Interpreting CRISP-ML(Q): Machine Learning Lifecycle Process
Translator | Bugatti
Reviewer | Sun Shujuan
Currently, there are no standard practices for building and managing machine learning (ML) applications. Machine learning projects are poorly organized, lack repeatability, and tend to fail outright in the long run. Therefore, we need a process to help us maintain quality, sustainability, robustness, and cost management throughout the machine learning lifecycle.
Figure 1. Machine Learning Development Lifecycle Process
Cross-industry standard process for developing machine learning applications using quality assurance methods (CRISP-ML(Q )) is an upgraded version of CRISP-DM to ensure the quality of machine learning products.
CRISP-ML (Q) has six separate phases:
1. Business and data understanding
2. Data preparation
3. Model Engineering
4. Model Evaluation
5. Model Deployment
6. Monitoring and Maintenance
These stages require continuous iteration and exploration to build better s solution. Even if there is order in the framework, the output of a later stage can determine whether we need to re-examine the previous stage.
Figure 2. Quality assurance at each stage
Quality assurance methods are introduced into each stage of the framework. This approach has requirements and constraints, such as performance metrics, data quality requirements, and robustness. It helps reduce risks that impact the success of machine learning applications. It can be achieved by continuously monitoring and maintaining the entire system.
For example: In e-commerce companies, data and concept drift will lead to model degradation; if we do not deploy a system to monitor these changes, the company will suffer losses, that is, lose customers.
Business and Data Understanding
At the beginning of the development process, we need to determine the project scope, success criteria, and feasibility of the ML application. After that, we started the data collection and quality verification process. The process is long and challenging.
Scope: What we hope to achieve by using the machine learning process. Is it to retain customers or reduce operating costs through automation?
Success Criteria: We must define clear and measurable business, machine learning (statistical indicators) and economic (KPI) success indicators.
Feasibility: We need to ensure data availability, suitability for machine learning applications, legal constraints, robustness, scalability, interpretability, and resource requirements.
Data Collection: By collecting data, versioning it for reproducibility and ensuring a continuous flow of real and generated data.
Data Quality Verification: Ensure quality by maintaining data descriptions, requirements and validations.
To ensure quality and reproducibility, we need to record the statistical properties of the data and the data generation process.
Data preparation
The second stage is very simple. We will prepare the data for the modeling phase. This includes data selection, data cleaning, feature engineering, data enhancement and normalization.
1. We start with feature selection, data selection, and handling of imbalanced classes through oversampling or undersampling.
2. Then, focus on reducing noise and handling missing values. For quality assurance purposes, we will add data unit tests to reduce erroneous values.
3. Depending on the model, we perform feature engineering and data augmentation such as one-hot encoding and clustering.
4. Normalize and extend data. This reduces the risk of biased features.
To ensure reproducibility, we created data modeling, transformation, and feature engineering pipelines.
Model Engineering
The constraints and requirements of the business and data understanding phases will determine the modeling phase. We need to understand the business problems and how we will develop machine learning models to solve them. We will focus on model selection, optimization and training, ensuring model performance metrics, robustness, scalability, interpretability, and optimizing storage and computing resources.
1. Research on model architecture and similar business problems.
2. Define model performance indicators.
3. Model selection.
4. Understand domain knowledge by integrating experts.
5. Model training.
6. Model compression and integration.
To ensure quality and reproducibility, we will store and version control model metadata, such as model architecture, training and validation data, hyperparameters, and environment descriptions.
Finally, we will track ML experiments and create ML pipelines to create repeatable training processes.
Model Evaluation
This is the stage where we test and ensure the model is ready for deployment.
- We will test the model performance on the test data set.
- Evaluate the robustness of the model by providing random or fake data.
- Enhance the interpretability of the model to meet regulatory requirements.
- Automatically or with domain experts, compare results to initial success metrics.
Every step of the evaluation phase is documented for quality assurance.
Model Deployment
Model deployment is the stage where we integrate machine learning models into existing systems. The model can be deployed on servers, browsers, software and edge devices. Predictions from the model are available in BI dashboards, APIs, web applications and plug-ins.
Model deployment process:
- Define hardware inference.
- Model evaluation in production environment.
- Ensure user acceptance and usability.
- Provide backup plans to minimize losses.
- Deployment strategy.
Monitoring and Maintenance
Models in production environments require continuous monitoring and maintenance. We will monitor model timeliness, hardware performance, and software performance.
Continuous monitoring is the first part of the process; if performance drops below a threshold, a decision is made automatically to retrain the model on new data. Furthermore, the maintenance part is not limited to model retraining. It requires decision-making mechanisms, acquiring new data, updating software and hardware, and improving ML processes based on business use cases.
In short, it is continuous integration, training and deployment of ML models.
Conclusion
Training and validating models is a small part of ML applications. Turning an initial idea into reality requires several processes. In this article we introduce CRISP-ML(Q) and how it focuses on risk assessment and quality assurance.
We first define the business goals, collect and clean data, build the model, verify the model with a test data set, and then deploy it to the production environment.
The key components of this framework are ongoing monitoring and maintenance. We will monitor data and software and hardware metrics to determine whether to retrain the model or upgrade the system.
If you are new to machine learning operations and want to learn more, read the free MLOps course reviewed by DataTalks.Club. You'll gain hands-on experience in all six phases, understanding the practical implementation of CRISP-ML.
Original title: Making Sense of CRISP-ML(Q): The Machine Learning Lifecycle Process, Author: Abid Ali Awan
The above is the detailed content of Interpreting CRISP-ML(Q): Machine Learning Lifecycle Process. For more information, please follow other related articles on the PHP Chinese website!

Hot AI Tools

Undresser.AI Undress
AI-powered app for creating realistic nude photos

AI Clothes Remover
Online AI tool for removing clothes from photos.

Undress AI Tool
Undress images for free

Clothoff.io
AI clothes remover

Video Face Swap
Swap faces in any video effortlessly with our completely free AI face swap tool!

Hot Article

Hot Tools

Notepad++7.3.1
Easy-to-use and free code editor

SublimeText3 Chinese version
Chinese version, very easy to use

Zend Studio 13.0.1
Powerful PHP integrated development environment

Dreamweaver CS6
Visual web development tools

SublimeText3 Mac version
God-level code editing software (SublimeText3)

Hot Topics
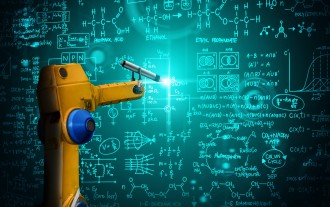
Machine learning is an important branch of artificial intelligence that gives computers the ability to learn from data and improve their capabilities without being explicitly programmed. Machine learning has a wide range of applications in various fields, from image recognition and natural language processing to recommendation systems and fraud detection, and it is changing the way we live. There are many different methods and theories in the field of machine learning, among which the five most influential methods are called the "Five Schools of Machine Learning". The five major schools are the symbolic school, the connectionist school, the evolutionary school, the Bayesian school and the analogy school. 1. Symbolism, also known as symbolism, emphasizes the use of symbols for logical reasoning and expression of knowledge. This school of thought believes that learning is a process of reverse deduction, through existing
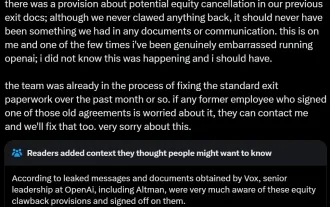
Last week, amid the internal wave of resignations and external criticism, OpenAI was plagued by internal and external troubles: - The infringement of the widow sister sparked global heated discussions - Employees signing "overlord clauses" were exposed one after another - Netizens listed Ultraman's "seven deadly sins" Rumors refuting: According to leaked information and documents obtained by Vox, OpenAI’s senior leadership, including Altman, was well aware of these equity recovery provisions and signed off on them. In addition, there is a serious and urgent issue facing OpenAI - AI safety. The recent departures of five security-related employees, including two of its most prominent employees, and the dissolution of the "Super Alignment" team have once again put OpenAI's security issues in the spotlight. Fortune magazine reported that OpenA
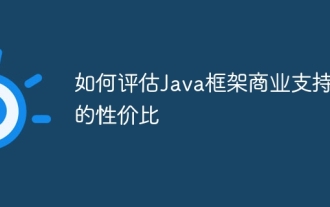
Evaluating the cost/performance of commercial support for a Java framework involves the following steps: Determine the required level of assurance and service level agreement (SLA) guarantees. The experience and expertise of the research support team. Consider additional services such as upgrades, troubleshooting, and performance optimization. Weigh business support costs against risk mitigation and increased efficiency.
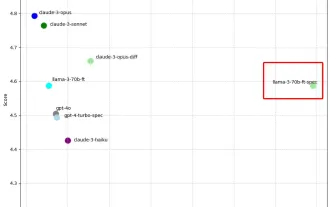
70B model, 1000 tokens can be generated in seconds, which translates into nearly 4000 characters! The researchers fine-tuned Llama3 and introduced an acceleration algorithm. Compared with the native version, the speed is 13 times faster! Not only is it fast, its performance on code rewriting tasks even surpasses GPT-4o. This achievement comes from anysphere, the team behind the popular AI programming artifact Cursor, and OpenAI also participated in the investment. You must know that on Groq, a well-known fast inference acceleration framework, the inference speed of 70BLlama3 is only more than 300 tokens per second. With the speed of Cursor, it can be said that it achieves near-instant complete code file editing. Some people call it a good guy, if you put Curs
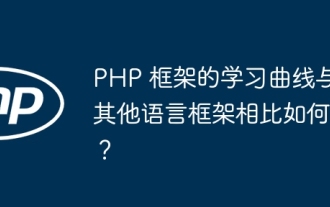
The learning curve of a PHP framework depends on language proficiency, framework complexity, documentation quality, and community support. The learning curve of PHP frameworks is higher when compared to Python frameworks and lower when compared to Ruby frameworks. Compared to Java frameworks, PHP frameworks have a moderate learning curve but a shorter time to get started.

The lightweight PHP framework improves application performance through small size and low resource consumption. Its features include: small size, fast startup, low memory usage, improved response speed and throughput, and reduced resource consumption. Practical case: SlimFramework creates REST API, only 500KB, high responsiveness and high throughput
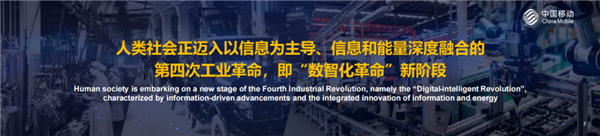
According to news on June 26, at the opening ceremony of the 2024 World Mobile Communications Conference Shanghai (MWC Shanghai), China Mobile Chairman Yang Jie delivered a speech. He said that currently, human society is entering the fourth industrial revolution, which is dominated by information and deeply integrated with information and energy, that is, the "digital intelligence revolution", and the formation of new productive forces is accelerating. Yang Jie believes that from the "mechanization revolution" driven by steam engines, to the "electrification revolution" driven by electricity, internal combustion engines, etc., to the "information revolution" driven by computers and the Internet, each round of industrial revolution is based on "information and "Energy" is the main line, bringing productivity development
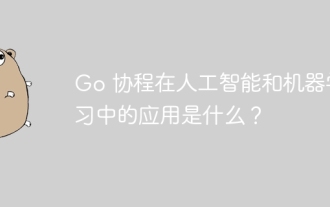
The applications of Go coroutines in the field of artificial intelligence and machine learning include: real-time training and prediction: parallel processing tasks to improve performance. Parallel hyperparameter optimization: Explore different settings simultaneously to speed up training. Distributed computing: Easily distribute tasks and take advantage of the cloud or cluster.
