


LeCun's paper was accused of 'washing'? The father of LSTM wrote angrily: Copy my work and mark it as original.
Turing Award winner Yann Lecun, as one of the three giants in the AI field, the papers he published are naturally studied as the "Bible".
However, recently, someone suddenly jumped out and criticized LeCun for "sitting on the ground": "It's nothing more than rephrasing my core point of view."
Could it be...
Schmidhuber stated in this long article that he hopes readers can study the original papers and judge the scientific content of these comments for themselves, and also hopes that his work will be recognized and recognized.
LeCun stated at the beginning of the paper that many of the ideas described in this paper were (almost all) presented by many authors in different contexts and in different forms. Schmidhuber countered that unfortunately, most of the paper The content is "similar" to our papers since 1990, and there is no citation mark.
Let’s first take a look at the evidence (part) of his attack on LeCun this time.
Evidence 1:
LeCun: Today’s artificial intelligence research must solve three main challenges: (1) How can machines learn to represent the world? , learn to predict, and learn to act primarily through observation (2) How machines can reason and plan in a manner compatible with gradient-based learning (3) How machines can do so in a hierarchical manner, at multiple levels of abstraction and at multiple times Learning representations for perception (3a) and action planning (3b) at scale
Schmidhuber: These issues were addressed in detail in a series of papers published in 1990, 1991, 1997 and 2015.
In 1990, the first work on gradient-based artificial neural networks (NN) for long-term planning and reinforcement learning (RL) and exploration through artificial curiosity was published.
It describes the combination of two recurrent neural networks (RNN, the most powerful NNN), called the controller and the world model.
Among them, the world model learns to predict the consequences of the controller's actions. The controller can use the world model to plan several time steps in advance and select the action sequence that maximizes the predicted reward.
Regarding the answer to hierarchical perception based on neural networks (3a), this question is at least partially inspired by my 1991 publication of "The First Deep Learning Machine—Neural Sequence Analyzer" Blocker" solution.
It uses unsupervised learning and predictive coding in deep hierarchies of recurrent neural networks (RNN) to find " Internal representation of long data sequences".
The answer to hierarchical action planning (3b) based on neural networks has been at least partially solved in 1990 by my paper on hierarchical reinforcement learning (HRL) solved this problem.
Evidence 2:
LeCun: Since both sub-modules of the cost module are differentiable , so the energy gradient can be back-propagated through other modules, especially the world module, performance module and perception module.
Schmidhuber: This is exactly what I published in 1990, citing the "System Identification with Feedforward Neural Networks" paper published in 1980.
In 2000, my former postdoc Marcus Hutter even published a theoretically optimal, general, non-differentiable method for learning world models and controllers. (See also the mathematically optimal self-referential AGI called a Gödel machine)
Evidence 3:
LeCun: Short-Term Memory The module architecture may be similar to a key-value memory network.
Schmidhuber: However, he failed to mention that I published the first such "key-value memory network" in 1991, when I described sequence processing "Fast Weight Controllers" or Fast Weight Programmers (FWPs) . FWP has a slow neural network that learns through backpropagation to quickly modify the fast weights of another neural network.
Evidence 4:
LeCun: The main originality of this paper The contribution lies in: (I) a holistic cognitive architecture in which all modules are differentiable and many of them trainable. (II) H-JEPA: Models that predict non-generative hierarchical architectures of the world that learn representations at multiple levels of abstraction and multiple time scales. (III) A series of non-contrastive self-supervised learning paradigms that produce representations that are simultaneously informative and predictable. (IV) Use H-JEPA as the basis for a predictive world model for hierarchical planning under uncertainty.
In this regard, Schmidhuber also proofread the four modules listed by LeCun one by one, and gave points that overlap with his paper.
At the end of the article, he stated that the focus of this article was not to attack the published papers or the ideas reflected by their authors. The key point is that these ideas are not as "original" as written in LeCun's paper.
He said that many of these ideas were put forward with the efforts of me and my colleagues. His "Main original contribution" that LeCun is now proposing is actually inseparable from my decades of research contributions. I Readers are expected to judge the validity of my comments for themselves.
From the father of LSTM to...
In fact, this is not the first time that this uncle has claimed that others have plagiarized his results.
As early as September last year, he posted on his blog that the most cited neural network paper results are based on the work completed in my laboratory:
" Needless to say, LSTM, and other pioneering work that is famous today such as ResNet, AlexNet, GAN, and Transformer are all related to my work. The first version of some work was done by me, but now these people are not The emphasis on martial ethics and irregular citations have caused problems with the current attribution of these results." Although the uncle is very angry, I have to say that Jürgen Schmidhuber has indeed been a bit unhappy over the years. They are both senior figures in the field of AI and have made many groundbreaking achievements, but the reputation and recognition they have received always seem to be far behind expectations.
Especially in 2018, when the three giants of deep learning: Yoshua Bengio, Geoffrey Hinton, and Yann LeCun won the Turing Award, many netizens questioned why the Turing Award was not awarded to LSTM. Father Jürgen Schmidhuber? He is also a master in the field of deep learning.
Back in 2015, three great minds, Bengio, Hinton, and LeCun, jointly posted a review on Nature, and the title was directly called "Deep Learning".
The article starts from traditional machine learning technology, summarizes the main architecture and methods of modern machine learning, describes the backpropagation algorithm for training multi-layer network architecture, and the birth of convolutional neural network, distributed Representation and language processing, as well as recurrent neural networks and their applications, among others.
Less than a month later, Schmidhuber posted a criticism on his blog.
Schmidhuber said that this article made him very unhappy because the entire article quoted the three authors’ own research results many times, while other pioneers had earlier views on deep learning. Contribution is not mentioned at all.
He believes that the "Three Deep Learning Giants" who won the Turing Award have become thieves who are greedy for others' credit and think they are self-interested. They use their status in the world to flatter each other and suppress their senior academics.
In 2016, Jürgen Schmidhuber had a head-to-head confrontation with "the father of GAN" Ian Goodfellow in the Tutorial of the NIPS conference.
At that time, when Goodfellow was talking about comparing GAN with other models, Schmidhuber stood up and interrupted with questions.
Schmidhuber’s question was very long, lasting about two minutes. The main content was to emphasize that he had proposed PM in 1992, and then talked about a lot of it. Principle, implementation process, etc., the final picture shows: Can you tell me if there are any similarities between your GAN and my PM?
Goodfellow did not show any weakness: We have communicated the issue you mentioned many times in emails before, and I have responded to you publicly a long time ago. I don’t want to waste the audience’s patience on this occasion.
Wait, wait...
Perhaps these "honey operations" of Schmidhuber can be explained by an email from LeCun:
"Jürgen said to everyone He is too obsessed with recognition and always says that he has not received many things that he deserves. Almost habitually, he always stands up at the end of every speech given by others and says that he is responsible for the results just presented. Generally speaking, , this behavior is unreasonable."
The above is the detailed content of LeCun's paper was accused of 'washing'? The father of LSTM wrote angrily: Copy my work and mark it as original.. For more information, please follow other related articles on the PHP Chinese website!

Hot AI Tools

Undresser.AI Undress
AI-powered app for creating realistic nude photos

AI Clothes Remover
Online AI tool for removing clothes from photos.

Undress AI Tool
Undress images for free

Clothoff.io
AI clothes remover

Video Face Swap
Swap faces in any video effortlessly with our completely free AI face swap tool!

Hot Article

Hot Tools

Notepad++7.3.1
Easy-to-use and free code editor

SublimeText3 Chinese version
Chinese version, very easy to use

Zend Studio 13.0.1
Powerful PHP integrated development environment

Dreamweaver CS6
Visual web development tools

SublimeText3 Mac version
God-level code editing software (SublimeText3)

Hot Topics










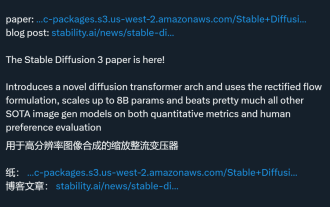
StableDiffusion3’s paper is finally here! This model was released two weeks ago and uses the same DiT (DiffusionTransformer) architecture as Sora. It caused quite a stir once it was released. Compared with the previous version, the quality of the images generated by StableDiffusion3 has been significantly improved. It now supports multi-theme prompts, and the text writing effect has also been improved, and garbled characters no longer appear. StabilityAI pointed out that StableDiffusion3 is a series of models with parameter sizes ranging from 800M to 8B. This parameter range means that the model can be run directly on many portable devices, significantly reducing the use of AI
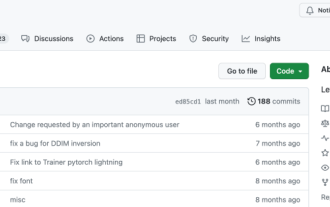
ICCV2023, the top computer vision conference held in Paris, France, has just ended! This year's best paper award is simply a "fight between gods". For example, the two papers that won the Best Paper Award included ControlNet, a work that subverted the field of Vincentian graph AI. Since being open sourced, ControlNet has received 24k stars on GitHub. Whether it is for diffusion models or the entire field of computer vision, this paper's award is well-deserved. The honorable mention for the best paper award was awarded to another equally famous paper, Meta's "Separate Everything" ”Model SAM. Since its launch, "Segment Everything" has become the "benchmark" for various image segmentation AI models, including those that came from behind.
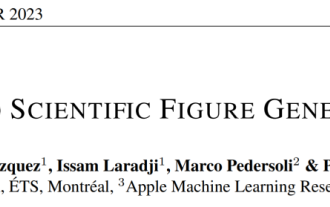
Generative AI has taken the artificial intelligence community by storm. Both individuals and enterprises have begun to be keen on creating related modal conversion applications, such as Vincent pictures, Vincent videos, Vincent music, etc. Recently, several researchers from scientific research institutions such as ServiceNow Research and LIVIA have tried to generate charts in papers based on text descriptions. To this end, they proposed a new method of FigGen, and the related paper was also included in ICLR2023 as TinyPaper. Picture paper address: https://arxiv.org/pdf/2306.00800.pdf Some people may ask, what is so difficult about generating the charts in the paper? How does this help scientific research?
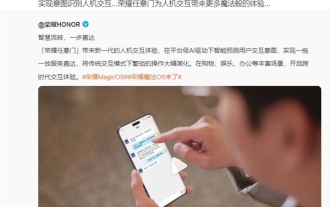
According to news on January 11, at a press conference held yesterday, Honor officially launched MagicOS 8.0. One of its special features called "Any Door" caused controversy. Luo Yonghao accused Zhao Ming on his rumor refuting account of Honor of copying the OneStep function of Smartisan phones, and shouted to Zhao Ming: "This is a naked copy of OneStep of Smartisan phones. Has Mr. Zhao been deceived by the children who made the product?" ? I believe your company will not be so shameless, and you are not Apple." In this regard, Honor officials have not publicly responded. According to Honor’s official introduction, the “any door” function brings a new generation of human-computer interaction experience.
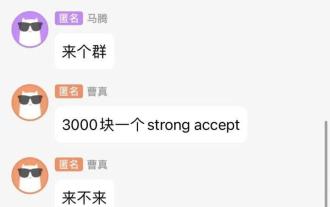
Just as the AAAI 2023 paper submission deadline was approaching, a screenshot of an anonymous chat in the AI submission group suddenly appeared on Zhihu. One of them claimed that he could provide "3,000 yuan a strong accept" service. As soon as the news came out, it immediately aroused public outrage among netizens. However, don’t rush yet. Zhihu boss "Fine Tuning" said that this is most likely just a "verbal pleasure". According to "Fine Tuning", greetings and gang crimes are unavoidable problems in any field. With the rise of openreview, the various shortcomings of cmt have become more and more clear. The space left for small circles to operate will become smaller in the future, but there will always be room. Because this is a personal problem, not a problem with the submission system and mechanism. Introducing open r
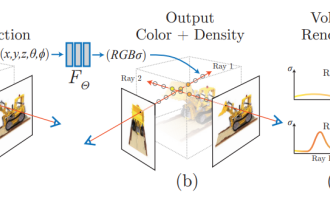
Since Neural Radiance Fields was proposed in 2020, the number of related papers has increased exponentially. It has not only become an important branch of three-dimensional reconstruction, but has also gradually become active at the research frontier as an important tool for autonomous driving. NeRF has suddenly emerged in the past two years, mainly because it skips the feature point extraction and matching, epipolar geometry and triangulation, PnP plus Bundle Adjustment and other steps of the traditional CV reconstruction pipeline, and even skips mesh reconstruction, mapping and light tracing, directly from 2D The input image is used to learn a radiation field, and then a rendered image that approximates a real photo is output from the radiation field. In other words, let an implicit three-dimensional model based on a neural network fit the specified perspective
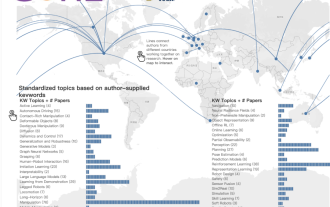
Since it was first held in 2017, CoRL has become one of the world's top academic conferences in the intersection of robotics and machine learning. CoRL is a single-theme conference for robot learning research, covering multiple topics such as robotics, machine learning and control, including theory and application. The 2023 CoRL Conference will be held in Atlanta, USA, from November 6th to 9th. According to official data, 199 papers from 25 countries were selected for CoRL this year. Popular topics include operations, reinforcement learning, and more. Although CoRL is smaller in scale than large AI academic conferences such as AAAI and CVPR, as the popularity of concepts such as large models, embodied intelligence, and humanoid robots increases this year, relevant research worthy of attention will also
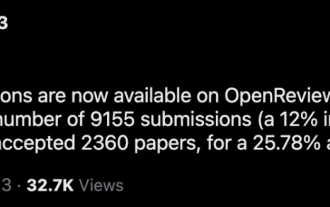
Just now, CVPR 2023 issued an article saying: This year, we received a record 9155 papers (12% more than CVPR2022), and accepted 2360 papers, with an acceptance rate of 25.78%. According to statistics, the number of submissions to CVPR only increased from 1,724 to 2,145 in the 7 years from 2010 to 2016. After 2017, it soared rapidly and entered a period of rapid growth. In 2019, it exceeded 5,000 for the first time, and by 2022, the number of submissions had reached 8,161. As you can see, a total of 9,155 papers were submitted this year, indeed setting a record. After the epidemic is relaxed, this year’s CVPR summit will be held in Canada. This year it will be a single-track conference and the traditional Oral selection will be cancelled. google research
