Can synthetic data make artificial intelligence better?
Although artificial intelligence (AI) has become more advanced due to exponential advances, the limitations of this modern technology still exist.
So, can synthetic data be the solution to all problems related to artificial intelligence?
In the fourth industrial revolution, every industry has discovered the potential of modern technologies; such as artificial intelligence (AI) and machine learning (ML).
Almost every other organization is deploying AI to create more efficient business processes and ensure better customer satisfaction. However, startups, SOHOs, and small and medium-sized businesses (SMBs) face a major problem when adopting AI – it’s known as the cold start problem. While startups and SMEs generally do not have the resources to collect big data, the cold start problem is essentially a lack of such relevant data.
On the other hand, industry giants already have the resources to collect real-world data and apply it to train their AI systems. Therefore, the odds of winning for small and medium-sized enterprises are great. In this case, synthetic data may be the necessary enabler.
Synthetic data can be the driving force behind data-driven business models. Furthermore, studies have shown that synthetic data produces the same results as real data. Synthetic data is considered cheaper and takes less time to process than real data. Therefore, the emergence of synthetic data can level the playing field currently dominated by large companies in favor of SMEs and startups.
Discover the Benefits of Synthetic Data
Synthetic data is computer-generated artificial data based on user-specified parameters to ensure the data is as close as possible to real-world historical data. Typically, game engines such as Unreal Engine and Unity are often used as simulation environments for testing and training AI-based applications such as self-driving cars. There are many advantages to developing AI-driven applications based on synthetic data. Some of the advantages include:
#1. Develop PrototypesFinding, aggregating, and modeling large amounts of relevant real-world data is a tedious process. Therefore, generating synthetic data may be the best solution. Such data will enable building prototypes and testing such prototypes to obtain the desired results before mass production. Building prototypes using synthetic data is more efficient and cost-effective than real data.
Open AI, a non-profit artificial intelligence research company, is developing a number of artificial intelligence-based applications. Among these applications, researchers have developed robots trained with synthetic data that can learn a new task after seeing an action performed just once. A California tech startup is developing an artificial intelligence platform with a vision similar to Amazon Go. The startup aims to provide checkout-free solutions for convenience stores and retailers with the help of synthetic data. They have also introduced AI-powered smart systems to monitor every shopper in the store to identify and analyze their learning patterns.
2. Ensure data privacy
In November 2018, 500 million Marriott customers were affected in a high-profile data breach. Of those 500 million people, 327 million had their data including passport information, email addresses, mailing addresses and credit card information stolen. Due to such incidents, people are worried about the security and privacy of their data.
Synthetic data can effectively solve such privacy issues. Synthetic data does not include any personal data. Therefore, data privacy can be easily ensured. Synthetic data is extremely useful in training AI systems for healthcare applications. AI systems often require real patient data. This threatens patient privacy. Synthetic data allows the development of advanced artificial intelligence applications in healthcare while maintaining patient confidentiality.
For example, researchers from Nvidia, working with the Mayo Clinic in Minnesota and the MGH and BWH Clinical Data Science Center in Boston, are using generative adversarial networks to generate synthetic data for training neural networks. The generated synthetic data contains 3,400 MRIs from the Alzheimer's Disease Neuroimaging Initiative dataset and 200 4D brain MRIs and tumors from the Multimodal Brain Tumor Image Segmentation Benchmark dataset. Likewise, simulated X-rays can be used alongside actual X-rays to train AI systems to recognize multiple health conditions.
3. Unprecedented Scenario Testing and Training
One of the most important processes in developing AI-driven applications is testing system performance. If the system is not producing the desired output, it needs to be retrained. In this case, synthetic data can prove beneficial. Synthetic data can generate scenarios to test AI systems instead of using real data or testing the system in a real environment. This method is cheaper and less time-consuming than obtaining real data.
Similarly, synthetic data can also train new or existing systems for scenarios that may arise in the future that lack real data or events. With this approach, researchers can develop more futuristic AI applications. Additionally, retraining AI systems using synthetic data is simpler because generating synthetic data is simpler than collecting accurate real-world data.
Due to these benefits, synthetic data has become an accessible alternative for testing and training autonomous vehicles. Many self-driving car developers are using simulated gaming environments like GTA V to train their AI-based systems. Likewise, May Mobility is building a self-driving micromobility service by training their vehicles using synthetic data.
Another self-driving car developer called Waymo has already tested its self-driving cars by driving 5 billion miles on simulated roads and another 8 million miles on real roads. The synthetic data approach allows developers to test their self-driving cars on simulated roads, which is much safer than direct testing on actual roads.
4. Improve data flexibility
Getting real data is a tedious process that involves paying for annotation and ensuring that any copyright infringement is avoided. Furthermore, real data can only be used in specific scenarios with sufficient historical data in a specific domain. Unlike real data, synthetic data can instantly represent any combination of objects, scenes, events, and people. Synthetic data can generate general datasets that can discover niche applications. As a result, researchers can explore endless possibilities with synthetic data. Several startups are creating an open data economy by developing training data sets that meet customer requirements.
5. Exploring the Limitations of Synthetic Data
While synthetic data can help AI reach undiscovered territories, its limitations may become a major obstacle to its mainstream deployment. For starters, synthetic data simulates several properties of real-world data, but it doesn't exactly replicate the original data. When modeling such synthetic data, AI systems will only look for common trends and situations in the real data. Therefore, rare scenarios contained in corner cases in real-world data may never be included in synthetic data.
In addition, researchers have not yet developed a mechanism to check whether the data is accurate. Finding flaws in real data and reducing them is simpler than using synthetic data. AI-driven systems already have a “dark side” that promotes unintentional bias. Using synthetic data, it may be premature to predict the scope and impact of this bias.
6. Overcoming the Challenge
The need for organizations to understand that synthetic data is a fairly new discovery. The efficiency and accuracy of such data has not been evaluated against current industry standards. Therefore, synthetic data should not be considered a stand-alone data source. Especially in applications facing safety concerns, such as healthcare applications and self-driving cars, synthetic data must be combined with real-world data to develop AI systems. But applications in retail have a lower risk factor and can easily rely on synthetic data.
For testing purposes, synthetic data is a viable and inexpensive solution. However, for other purposes, the results of an AI system need to be thoroughly studied and analyzed before employing synthetic data as a stand-alone solution. With further research, synthetic data may become more reliable for a variety of operations.
The above is the detailed content of Can synthetic data make artificial intelligence better?. For more information, please follow other related articles on the PHP Chinese website!

Hot AI Tools

Undresser.AI Undress
AI-powered app for creating realistic nude photos

AI Clothes Remover
Online AI tool for removing clothes from photos.

Undress AI Tool
Undress images for free

Clothoff.io
AI clothes remover

Video Face Swap
Swap faces in any video effortlessly with our completely free AI face swap tool!

Hot Article

Hot Tools

Notepad++7.3.1
Easy-to-use and free code editor

SublimeText3 Chinese version
Chinese version, very easy to use

Zend Studio 13.0.1
Powerful PHP integrated development environment

Dreamweaver CS6
Visual web development tools

SublimeText3 Mac version
God-level code editing software (SublimeText3)

Hot Topics
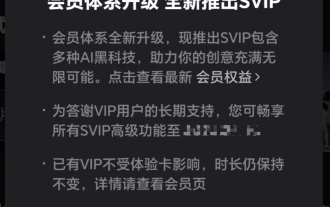
This site reported on June 27 that Jianying is a video editing software developed by FaceMeng Technology, a subsidiary of ByteDance. It relies on the Douyin platform and basically produces short video content for users of the platform. It is compatible with iOS, Android, and Windows. , MacOS and other operating systems. Jianying officially announced the upgrade of its membership system and launched a new SVIP, which includes a variety of AI black technologies, such as intelligent translation, intelligent highlighting, intelligent packaging, digital human synthesis, etc. In terms of price, the monthly fee for clipping SVIP is 79 yuan, the annual fee is 599 yuan (note on this site: equivalent to 49.9 yuan per month), the continuous monthly subscription is 59 yuan per month, and the continuous annual subscription is 499 yuan per year (equivalent to 41.6 yuan per month) . In addition, the cut official also stated that in order to improve the user experience, those who have subscribed to the original VIP
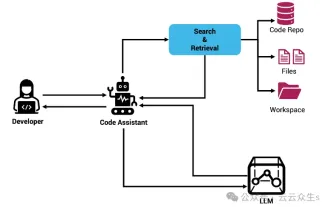
Improve developer productivity, efficiency, and accuracy by incorporating retrieval-enhanced generation and semantic memory into AI coding assistants. Translated from EnhancingAICodingAssistantswithContextUsingRAGandSEM-RAG, author JanakiramMSV. While basic AI programming assistants are naturally helpful, they often fail to provide the most relevant and correct code suggestions because they rely on a general understanding of the software language and the most common patterns of writing software. The code generated by these coding assistants is suitable for solving the problems they are responsible for solving, but often does not conform to the coding standards, conventions and styles of the individual teams. This often results in suggestions that need to be modified or refined in order for the code to be accepted into the application

To learn more about AIGC, please visit: 51CTOAI.x Community https://www.51cto.com/aigc/Translator|Jingyan Reviewer|Chonglou is different from the traditional question bank that can be seen everywhere on the Internet. These questions It requires thinking outside the box. Large Language Models (LLMs) are increasingly important in the fields of data science, generative artificial intelligence (GenAI), and artificial intelligence. These complex algorithms enhance human skills and drive efficiency and innovation in many industries, becoming the key for companies to remain competitive. LLM has a wide range of applications. It can be used in fields such as natural language processing, text generation, speech recognition and recommendation systems. By learning from large amounts of data, LLM is able to generate text
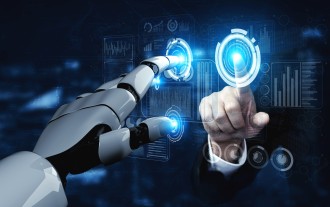
Large Language Models (LLMs) are trained on huge text databases, where they acquire large amounts of real-world knowledge. This knowledge is embedded into their parameters and can then be used when needed. The knowledge of these models is "reified" at the end of training. At the end of pre-training, the model actually stops learning. Align or fine-tune the model to learn how to leverage this knowledge and respond more naturally to user questions. But sometimes model knowledge is not enough, and although the model can access external content through RAG, it is considered beneficial to adapt the model to new domains through fine-tuning. This fine-tuning is performed using input from human annotators or other LLM creations, where the model encounters additional real-world knowledge and integrates it
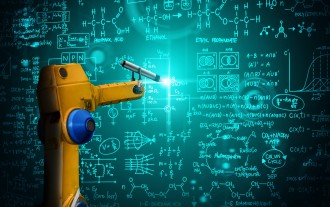
Machine learning is an important branch of artificial intelligence that gives computers the ability to learn from data and improve their capabilities without being explicitly programmed. Machine learning has a wide range of applications in various fields, from image recognition and natural language processing to recommendation systems and fraud detection, and it is changing the way we live. There are many different methods and theories in the field of machine learning, among which the five most influential methods are called the "Five Schools of Machine Learning". The five major schools are the symbolic school, the connectionist school, the evolutionary school, the Bayesian school and the analogy school. 1. Symbolism, also known as symbolism, emphasizes the use of symbols for logical reasoning and expression of knowledge. This school of thought believes that learning is a process of reverse deduction, through existing
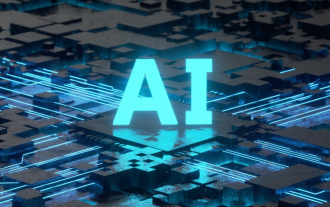
Editor |ScienceAI Question Answering (QA) data set plays a vital role in promoting natural language processing (NLP) research. High-quality QA data sets can not only be used to fine-tune models, but also effectively evaluate the capabilities of large language models (LLM), especially the ability to understand and reason about scientific knowledge. Although there are currently many scientific QA data sets covering medicine, chemistry, biology and other fields, these data sets still have some shortcomings. First, the data form is relatively simple, most of which are multiple-choice questions. They are easy to evaluate, but limit the model's answer selection range and cannot fully test the model's ability to answer scientific questions. In contrast, open-ended Q&A
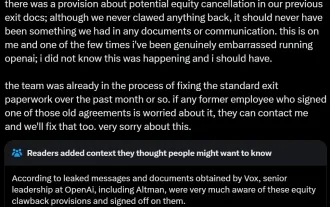
Last week, amid the internal wave of resignations and external criticism, OpenAI was plagued by internal and external troubles: - The infringement of the widow sister sparked global heated discussions - Employees signing "overlord clauses" were exposed one after another - Netizens listed Ultraman's "seven deadly sins" Rumors refuting: According to leaked information and documents obtained by Vox, OpenAI’s senior leadership, including Altman, was well aware of these equity recovery provisions and signed off on them. In addition, there is a serious and urgent issue facing OpenAI - AI safety. The recent departures of five security-related employees, including two of its most prominent employees, and the dissolution of the "Super Alignment" team have once again put OpenAI's security issues in the spotlight. Fortune magazine reported that OpenA
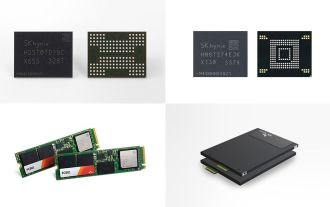
According to news from this site on August 1, SK Hynix released a blog post today (August 1), announcing that it will attend the Global Semiconductor Memory Summit FMS2024 to be held in Santa Clara, California, USA from August 6 to 8, showcasing many new technologies. generation product. Introduction to the Future Memory and Storage Summit (FutureMemoryandStorage), formerly the Flash Memory Summit (FlashMemorySummit) mainly for NAND suppliers, in the context of increasing attention to artificial intelligence technology, this year was renamed the Future Memory and Storage Summit (FutureMemoryandStorage) to invite DRAM and storage vendors and many more players. New product SK hynix launched last year
