not use the ResNet residual block?"
Ainsworth replied that although he himself is not very familiar with DDPM, he bluntly stated that it is used for distributed Training will be very exciting.
The above is the detailed content of Merging two models with zero obstacles, linear connection of large ResNet models takes only seconds, inspiring new research on neural networks. For more information, please follow other related articles on the PHP Chinese website!

Hot AI Tools

Undresser.AI Undress
AI-powered app for creating realistic nude photos

AI Clothes Remover
Online AI tool for removing clothes from photos.

Undress AI Tool
Undress images for free

Clothoff.io
AI clothes remover

Video Face Swap
Swap faces in any video effortlessly with our completely free AI face swap tool!

Hot Article

Hot Tools

Notepad++7.3.1
Easy-to-use and free code editor

SublimeText3 Chinese version
Chinese version, very easy to use

Zend Studio 13.0.1
Powerful PHP integrated development environment

Dreamweaver CS6
Visual web development tools

SublimeText3 Mac version
God-level code editing software (SublimeText3)

Hot Topics
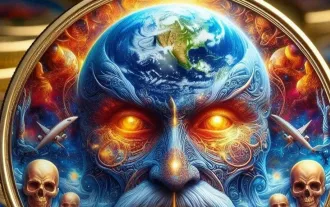
WorldCoin (WLD) stands out in the cryptocurrency market with its unique biometric verification and privacy protection mechanisms, attracting the attention of many investors. WLD has performed outstandingly among altcoins with its innovative technologies, especially in combination with OpenAI artificial intelligence technology. But how will the digital assets behave in the next few years? Let's predict the future price of WLD together. The 2025 WLD price forecast is expected to achieve significant growth in WLD in 2025. Market analysis shows that the average WLD price may reach $1.31, with a maximum of $1.36. However, in a bear market, the price may fall to around $0.55. This growth expectation is mainly due to WorldCoin2.
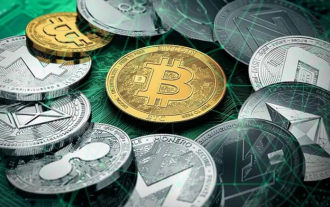
Factors of rising virtual currency prices include: 1. Increased market demand, 2. Decreased supply, 3. Stimulated positive news, 4. Optimistic market sentiment, 5. Macroeconomic environment; Decline factors include: 1. Decreased market demand, 2. Increased supply, 3. Strike of negative news, 4. Pessimistic market sentiment, 5. Macroeconomic environment.
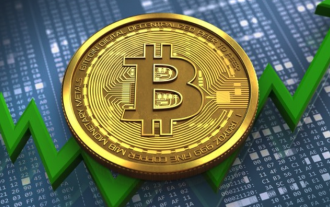
Exchanges that support cross-chain transactions: 1. Binance, 2. Uniswap, 3. SushiSwap, 4. Curve Finance, 5. Thorchain, 6. 1inch Exchange, 7. DLN Trade, these platforms support multi-chain asset transactions through various technologies.
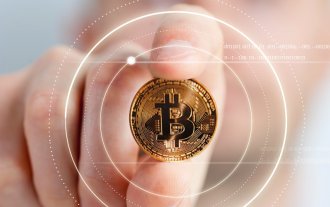
The steps to draw a Bitcoin structure analysis chart include: 1. Determine the purpose and audience of the drawing, 2. Select the right tool, 3. Design the framework and fill in the core components, 4. Refer to the existing template. Complete steps ensure that the chart is accurate and easy to understand.
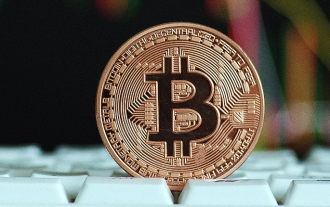
Suggestions for choosing a cryptocurrency exchange: 1. For liquidity requirements, priority is Binance, Gate.io or OKX, because of its order depth and strong volatility resistance. 2. Compliance and security, Coinbase, Kraken and Gemini have strict regulatory endorsement. 3. Innovative functions, KuCoin's soft staking and Bybit's derivative design are suitable for advanced users.
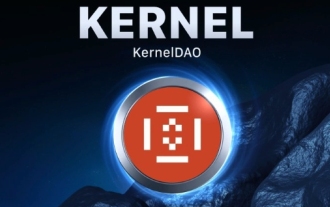
In the bustling world of cryptocurrencies, new opportunities always emerge. At present, KernelDAO (KERNEL) airdrop activity is attracting much attention and attracting the attention of many investors. So, what is the origin of this project? What benefits can BNB Holder get from it? Don't worry, the following will reveal it one by one for you.

Aavenomics is a proposal to modify the AAVE protocol token and introduce token repos, which has implemented a quorum for AAVEDAO. Marc Zeller, founder of the AAVE Project Chain (ACI), announced this on X, noting that it marks a new era for the agreement. Marc Zeller, founder of the AAVE Chain Initiative (ACI), announced on X that the Aavenomics proposal includes modifying the AAVE protocol token and introducing token repos, has achieved a quorum for AAVEDAO. According to Zeller, this marks a new era for the agreement. AaveDao members voted overwhelmingly to support the proposal, which was 100 per week on Wednesday
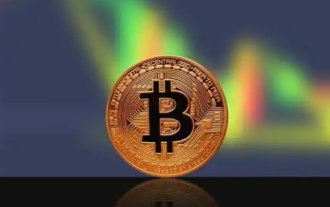
Cryptocurrency data platforms suitable for beginners include CoinMarketCap and non-small trumpet. 1. CoinMarketCap provides global real-time price, market value, and trading volume rankings for novice and basic analysis needs. 2. The non-small quotation provides a Chinese-friendly interface, suitable for Chinese users to quickly screen low-risk potential projects.
