


13 billion parameters, 8 A100 training, UC Berkeley releases dialogue model Koala
Since Meta released and open sourced the LLaMA series of models, researchers from Stanford University, UC Berkeley and other institutions have carried out "second creation" on the basis of LLaMA, and have successively launched Alpaca, Vicuna and other " Alpaca" large model.
Alpaca has become the new leader in the open source community. Due to the abundance of "secondary creations", the English words for the biological alpaca genus are almost out of use, but it is also possible to name the large model after other animals.
Recently, UC Berkeley’s Berkeley Artificial Intelligence Institute (BAIR) released a conversation model Koala (literally translated as Koala) that can run on consumer-grade GPUs. Koala fine-tunes the LLaMA model using conversation data collected from the web.
Project address: https://bair.berkeley.edu/blog/2023/04/03/koala/
Koala has launched an online test demo:
- ##Demo address: https://chat.lmsys.org/?model=koala-13b
- Open source address: https://github.com/young-geng/EasyLM
Like Vicuna, Koala also uses conversation data collected from the network to fine-tune the LLaMA model, with a focus on ChatGPT Public data of closed-source large model dialogues.
The research team stated that the Koala model is implemented in EasyLM using JAX/Flax and the Koala model is trained on a single Nvidia DGX server equipped with 8 A100 GPUs. It takes 6 hours to complete 2 epochs of training. The cost of such training is typically less than $100 on public cloud computing platforms.
The research team experimentally compared Koala with ChatGPT and Stanford University's Alpaca. The results showed that Koala-13B with 13 billion parameters can effectively respond to various user queries and generate Response is generally better than Alpaca's and is comparable to ChatGPT's performance in more than half of the cases.
The most important significance of Koala is that it shows that when trained on a higher quality data set, a model small enough to run locally can also achieve excellent performance similar to that of a large model . This means that the open source community should work harder to curate high-quality datasets, as this may lead to more secure, realistic, and powerful models than simply increasing the size of existing systems. From this perspective, Koala is a small but refined alternative to ChatGPT.
However, Koala is only a research prototype and still has significant flaws in content, security, and reliability, and should not be used for any purpose other than research.
Datasets and TrainingThe main hurdle in building a conversation model is managing the training data. Large conversation models such as ChatGPT, Bard, Bing Chat, and Claude all use proprietary datasets with extensive human annotations. To build Koala's training dataset, the research team collected and curated conversation data from the web and public datasets, which contain data shared publicly by users speaking to large language models such as ChatGPT.
Unlike other models that crawl as much network data as possible to maximize the data set, Koala focuses on collecting small high-quality data sets, including the question and answer part of public data sets, human Feedback (positive and negative) and dialogue with existing language models. Specifically, Koala's training data set includes the following parts:
ChatGPT distillation data:
- Publicly available chatGPT conversation data (ShareGPT);
- Human ChatGPT comparison corpus (HC3), which uses both human and ChatGPT responses from the HC3 dataset.
Open source data:
- Open Instruction Generalist (OIG);
- Dataset used by the Stanford Alpaca model;
- Anthropic HH ;
- OpenAI WebGPT;
- OpenAI Summarization.
Experimentation and Evaluation
This study conducted a manual evaluation comparing the generation of Koala-All with Koala-Distill, Alpaca and ChatGPT. The results are compared and the results are shown in the figure below. Among them, two different data sets are used for testing, one is Stanford's Alpaca test set, which includes 180 test queries (Alpaca Test Set), and the other is the Koala Test Set.
Overall, the Koala model is sufficient to demonstrate many features of LLM, while being small enough to facilitate fine-tuning or in situations where computing resources are limited. Use below. The research team hopes that the Koala model will become a useful platform for future academic research on large-scale language models. Potential research application directions may include:
- Safety and alignment: Koala allows further research on language models security and better alignment with human intent.
- Model Bias: Koala enables us to better understand bias in large language models, delve into quality issues in conversation datasets, and ultimately help improve the performance of large language models.
- Understanding large language models: Because Koala models can run on relatively cheap consumer-grade GPUs and perform a variety of tasks, Koala allows us to better examine and understand conversational language The internal structure of the model makes the language model more interpretable.
The above is the detailed content of 13 billion parameters, 8 A100 training, UC Berkeley releases dialogue model Koala. For more information, please follow other related articles on the PHP Chinese website!

Hot AI Tools

Undresser.AI Undress
AI-powered app for creating realistic nude photos

AI Clothes Remover
Online AI tool for removing clothes from photos.

Undress AI Tool
Undress images for free

Clothoff.io
AI clothes remover

Video Face Swap
Swap faces in any video effortlessly with our completely free AI face swap tool!

Hot Article

Hot Tools

Notepad++7.3.1
Easy-to-use and free code editor

SublimeText3 Chinese version
Chinese version, very easy to use

Zend Studio 13.0.1
Powerful PHP integrated development environment

Dreamweaver CS6
Visual web development tools

SublimeText3 Mac version
God-level code editing software (SublimeText3)

Hot Topics
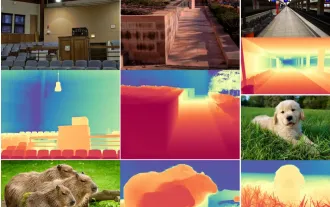
0.What does this article do? We propose DepthFM: a versatile and fast state-of-the-art generative monocular depth estimation model. In addition to traditional depth estimation tasks, DepthFM also demonstrates state-of-the-art capabilities in downstream tasks such as depth inpainting. DepthFM is efficient and can synthesize depth maps within a few inference steps. Let’s read about this work together ~ 1. Paper information title: DepthFM: FastMonocularDepthEstimationwithFlowMatching Author: MingGui, JohannesS.Fischer, UlrichPrestel, PingchuanMa, Dmytr
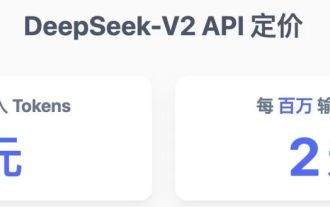
Imagine an artificial intelligence model that not only has the ability to surpass traditional computing, but also achieves more efficient performance at a lower cost. This is not science fiction, DeepSeek-V2[1], the world’s most powerful open source MoE model is here. DeepSeek-V2 is a powerful mixture of experts (MoE) language model with the characteristics of economical training and efficient inference. It consists of 236B parameters, 21B of which are used to activate each marker. Compared with DeepSeek67B, DeepSeek-V2 has stronger performance, while saving 42.5% of training costs, reducing KV cache by 93.3%, and increasing the maximum generation throughput to 5.76 times. DeepSeek is a company exploring general artificial intelligence
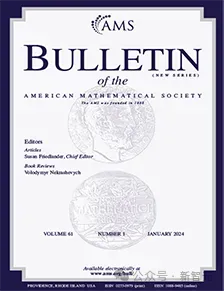
AI is indeed changing mathematics. Recently, Tao Zhexuan, who has been paying close attention to this issue, forwarded the latest issue of "Bulletin of the American Mathematical Society" (Bulletin of the American Mathematical Society). Focusing on the topic "Will machines change mathematics?", many mathematicians expressed their opinions. The whole process was full of sparks, hardcore and exciting. The author has a strong lineup, including Fields Medal winner Akshay Venkatesh, Chinese mathematician Zheng Lejun, NYU computer scientist Ernest Davis and many other well-known scholars in the industry. The world of AI has changed dramatically. You know, many of these articles were submitted a year ago.
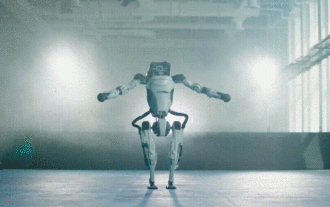
Boston Dynamics Atlas officially enters the era of electric robots! Yesterday, the hydraulic Atlas just "tearfully" withdrew from the stage of history. Today, Boston Dynamics announced that the electric Atlas is on the job. It seems that in the field of commercial humanoid robots, Boston Dynamics is determined to compete with Tesla. After the new video was released, it had already been viewed by more than one million people in just ten hours. The old people leave and new roles appear. This is a historical necessity. There is no doubt that this year is the explosive year of humanoid robots. Netizens commented: The advancement of robots has made this year's opening ceremony look like a human, and the degree of freedom is far greater than that of humans. But is this really not a horror movie? At the beginning of the video, Atlas is lying calmly on the ground, seemingly on his back. What follows is jaw-dropping
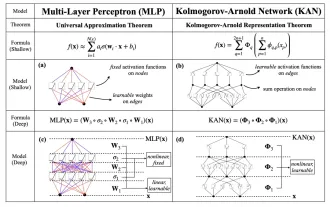
Earlier this month, researchers from MIT and other institutions proposed a very promising alternative to MLP - KAN. KAN outperforms MLP in terms of accuracy and interpretability. And it can outperform MLP running with a larger number of parameters with a very small number of parameters. For example, the authors stated that they used KAN to reproduce DeepMind's results with a smaller network and a higher degree of automation. Specifically, DeepMind's MLP has about 300,000 parameters, while KAN only has about 200 parameters. KAN has a strong mathematical foundation like MLP. MLP is based on the universal approximation theorem, while KAN is based on the Kolmogorov-Arnold representation theorem. As shown in the figure below, KAN has
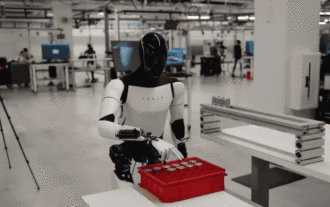
The latest video of Tesla's robot Optimus is released, and it can already work in the factory. At normal speed, it sorts batteries (Tesla's 4680 batteries) like this: The official also released what it looks like at 20x speed - on a small "workstation", picking and picking and picking: This time it is released One of the highlights of the video is that Optimus completes this work in the factory, completely autonomously, without human intervention throughout the process. And from the perspective of Optimus, it can also pick up and place the crooked battery, focusing on automatic error correction: Regarding Optimus's hand, NVIDIA scientist Jim Fan gave a high evaluation: Optimus's hand is the world's five-fingered robot. One of the most dexterous. Its hands are not only tactile
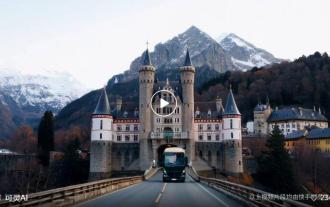
What? Is Zootopia brought into reality by domestic AI? Exposed together with the video is a new large-scale domestic video generation model called "Keling". Sora uses a similar technical route and combines a number of self-developed technological innovations to produce videos that not only have large and reasonable movements, but also simulate the characteristics of the physical world and have strong conceptual combination capabilities and imagination. According to the data, Keling supports the generation of ultra-long videos of up to 2 minutes at 30fps, with resolutions up to 1080p, and supports multiple aspect ratios. Another important point is that Keling is not a demo or video result demonstration released by the laboratory, but a product-level application launched by Kuaishou, a leading player in the short video field. Moreover, the main focus is to be pragmatic, not to write blank checks, and to go online as soon as it is released. The large model of Ke Ling is already available in Kuaiying.
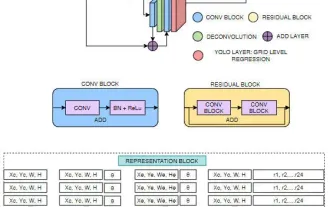
Target detection is a relatively mature problem in autonomous driving systems, among which pedestrian detection is one of the earliest algorithms to be deployed. Very comprehensive research has been carried out in most papers. However, distance perception using fisheye cameras for surround view is relatively less studied. Due to large radial distortion, standard bounding box representation is difficult to implement in fisheye cameras. To alleviate the above description, we explore extended bounding box, ellipse, and general polygon designs into polar/angular representations and define an instance segmentation mIOU metric to analyze these representations. The proposed model fisheyeDetNet with polygonal shape outperforms other models and simultaneously achieves 49.5% mAP on the Valeo fisheye camera dataset for autonomous driving
