


Virtual-real domain adaptation method for autonomous driving lane detection and classification
arXiv paper "Sim-to-Real Domain Adaptation for Lane Detection and Classification in Autonomous Driving", May 2022, work at the University of Waterloo, Canada.
While supervised detection and classification frameworks for autonomous driving require large annotated datasets, Unsupervised Domain Adaptation (UDA) driven by synthetic data generated by illuminating real simulated environments , Unsupervised Domain Adaptation) method is a low-cost, less time-consuming solution. This paper proposes a UDA scheme of adversarial discriminative and generative methods for lane line detection and classification applications in autonomous driving.
Also introduces the Simulanes dataset generator, which takes advantage of CARLA's huge traffic scenes and weather conditions to create a natural synthetic dataset. The proposed UDA framework takes the labeled synthetic dataset as the source domain, while the target domain is the unlabeled real data. Use adversarial generation and feature discriminator to debug the learning model and predict the lane location and category of the target domain. Evaluation is performed with real and synthetic datasets.
The open source UDA framework is atgithubcom/anita-hu/sim2real-lane-detection, and the data set generator is at github.com/anita-hu/simulanes.
Real-world driving is diverse, with varying traffic conditions, weather, and surrounding environments. Therefore, the diversity of simulation scenarios is crucial to the good adaptability of the model in the real world. There are many open source simulators for autonomous driving, namely CARLA and LGSVL. This article chooses CARLA to generate the simulation data set. In addition to the flexible Python API, CARLA also contains rich pre-drawn map content covering urban, rural and highway scenes.
Simulation data generator Simulanes generates a variety of simulation scenarios in urban, rural and highway environments, including 15 lane categories and dynamic weather. The figure shows samples from the synthetic dataset. Pedestrian and vehicle participants are randomly generated and placed on the map, increasing the difficulty of the dataset through occlusion. According to the TuSimple and CULane datasets, the maximum number of lanes near the vehicle is limited to 4, and row anchors are used as labels.
Since the CARLA simulator does not directly provide lane location labels, CARLA's waypoint system is used to generate labels. A CARLA waypoint is a predefined position for the vehicle autopilot to follow, located in the center of the lane. In order to obtain the lane position label, the waypoint of the current lane is moved left and right by W/2, where W is the lane width given by the simulator. These moved waypoints are then projected into the camera coordinate system and spline-fitted to generate labels along predetermined row anchor points. The class label is given by the simulator and is one of 15 classes.
To generate a dataset with N frames, divide N evenly across all available maps. From the default CARLA map, towns 1, 3, 4, 5, 7 and 10 were used, while towns 2 and 6 were not used due to differences between the extracted lane position labels and the lane positions of the image. For each map, vehicle participants are spawned at random locations and move randomly. Dynamic weather is achieved by smoothly changing the position of the sun as a sinusoidal function of time and occasionally producing storms, which affect the appearance of the environment through variables such as cloud cover, water volume and standing water. To avoid saving multiple frames at the same location, check if the vehicle has moved from the previous frame's location and regenerate a new vehicle if it has been stationary for too long.
When the sim-to-real algorithm is applied to lane detection, an end-to-end approach is adopted and the Ultra-Fast-Lane-Detection (UFLD) model is used as the basic network. UFLD was chosen because its lightweight architecture can achieve 300 frames/second at the same input resolution while achieving performance comparable to state-of-the-art methods. UFLD formulates the lane detection task as a row-based selection method, where each lane is represented by a series of horizontal positions of predefined rows, i.e., row anchors. For each row anchor, the position is divided into w grid cells. For the i-th lane and j-th row anchor, location prediction becomes a classification problem, where the model outputs the probability Pi,j of selecting (w 1) grid cell. The additional dimension in the output is no lanes.
UFLD proposes an auxiliary segmentation branch to aggregate features at multiple scales to model local features. This is only used during training. With the UFLD method, cross-entropy loss is used for segmentation loss Lseg. For lane classification, a small branch of the fully connected (FC) layer is added to receive the same features as the FC layer for lane position prediction. The lane classification loss Lcls also uses cross-entropy loss.
In order to alleviate the domain drift problem of UDA settings, UNIT ("Unsupervised Image-to-Image Translation Networks", NIPS, 2017) & MUNIT## are adopted #("Multimodal unsupervised image-to-image translation," ECCV 2018) adversarial generation method, and adversarial discriminative method using feature discriminator. As shown in the figure: an adversarial generation method (A) and an adversarial discrimination method (B) are proposed. UNIT and MUNIT are represented in (A), which shows the generator input for image translation. Additional style inputs to MUNIT are shown with dashed blue lines. For simplicity, the MUNIT-style encoder output is omitted as it is not used for image translation.
The above is the detailed content of Virtual-real domain adaptation method for autonomous driving lane detection and classification. For more information, please follow other related articles on the PHP Chinese website!

Hot AI Tools

Undresser.AI Undress
AI-powered app for creating realistic nude photos

AI Clothes Remover
Online AI tool for removing clothes from photos.

Undress AI Tool
Undress images for free

Clothoff.io
AI clothes remover

Video Face Swap
Swap faces in any video effortlessly with our completely free AI face swap tool!

Hot Article

Hot Tools

Notepad++7.3.1
Easy-to-use and free code editor

SublimeText3 Chinese version
Chinese version, very easy to use

Zend Studio 13.0.1
Powerful PHP integrated development environment

Dreamweaver CS6
Visual web development tools

SublimeText3 Mac version
God-level code editing software (SublimeText3)

Hot Topics
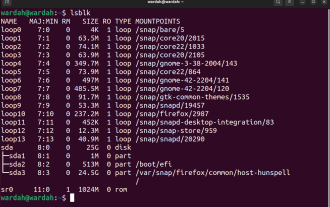
DDREASE is a tool for recovering data from file or block devices such as hard drives, SSDs, RAM disks, CDs, DVDs and USB storage devices. It copies data from one block device to another, leaving corrupted data blocks behind and moving only good data blocks. ddreasue is a powerful recovery tool that is fully automated as it does not require any interference during recovery operations. Additionally, thanks to the ddasue map file, it can be stopped and resumed at any time. Other key features of DDREASE are as follows: It does not overwrite recovered data but fills the gaps in case of iterative recovery. However, it can be truncated if the tool is instructed to do so explicitly. Recover data from multiple files or blocks to a single
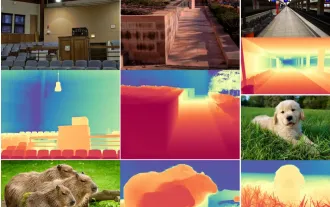
0.What does this article do? We propose DepthFM: a versatile and fast state-of-the-art generative monocular depth estimation model. In addition to traditional depth estimation tasks, DepthFM also demonstrates state-of-the-art capabilities in downstream tasks such as depth inpainting. DepthFM is efficient and can synthesize depth maps within a few inference steps. Let’s read about this work together ~ 1. Paper information title: DepthFM: FastMonocularDepthEstimationwithFlowMatching Author: MingGui, JohannesS.Fischer, UlrichPrestel, PingchuanMa, Dmytr
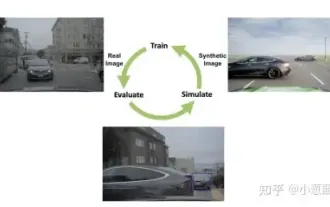
Yesterday during the interview, I was asked whether I had done any long-tail related questions, so I thought I would give a brief summary. The long-tail problem of autonomous driving refers to edge cases in autonomous vehicles, that is, possible scenarios with a low probability of occurrence. The perceived long-tail problem is one of the main reasons currently limiting the operational design domain of single-vehicle intelligent autonomous vehicles. The underlying architecture and most technical issues of autonomous driving have been solved, and the remaining 5% of long-tail problems have gradually become the key to restricting the development of autonomous driving. These problems include a variety of fragmented scenarios, extreme situations, and unpredictable human behavior. The "long tail" of edge scenarios in autonomous driving refers to edge cases in autonomous vehicles (AVs). Edge cases are possible scenarios with a low probability of occurrence. these rare events
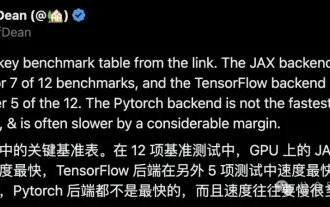
The performance of JAX, promoted by Google, has surpassed that of Pytorch and TensorFlow in recent benchmark tests, ranking first in 7 indicators. And the test was not done on the TPU with the best JAX performance. Although among developers, Pytorch is still more popular than Tensorflow. But in the future, perhaps more large models will be trained and run based on the JAX platform. Models Recently, the Keras team benchmarked three backends (TensorFlow, JAX, PyTorch) with the native PyTorch implementation and Keras2 with TensorFlow. First, they select a set of mainstream
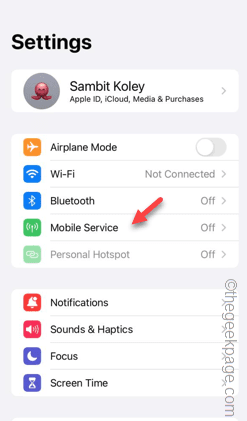
Facing lag, slow mobile data connection on iPhone? Typically, the strength of cellular internet on your phone depends on several factors such as region, cellular network type, roaming type, etc. There are some things you can do to get a faster, more reliable cellular Internet connection. Fix 1 – Force Restart iPhone Sometimes, force restarting your device just resets a lot of things, including the cellular connection. Step 1 – Just press the volume up key once and release. Next, press the Volume Down key and release it again. Step 2 – The next part of the process is to hold the button on the right side. Let the iPhone finish restarting. Enable cellular data and check network speed. Check again Fix 2 – Change data mode While 5G offers better network speeds, it works better when the signal is weaker
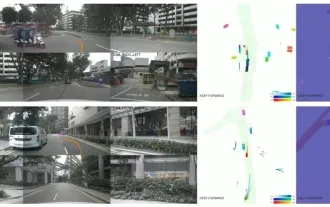
In the past month, due to some well-known reasons, I have had very intensive exchanges with various teachers and classmates in the industry. An inevitable topic in the exchange is naturally end-to-end and the popular Tesla FSDV12. I would like to take this opportunity to sort out some of my thoughts and opinions at this moment for your reference and discussion. How to define an end-to-end autonomous driving system, and what problems should be expected to be solved end-to-end? According to the most traditional definition, an end-to-end system refers to a system that inputs raw information from sensors and directly outputs variables of concern to the task. For example, in image recognition, CNN can be called end-to-end compared to the traditional feature extractor + classifier method. In autonomous driving tasks, input data from various sensors (camera/LiDAR
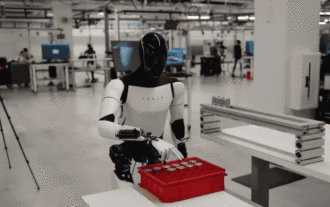
The latest video of Tesla's robot Optimus is released, and it can already work in the factory. At normal speed, it sorts batteries (Tesla's 4680 batteries) like this: The official also released what it looks like at 20x speed - on a small "workstation", picking and picking and picking: This time it is released One of the highlights of the video is that Optimus completes this work in the factory, completely autonomously, without human intervention throughout the process. And from the perspective of Optimus, it can also pick up and place the crooked battery, focusing on automatic error correction: Regarding Optimus's hand, NVIDIA scientist Jim Fan gave a high evaluation: Optimus's hand is the world's five-fingered robot. One of the most dexterous. Its hands are not only tactile
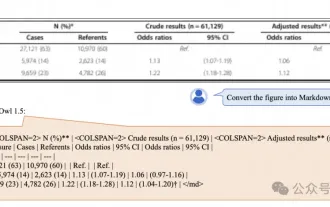
New SOTA for multimodal document understanding capabilities! Alibaba's mPLUG team released the latest open source work mPLUG-DocOwl1.5, which proposed a series of solutions to address the four major challenges of high-resolution image text recognition, general document structure understanding, instruction following, and introduction of external knowledge. Without further ado, let’s look at the effects first. One-click recognition and conversion of charts with complex structures into Markdown format: Charts of different styles are available: More detailed text recognition and positioning can also be easily handled: Detailed explanations of document understanding can also be given: You know, "Document Understanding" is currently An important scenario for the implementation of large language models. There are many products on the market to assist document reading. Some of them mainly use OCR systems for text recognition and cooperate with LLM for text processing.
