


Re-examining the Prompt optimization problem, prediction bias makes language model context learning stronger
LLMs have achieved good performance under In-context Learning, but choosing different examples will lead to completely different performances. A recent research work proposes a prompt search strategy from the perspective of predictive bias, and approximately finds the optimal combination of examples.
- Paper link: https://arxiv.org/abs/2303.13217
- Code link: https://github.com/MaHuanAAA /g_fair_searching
Research Introduction
Large language models have shown amazing capabilities in context learning. These models can learn from contexts constructed from several input and output examples without the need for fine-tuning. Optimizations are applied directly to many downstream tasks. However, previous research has shown that contextual learning can exhibit a high degree of instability due to changes in training examples, example order, and prompt formats. Therefore, constructing appropriate prompts is crucial to improve the performance of contextual learning.
Previous research usually studies this issue from two directions: (1) prompt tuning in the encoding space (prompt tuning), (2) searching in the original space (prompt searching).
The key idea of Prompt tuning is to inject task-specific embeddings into hidden layers and then use gradient-based optimization to adjust these embeddings. However, these methods require modifying the original inference process of the model and obtaining the model gradient, which is impractical in black-box LLM services like GPT-3 and ChatGPT. Furthermore, hint tuning introduces additional computational and storage costs, which are generally expensive for LLM.
A more feasible and efficient approach is to optimize the prompts by searching the original text space for approximate demonstration samples and sequences. Some work build prompts from the "Global view" or the "Local view". Global view-based methods usually optimize the different elements of the prompt as a whole to achieve better performance. For example, Diversity-guided [1] approaches exploit the overall diversity of demonstrations for search, or attempt to optimize the entire sample combination order [2] to achieve better performance. In contrast to the Global view, Local view-based methods work by designing different heuristic selection criteria, such as KATE [3].
But these methods have their own limitations: (1) Most current research mainly focuses on searching for cues along a single factor, such as example selection or order. However, the overall impact of each factor on performance is unclear. (2) These methods are usually based on heuristic criteria and require a unified perspective to explain how these methods work. (3) More importantly, existing methods optimize hints globally or locally, which may lead to unsatisfactory performance.
This article re-examines the prompt optimization problem in the field of NLP from the perspective of "prediction bias" and discovers a key phenomenon: the quality of a given prompt depends on its inherent bias. Based on this phenomenon, the article proposes an alternative criterion to evaluate the quality of prompts based on prediction bias. This metric can evaluate prompts through a single forward process without the need for an additional development set.
Specifically, by inputting a "no content" test under a given prompt, the model is expected to output a uniform prediction distribution (a "no content" input does not contain any useful information) . Therefore, the uniformity of the prediction distribution is used in this paper to represent the prediction deviation of a given prompt. This is similar to the metric used by the previous post-calibration method [4], but unlike post-calibration which uses this metric for probabilistic post-calibration under a fixed prompt, this paper further explores its application in automatically searching for approximate prompts. And through extensive experiments, we confirmed the correlation between the inherent bias of a given prompt and its average task performance on a given test set.
Furthermore, this bias-based metric enables the method to search for suitable prompts in a “local-to-global” manner. However, a realistic problem is that it is not possible to search for the optimal solution by traversing all combinations because its complexity would exceed O (N!).
This work proposes two novel strategies to search for high-quality prompts in an efficient manner: (1) T-fair-Prompting (2) G-fair-Prompting. T-fair-Prompting uses an intuitive approach, first calculating the deviation of each example individually forming a prompt, and then selecting the Top-k fairest examples to combine into the final prompt. This strategy is quite efficient, with complexity O (N). But it should be noted that T-fair-Prompting is based on the assumption that the optimal prompt is usually constructed from the least biased examples. However, this may not hold true in practical situations and often leads to local optimal solutions. Therefore, G-fair-Prompting is further introduced in the article to improve search quality. G-fair-Prompting follows the regular process of greedy search to find the optimal solution by making local optimal choices at each step. At each step of the algorithm, examples are selected such that the updated prompt achieves optimal fairness with a worst-case time complexity of O (N^2), significantly improving search quality. G-fair-Prompting works from a local-to-global perspective, where the bias of individual samples is considered in the early stages, while the later stages focus on reducing the global prediction bias.
Experimental results
This study proposes an effective and interpretable method to improve the context learning performance of language models, which can be applied to various downstream tasks. The article verifies the effectiveness of these two strategies on various LLMs (including the GPT series of models and the recently released LMaMA series). Compared with the SOTA method, G-fair-Prompting achieved more than 10% on different downstream tasks. relative improvement.
The closest thing to this research is the Calibration-before-use [4] method, both of which use “content-free” input to improve model performance. However, the Calibration-before-use method is designed to use this standard to calibrate the output, which is still susceptible to the quality of the examples used. In contrast, this paper aims to search the original space to find a near-optimal prompt to improve the performance of the model without any post-processing of the model output. Furthermore, this paper is the first to demonstrate through extensive experiments the link between prediction bias and final task performance, which has not yet been studied in calibration-before-use methods.
Through experiments, it can also be found that even without calibration, the prompt selected by the method proposed in this article can be better than the calibrated randomly selected prompt. This shows that the method can be practical and effective in practical applications and can provide inspiration for future natural language processing research.
The above is the detailed content of Re-examining the Prompt optimization problem, prediction bias makes language model context learning stronger. For more information, please follow other related articles on the PHP Chinese website!

Hot AI Tools

Undresser.AI Undress
AI-powered app for creating realistic nude photos

AI Clothes Remover
Online AI tool for removing clothes from photos.

Undress AI Tool
Undress images for free

Clothoff.io
AI clothes remover

Video Face Swap
Swap faces in any video effortlessly with our completely free AI face swap tool!

Hot Article

Hot Tools

Notepad++7.3.1
Easy-to-use and free code editor

SublimeText3 Chinese version
Chinese version, very easy to use

Zend Studio 13.0.1
Powerful PHP integrated development environment

Dreamweaver CS6
Visual web development tools

SublimeText3 Mac version
God-level code editing software (SublimeText3)

Hot Topics
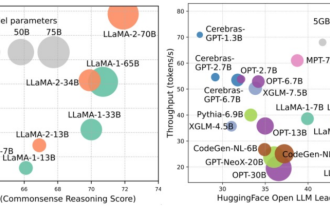
Large-scale language models (LLMs) have demonstrated compelling capabilities in many important tasks, including natural language understanding, language generation, and complex reasoning, and have had a profound impact on society. However, these outstanding capabilities require significant training resources (shown in the left image) and long inference times (shown in the right image). Therefore, researchers need to develop effective technical means to solve their efficiency problems. In addition, as can be seen from the right side of the figure, some efficient LLMs (LanguageModels) such as Mistral-7B have been successfully used in the design and deployment of LLMs. These efficient LLMs can significantly reduce inference memory while maintaining similar accuracy to LLaMA1-33B
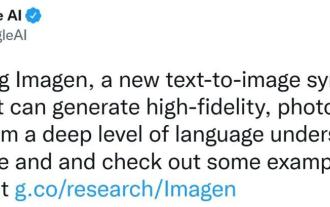
In recent years, multimodal learning has received much attention, especially in the two directions of text-image synthesis and image-text contrastive learning. Some AI models have attracted widespread public attention due to their application in creative image generation and editing, such as the text image models DALL・E and DALL-E 2 launched by OpenAI, and NVIDIA's GauGAN and GauGAN2. Not to be outdone, Google released its own text-to-image model Imagen at the end of May, which seems to further expand the boundaries of caption-conditional image generation. Given just a description of a scene, Imagen can generate high-quality, high-resolution
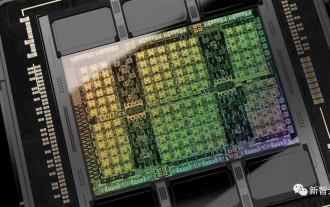
3nm process, performance surpasses H100! Recently, foreign media DigiTimes broke the news that Nvidia is developing the next-generation GPU, the B100, code-named "Blackwell". It is said that as a product for artificial intelligence (AI) and high-performance computing (HPC) applications, the B100 will use TSMC's 3nm process process, as well as more complex multi-chip module (MCM) design, and will appear in the fourth quarter of 2024. For Nvidia, which monopolizes more than 80% of the artificial intelligence GPU market, it can use the B100 to strike while the iron is hot and further attack challengers such as AMD and Intel in this wave of AI deployment. According to NVIDIA estimates, by 2027, the output value of this field is expected to reach approximately
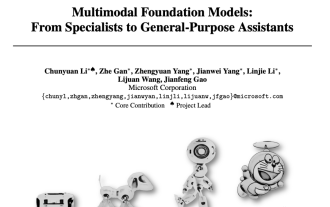
The most comprehensive review of multimodal large models is here! Written by 7 Chinese researchers at Microsoft, it has 119 pages. It starts from two types of multi-modal large model research directions that have been completed and are still at the forefront, and comprehensively summarizes five specific research topics: visual understanding and visual generation. The multi-modal large-model multi-modal agent supported by the unified visual model LLM focuses on a phenomenon: the multi-modal basic model has moved from specialized to universal. Ps. This is why the author directly drew an image of Doraemon at the beginning of the paper. Who should read this review (report)? In the original words of Microsoft: As long as you are interested in learning the basic knowledge and latest progress of multi-modal basic models, whether you are a professional researcher or a student, this content is very suitable for you to come together.
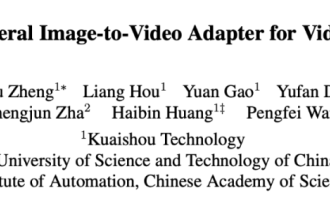
The image-to-video generation (I2V) task is a challenge in the field of computer vision that aims to convert static images into dynamic videos. The difficulty of this task is to extract and generate dynamic information in the temporal dimension from a single image while maintaining the authenticity and visual coherence of the image content. Existing I2V methods often require complex model architectures and large amounts of training data to achieve this goal. Recently, a new research result "I2V-Adapter: AGeneralImage-to-VideoAdapter for VideoDiffusionModels" led by Kuaishou was released. This research introduces an innovative image-to-video conversion method and proposes a lightweight adapter module, i.e.
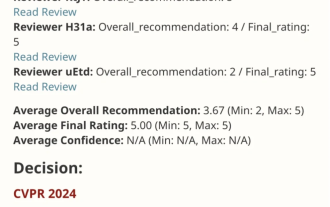
This work of EfficientSAM was included in CVPR2024 with a perfect score of 5/5/5! The author shared the result on a social media, as shown in the picture below: The LeCun Turing Award winner also strongly recommended this work! In recent research, Meta researchers have proposed a new improved method, namely mask image pre-training (SAMI) using SAM. This method combines MAE pre-training technology and SAM models to achieve high-quality pre-trained ViT encoders. Through SAMI, researchers try to improve the performance and efficiency of the model and provide better solutions for vision tasks. The proposal of this method brings new ideas and opportunities to further explore and develop the fields of computer vision and deep learning. by combining different
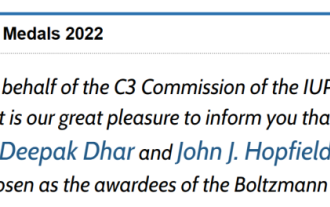
The two scientists who have won the 2022 Boltzmann Prize have been announced. This award was established by the IUPAP Committee on Statistical Physics (C3) to recognize researchers who have made outstanding achievements in the field of statistical physics. The winner must be a scientist who has not previously won a Boltzmann Prize or a Nobel Prize. This award began in 1975 and is awarded every three years in memory of Ludwig Boltzmann, the founder of statistical physics. Deepak Dharistheoriginalstatement. Reason for award: In recognition of Deepak Dharistheoriginalstatement's pioneering contributions to the field of statistical physics, including Exact solution of self-organized critical model, interface growth, disorder
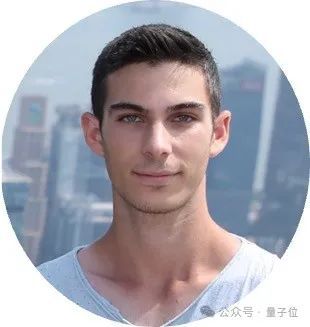
Video generation is progressing in full swing, and Pika has welcomed a great general - Google researcher Omer Bar-Tal, who serves as Pika's founding scientist. A month ago, Google released the video generation model Lumiere as a co-author, and the effect was amazing. At that time, netizens said: Google joins the video generation battle, and there is another good show to watch. Some people in the industry, including StabilityAI CEO and former colleagues from Google, sent their blessings. Lumiere's first work, Omer Bar-Tal, who just graduated with a master's degree, graduated from the Department of Mathematics and Computer Science at Tel Aviv University in 2021, and then went to the Weizmann Institute of Science to study for a master's degree in computer science, mainly focusing on research in the field of image and video synthesis. His thesis results have been published many times
