


General artificial intelligence, artificial intelligence perception, and large language models
Perhaps you haven’t noticed that the recent performance of artificial intelligence systems has become more and more surprising.
For example, OpenAI’s new model DALL-E 2 can generate engaging original images based on simple text prompts. Models like DALL-E make it harder to deny the notion that artificial intelligence can be creative. Consider, for example, DALL-E's imaginative take on "a hip-hop cow wearing a denim jacket recording a hit single in the studio." Or for a more abstract example, check out DALL-E's explanation of the old Peter Thiel line "We want flying cars, not 140 characters."
Meanwhile, DeepMind recently announced a new technology called Gato's new model, which can single-handedly perform hundreds of different tasks, from playing video games to having conversations to stacking real-world blocks with a robotic arm. Almost all previous AI models were able to do one thing and one thing only—for example, play chess. As such, Gato represents an important step toward broader, more flexible machine intelligence.
And today’s large language models (LLMs)—from OpenAI’s GPT-3 to Google’s PaLM to Facebook’s OPT—have a dizzying array of language capabilities. They can have nuanced and in-depth conversations about almost any topic. They can generate impressive, original content themselves, from business memos to poetry. To give just one recent example, GPT-3 recently authored a well-written academic paper about itself and is currently undergoing peer review for publication in a prestigious scientific journal.
These advances have inspired bold speculations and heated discussions in the artificial intelligence community about the direction of technological development.
Some credible AI researchers believe we are now very close to “artificial general intelligence” (AGI), an oft-discussed benchmark that refers to powerful, flexible artificial intelligence that can operate on any Outperforming humans in cognitive tasks. Last month, a Google engineer named Blake Lemoine made headlines by dramatically claiming that Google's large-scale language model, LaMDA, is sentient.
Resistance to such claims has been equally strong, with many AI commentators dismissing the possibility out of hand.
So, what are we to make of all the amazing recent advances in artificial intelligence? How should we think about concepts like artificial intelligence and artificial intelligence perception?
Public discourse on these topics needs to be reframed in several important ways. Over-excited enthusiasts who believe that superintelligent AI is just around the corner, and dismissive skeptics who believe that recent developments in AI are just hype, are both off the mark in their thinking about some fundamental aspects of modern AI.
AI is an incoherent concept
A basic principle about artificial intelligence that is often overlooked is that artificial intelligence is fundamentally different from human intelligence.
It is a mistake to compare artificial intelligence to human intelligence too directly. Today’s artificial intelligence is more than just a “less evolved” form of human intelligence. Tomorrow’s super-advanced AI won’t just be a more powerful version of human intelligence, either.
Many different intelligence modes and dimensions are possible. Artificial intelligence is best thought of not as an imperfect imitation of human intelligence, but as a unique, alien form of intelligence whose contours and capabilities differ from ours in fundamental ways.
To make this more concrete, briefly consider the state of artificial intelligence today. Today’s artificial intelligence far exceeds human capabilities in some areas – while falling far behind in others.
For example: For half a century, the "protein folding problem" has been a major challenge in the field of biology. In short, the protein folding problem requires predicting the three-dimensional shape of a protein based on its one-dimensional amino acid sequence. Decades and generations of the world's brightest minds have worked together to fail to solve this challenge. One reviewer in 2007 described it as "one of the most important yet unsolved problems in modern science."
At the end of 2020, an AI model from DeepMind called AlphaFold provided a solution to the protein folding problem. As John Moult, who has been engaged in protein research for a long time, said, "This is the first time in history that serious scientific problems have been solved by AI."
Solving the mystery of protein folding requires spatial understanding and high-dimensional reasoning forms, and this It is simply beyond the grasp of human thinking. But it’s not beyond the grasp of modern machine learning systems.
Meanwhile, any healthy human child possesses “embodied intelligence” that far exceeds that of the world’s most sophisticated artificial intelligence.
From a young age, humans can effortlessly do things like play catch, walk across unfamiliar terrain, or open the kitchen refrigerator for a snack. It turns out that these physical abilities are difficult for artificial intelligence to master.
This is encapsulated in the "Moravec Paradox". As AI researcher Hans Moravec said in the 1980s: “It is relatively easy to get a computer to perform at an adult level on an intelligence test or to play chess, but it is difficult or impossible to get a computer to perform at the level of a one-year-old child. .Perception and mobility."
Moravec's explanation for this unintuitive fact is evolutionary: "Encoded over a billion years in the large, highly evolved sensory and motor parts of the human brain Experience about the nature of the world and how to live in it. [On the other hand,] the thoughtful process we call higher reasoning is, I believe, the thinnest layer of the human mind, and it works only because It is underpinned by this older, more powerful, albeit often unconscious, sensorimotor knowledge. We are all outstanding Olympians in the realms of perception and movement, so good that we make the difficult look easy.”
To this day, robots still struggle with basic physical abilities. Just a few weeks ago, a team of DeepMind researchers wrote in a new paper: "Current AI systems' understanding of 'intuitive physics' pales in comparison to that of very young children."
What is the result of all this?
There is no such thing as general artificial intelligence.
AGI is neither possible nor possible. Rather, it is incoherent as a concept.
Intelligence is not a single, well-defined, generalizable ability, or even a specific set of abilities. At the highest level, intelligent behavior is simply an agent acquiring and using knowledge about its environment to pursue its goals. Because there are a large (theoretically infinite) number of different types of agents, environments, and goals, intelligence can manifest itself in countless different ways.
AI guru Yann LeCun summed it up well: "There is no such thing as general artificial intelligence...even humans are specialized."
Convert "general" or "true" artificial intelligence Defining AI as something that can do what humans can do (but better)—thinking human intelligence is general intelligence—is short-sighted and human-centered. If we regard human intelligence as the ultimate anchor and standard for the development of artificial intelligence, we will miss all the powerful, profound, unexpected, socially beneficial, and completely non-human capabilities that machine intelligence may have.
Imagine an AI that had an atomic-level understanding of the composition of Earth’s atmosphere and could dynamically predict with extremely high accuracy how the entire system would evolve over time. Imagine if it could be designed to engineer a precise, safe geoengineering intervention in which we deposit certain amounts of certain compounds in certain places in the atmosphere, thus counteracting the greenhouse effect caused by humanity's continued carbon emissions, mitigating global change. Effects of warming on the Earth's surface.
Imagine an artificial intelligence that can understand every biological and chemical mechanism in the human body down to the molecular level. Imagine if it could thus prescribe a diet tailored to optimize each person's health, could accurately diagnose the root cause of any disease, could generate new personalized therapies (even if they didn't exist yet) to treat any serious disease .
Imagine an AI that could invent a protocol to fuse atomic nuclei in a way that safely produces more energy than it consumes, unlocking nuclear fusion as a cheap, sustainable, infinitely abundant of human energy.
All these scenarios are still fantasies today and are out of reach for today’s artificial intelligence. The point is that the true potential of AI lies in the path that leads to the development of new forms of intelligence that are completely unlike anything humans can do. If AI can achieve such a goal, who cares if it is "universal" in the sense of matching human capabilities overall?
Positioning ourselves as “general artificial intelligence” limits and diminishes the technology’s potential. And—because human intelligence is not general intelligence, which does not exist—it is conceptually incoherent in the first place.
What is it like to be an artificial intelligence?
This brings us to a related topic in the big picture of artificial intelligence, which is currently receiving a lot of public attention: the question of whether artificial intelligence is, or ever will be, sentient.
Google engineer Blake Lemoine sparked a wave of controversy and commentary last month when he publicly asserted that one of Google's large language models had become aware. (Before forming any definite opinions, it's worth reading the full transcript of the discussion between Lemoine and AI for yourself.)
Most people — especially AI experts — think Lemoine's claims are wrong and inaccurate. reasonable.
Google said in its official response: "Our team has reviewed Black's concerns and informed him that the evidence does not support his claims." Stanford University professor Erik Brynjolfsson believes that sensory artificial intelligence may still be 50 years away time. Gary Marcus chimed in, calling Lemoine's claims "nonsense," concluding that "there's nothing to see here."
The problem with this entire discussion—including the experts’ dismissal of it—is that the existence or absence of perception is, by definition, unprovable, unfalsifiable, and unknowable.
When we talk about perception, we are referring to the agent’s subjective inner experience, not to any external intellectual manifestation. No one—not Blake Lemoine, not Erik Brynjolfsson, not Gary Marcus—can be entirely sure what highly complex artificial neural networks do or do not experience internally.
In 1974, the philosopher Thomas Nagel published an article titled "What is it like to be a bat?" "Article. One of the most influential philosophical papers of the 20th century, this article boiled down the notoriously elusive concept of consciousness into a simple, intuitive definition: An agent is conscious if there is something willing to be that agent . For example, being my next door neighbor or even his dog is something; but being his mailbox is nothing like it.
A key message of the paper is that it is impossible to know exactly what it is like to be another organism or species in a meaningful way. The more unlike us another organism or species is, the more inaccessible its internal experience will be.
Nagel used bats as an example to illustrate this point. He chose bats because, as mammals, they are highly complex creatures, but their experience of life is very different from ours: they fly, they use sonar as their primary means of perceiving the world, and so on.
As Nagel puts it (it is worth quoting several paragraphs from the paper in full):
"Our own experience provides the basic material for our imagination, and therefore the scope of imagination is limited .Imagine a person with webbed arms that allow him to fly around at dusk and dawn and bugs in his mouth, which doesn't help; a person with very poor eyesight who perceives the world around him through a system of reflected high-frequency sound signals ;The guy hangs upside down in the attic all day long.
"As far as I can imagine (which isn't very far off), it only tells me what it would be like to act like a bat. But that's not the problem. I wonder what it feels like for a bat to be a bat. However, if I tried to imagine this, I would be limited to the resources of my own mind, which are insufficient for the task. I cannot achieve it by imagining additions to my present experience, or by imagining fragments gradually subtracted from it, or by imagining some combination of additions, subtractions, and modifications. ”
Artificial neural networks are more alien and inaccessible to us humans than bats, which are at least mammals and carbon-based life forms.
Likewise, too many commenters are The fundamental mistake made on this topic (often without even thinking about it) is to assume that we can simply map our expectations of human perception or intelligence to artificial intelligence. way to determine or even think about the intrinsic experience of AI. We simply cannot be sure.
So how can we approach the topic of AI perception in a productive way?
We can start with Turing ( Alan Turing was inspired by the Turing Test, first proposed in 1950. Often criticized or misunderstood, and certainly imperfect, the Turing Test has stood the test of time as a reference point in the field of AI because it captures Certain fundamental insights into the nature of machine intelligence.
The Turing Test acknowledges and accepts the reality that we will never have direct access to the internal experience of an artificial intelligence. Its entire premise is that if we want to measure the intelligence of an artificial intelligence, Our only option is to observe its behavior and draw appropriate inferences. (To be clear, Turing was concerned with assessing a machine's ability to think, not necessarily its ability to feel; however, for our purposes In other words, what is relevant is the fundamentals.)
Douglas Hofstadter articulated this idea particularly eloquently: "How do you know that when I'm talking to you, anything similar to what you're talking about is going on inside me? 'Thinking' thing? The Turing test is an amazing probe—like a particle accelerator in physics. Just like in physics, when you want to understand what's going on at the atomic or subatomic level, since you can't see it directly, you scatter accelerated particles away from relevant targets and observe their behavior. From this you can infer the internal properties of the target. The Turing Test extends this idea to the mind. It treats ideas as "objects" that are not directly visible but whose structure can be inferred more abstractly. By 'distracting' the problem from the target's mind, you can understand its inner workings, just like in physics. ”
To make any progress in discussions about artificial intelligence perception, we must orient ourselves toward observable representations as proxies for internal experience; otherwise, we will end up in a loose, empty, dead-end world. Going in circles in the debate.
Erik Brynjolfsson is convinced that today’s AI is not sentient. However, his comments indicate that he believes AI will eventually be sentient. When he encounters a truly sentient AI , how would he know? What would he be looking for?
You Are What You Do
In debates about AI, skeptics often describe the technology in simplified terms that downplay its capabilities.
As one AI researcher put it in response to the Blake Lemoine news: "The hope of gaining awareness, understanding, or common sense from symbolic and data processing using higher-dimensional parametric functions is mysterious." In a recent blog post, Gary Marcus argued that today’s AI models aren’t even “telepathically intelligent” because “all they do is match patterns and pull data from massive statistical databases.” He argued that Google’s large The language model LaMDA is just a "spreadsheet of words".
This line of reasoning is misleading and trivial. After all, if we so choose, we can build human intelligence in a similarly simplified way: our brains are "just" a large collection of neurons interconnected in specific ways, "just" a collection of basic chemical reactions within our skulls.
But this misses the point. The power and magic of human intelligence lies not in specific mechanisms but in the incredible ability to emerge in some way. Simple basic functions can produce profoundly intellectual systems.
Ultimately, we must judge artificial intelligence based on its capabilities.
If we compare the state of artificial intelligence five years ago to the state of technology today, there is no doubt that its capabilities and depth have increased significantly (and are still accelerating) due to breakthroughs in areas such as self-supervised learning. Ways to scale, transformer and reinforcement learning.
Artificial intelligence is not like human intelligence. When and if an AI becomes sentient—when and if it ever becomes, in Nagel's formulation, "like something"—it will be incomparable to what it is like to be human. Artificial intelligence is its own unique, unfamiliar, fascinating, and rapidly evolving form of cognition.
What matters is what artificial intelligence can achieve. Breakthroughs in fundamental science like AlphaFold, addressing species-level challenges like climate change, promoting human health and longevity, and deepening our understanding of how the universe works—these results are a true test of the power and complexity of AI.
The above is the detailed content of General artificial intelligence, artificial intelligence perception, and large language models. For more information, please follow other related articles on the PHP Chinese website!

Hot AI Tools

Undresser.AI Undress
AI-powered app for creating realistic nude photos

AI Clothes Remover
Online AI tool for removing clothes from photos.

Undress AI Tool
Undress images for free

Clothoff.io
AI clothes remover

Video Face Swap
Swap faces in any video effortlessly with our completely free AI face swap tool!

Hot Article

Hot Tools

Notepad++7.3.1
Easy-to-use and free code editor

SublimeText3 Chinese version
Chinese version, very easy to use

Zend Studio 13.0.1
Powerful PHP integrated development environment

Dreamweaver CS6
Visual web development tools

SublimeText3 Mac version
God-level code editing software (SublimeText3)

Hot Topics
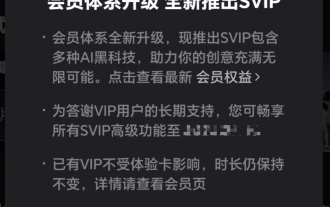
This site reported on June 27 that Jianying is a video editing software developed by FaceMeng Technology, a subsidiary of ByteDance. It relies on the Douyin platform and basically produces short video content for users of the platform. It is compatible with iOS, Android, and Windows. , MacOS and other operating systems. Jianying officially announced the upgrade of its membership system and launched a new SVIP, which includes a variety of AI black technologies, such as intelligent translation, intelligent highlighting, intelligent packaging, digital human synthesis, etc. In terms of price, the monthly fee for clipping SVIP is 79 yuan, the annual fee is 599 yuan (note on this site: equivalent to 49.9 yuan per month), the continuous monthly subscription is 59 yuan per month, and the continuous annual subscription is 499 yuan per year (equivalent to 41.6 yuan per month) . In addition, the cut official also stated that in order to improve the user experience, those who have subscribed to the original VIP
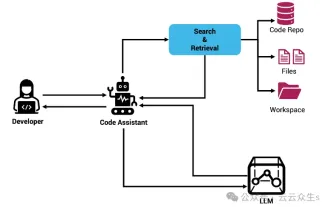
Improve developer productivity, efficiency, and accuracy by incorporating retrieval-enhanced generation and semantic memory into AI coding assistants. Translated from EnhancingAICodingAssistantswithContextUsingRAGandSEM-RAG, author JanakiramMSV. While basic AI programming assistants are naturally helpful, they often fail to provide the most relevant and correct code suggestions because they rely on a general understanding of the software language and the most common patterns of writing software. The code generated by these coding assistants is suitable for solving the problems they are responsible for solving, but often does not conform to the coding standards, conventions and styles of the individual teams. This often results in suggestions that need to be modified or refined in order for the code to be accepted into the application

To learn more about AIGC, please visit: 51CTOAI.x Community https://www.51cto.com/aigc/Translator|Jingyan Reviewer|Chonglou is different from the traditional question bank that can be seen everywhere on the Internet. These questions It requires thinking outside the box. Large Language Models (LLMs) are increasingly important in the fields of data science, generative artificial intelligence (GenAI), and artificial intelligence. These complex algorithms enhance human skills and drive efficiency and innovation in many industries, becoming the key for companies to remain competitive. LLM has a wide range of applications. It can be used in fields such as natural language processing, text generation, speech recognition and recommendation systems. By learning from large amounts of data, LLM is able to generate text
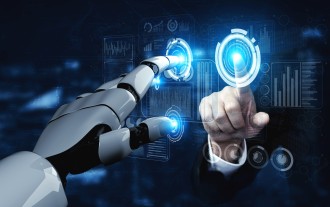
Large Language Models (LLMs) are trained on huge text databases, where they acquire large amounts of real-world knowledge. This knowledge is embedded into their parameters and can then be used when needed. The knowledge of these models is "reified" at the end of training. At the end of pre-training, the model actually stops learning. Align or fine-tune the model to learn how to leverage this knowledge and respond more naturally to user questions. But sometimes model knowledge is not enough, and although the model can access external content through RAG, it is considered beneficial to adapt the model to new domains through fine-tuning. This fine-tuning is performed using input from human annotators or other LLM creations, where the model encounters additional real-world knowledge and integrates it
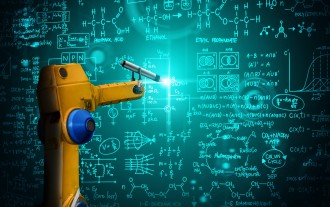
Machine learning is an important branch of artificial intelligence that gives computers the ability to learn from data and improve their capabilities without being explicitly programmed. Machine learning has a wide range of applications in various fields, from image recognition and natural language processing to recommendation systems and fraud detection, and it is changing the way we live. There are many different methods and theories in the field of machine learning, among which the five most influential methods are called the "Five Schools of Machine Learning". The five major schools are the symbolic school, the connectionist school, the evolutionary school, the Bayesian school and the analogy school. 1. Symbolism, also known as symbolism, emphasizes the use of symbols for logical reasoning and expression of knowledge. This school of thought believes that learning is a process of reverse deduction, through existing
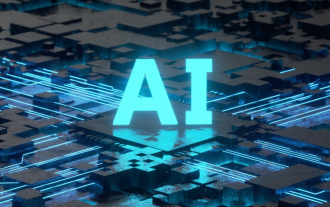
Editor |ScienceAI Question Answering (QA) data set plays a vital role in promoting natural language processing (NLP) research. High-quality QA data sets can not only be used to fine-tune models, but also effectively evaluate the capabilities of large language models (LLM), especially the ability to understand and reason about scientific knowledge. Although there are currently many scientific QA data sets covering medicine, chemistry, biology and other fields, these data sets still have some shortcomings. First, the data form is relatively simple, most of which are multiple-choice questions. They are easy to evaluate, but limit the model's answer selection range and cannot fully test the model's ability to answer scientific questions. In contrast, open-ended Q&A
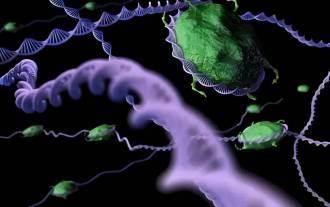
Editor | KX In the field of drug research and development, accurately and effectively predicting the binding affinity of proteins and ligands is crucial for drug screening and optimization. However, current studies do not take into account the important role of molecular surface information in protein-ligand interactions. Based on this, researchers from Xiamen University proposed a novel multi-modal feature extraction (MFE) framework, which for the first time combines information on protein surface, 3D structure and sequence, and uses a cross-attention mechanism to compare different modalities. feature alignment. Experimental results demonstrate that this method achieves state-of-the-art performance in predicting protein-ligand binding affinities. Furthermore, ablation studies demonstrate the effectiveness and necessity of protein surface information and multimodal feature alignment within this framework. Related research begins with "S
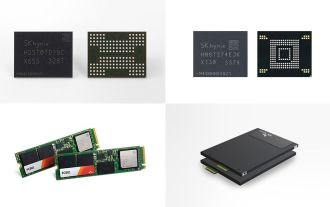
According to news from this site on August 1, SK Hynix released a blog post today (August 1), announcing that it will attend the Global Semiconductor Memory Summit FMS2024 to be held in Santa Clara, California, USA from August 6 to 8, showcasing many new technologies. generation product. Introduction to the Future Memory and Storage Summit (FutureMemoryandStorage), formerly the Flash Memory Summit (FlashMemorySummit) mainly for NAND suppliers, in the context of increasing attention to artificial intelligence technology, this year was renamed the Future Memory and Storage Summit (FutureMemoryandStorage) to invite DRAM and storage vendors and many more players. New product SK hynix launched last year
