


Detailed explanation of three methods of parsing parameters in Python
This article brings you relevant knowledge about Python, which mainly organizes issues related to three methods of parsing parameters. The first option is to use argparse, which is a popular Python module, specifically used for command line parsing; another method is to read a JSON file, where we can place all hyperparameters; the third and little-known method is to use a YAML file. Let’s take a look at it together. I hope it will help Everyone is helpful.
[Related recommendations: Python3 video tutorial ]
The main purpose of what we share today is to use the command line and Configuration files to improve code efficiency
Let's go!
We use the parameter adjustment process in machine learning to practice. There are three ways to choose from. The first option is to use argparse, which is a popular Python module dedicated to command line parsing; the other is to read a JSON file where we can put all the hyperparameters; the third is also less known The solution is to use YAML files! Curious, let’s get started!
Prerequisites
In the code below, I will use Visual Studio Code, which is a very efficient integrated Python development environment. The beauty of this tool is that it supports every programming language by installing extensions, integrates the terminal and allows working with a large number of Python scripts and Jupyter notebooks
datasets, using the Shared Bike Dataset on Kaggle
Using argparse
As shown in the picture above, we have a standard structure to organize our small project:
- Contains our data Set a folder named data
- train.py file
- options.py file for specifying hyperparameters
First, we can create a file train.py, in which we have the basic procedure to import the data, train the model on the training data and evaluate it on the test set:
import pandas as pd import numpy as np from sklearn.ensemble import RandomForestRegressor from sklearn.model_selection import train_test_split from sklearn.preprocessing import StandardScaler from sklearn.metrics import mean_squared_error, mean_absolute_error from options import train_options df = pd.read_csv('data\hour.csv') print(df.head()) opt = train_options() X=df.drop(['instant','dteday','atemp','casual','registered','cnt'],axis=1).values y =df['cnt'].values X_train, X_test, y_train, y_test = train_test_split(X, y, test_size=0.2) if opt.normalize == True: scaler = StandardScaler() X = scaler.fit_transform(X) rf = RandomForestRegressor(n_estimators=opt.n_estimators,max_features=opt.max_features,max_depth=opt.max_depth) model = rf.fit(X_train,y_train) y_pred = model.predict(X_test) rmse = np.sqrt(mean_squared_error(y_pred, y_test)) mae = mean_absolute_error(y_pred, y_test) print("rmse: ",rmse) print("mae: ",mae)
In the code, we also import the files contained in options.py train_options function in the file. The latter file is a Python file from which we can change the hyperparameters considered in train.py:
import argparse def train_options(): parser = argparse.ArgumentParser() parser.add_argument("--normalize", default=True, type=bool, help='maximum depth') parser.add_argument("--n_estimators", default=100, type=int, help='number of estimators') parser.add_argument("--max_features", default=6, type=int, help='maximum of features',) parser.add_argument("--max_depth", default=5, type=int,help='maximum depth') opt = parser.parse_args() return opt
In this example, we use the argparse library, which is very popular when parsing command line arguments. First, we initialize the parser, then, we can add the parameters we want to access.
Here is an example of running code:
python train.py
To change the default values of hyperparameters, there are two ways. The first option is to set different default values in the options.py file. Another option is to pass the hyperparameter value from the command line:
python train.py --n_estimators 200
We need to specify the name of the hyperparameter we want to change and the corresponding value.
python train.py --n_estimators 200 --max_depth 7
Using JSON files
As before, we can keep a similar file structure. In this case, we replace the options.py file with a JSON file. In other words, we want to specify the values of the hyperparameters in a JSON file and pass them to the train.py file. JSON files can be a fast and intuitive alternative to the argparse library, leveraging key-value pairs to store data. Next we create an options.json file that contains the data we need to pass to other code later.
{ "normalize":true, "n_estimators":100, "max_features":6, "max_depth":5 }
As you can see above, it is very similar to a Python dictionary. But unlike a dictionary, it contains data in text/string format. Additionally, there are some common data types with slightly different syntax. For example, Boolean values are false/true, while Python recognizes False/True. Other possible values in JSON are arrays, which are represented as Python lists using square brackets.
The beauty of working with JSON data in Python is that it can be converted into a Python dictionary via the load method:
f = open("options.json", "rb") parameters = json.load(f)
To access a specific item, we just need to quote it within square brackets Key name:
if parameters["normalize"] == True: scaler = StandardScaler() X = scaler.fit_transform(X) rf=RandomForestRegressor(n_estimators=parameters["n_estimators"],max_features=parameters["max_features"],max_depth=parameters["max_depth"],random_state=42) model = rf.fit(X_train,y_train) y_pred = model.predict(X_test)
Using YAML files
The last option is to take advantage of the potential of YAML. As with JSON files, we read the YAML file in Python code as a dictionary to access the values of the hyperparameters. YAML is a human-readable data representation language in which hierarchies are represented using double-space characters instead of parentheses like in JSON files. Below we show what the options.yaml file will contain:
normalize: True n_estimators: 100 max_features: 6 max_depth: 5
In train.py, we open the options.yaml file, which will always be converted to a Python dictionary using the load method, this time from the yaml library Imported in:
import yaml f = open('options.yaml','rb') parameters = yaml.load(f, Loader=yaml.FullLoader)
As before, we can access the value of the hyperparameter using the syntax required by the dictionary.
Final Thoughts
Configuration files compile very quickly, whereas argparse requires writing a line of code for each argument we want to add.
So we should choose the most appropriate method according to our different situations
For example, if we need to add comments to parameters, JSON is not suitable because it does not allow comments, and YAML and argparse might be a good fit.
【Related recommendations: Python3 video tutorial】
The above is the detailed content of Detailed explanation of three methods of parsing parameters in Python. For more information, please follow other related articles on the PHP Chinese website!

Hot AI Tools

Undresser.AI Undress
AI-powered app for creating realistic nude photos

AI Clothes Remover
Online AI tool for removing clothes from photos.

Undress AI Tool
Undress images for free

Clothoff.io
AI clothes remover

Video Face Swap
Swap faces in any video effortlessly with our completely free AI face swap tool!

Hot Article

Hot Tools

Notepad++7.3.1
Easy-to-use and free code editor

SublimeText3 Chinese version
Chinese version, very easy to use

Zend Studio 13.0.1
Powerful PHP integrated development environment

Dreamweaver CS6
Visual web development tools

SublimeText3 Mac version
God-level code editing software (SublimeText3)

Hot Topics
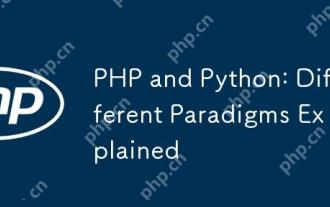
PHP is mainly procedural programming, but also supports object-oriented programming (OOP); Python supports a variety of paradigms, including OOP, functional and procedural programming. PHP is suitable for web development, and Python is suitable for a variety of applications such as data analysis and machine learning.
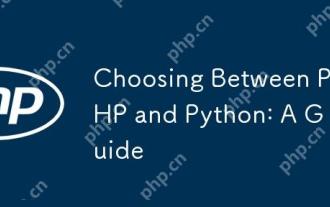
PHP is suitable for web development and rapid prototyping, and Python is suitable for data science and machine learning. 1.PHP is used for dynamic web development, with simple syntax and suitable for rapid development. 2. Python has concise syntax, is suitable for multiple fields, and has a strong library ecosystem.
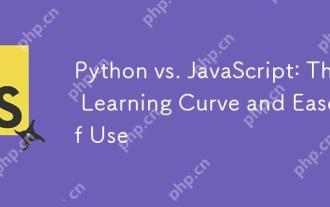
Python is more suitable for beginners, with a smooth learning curve and concise syntax; JavaScript is suitable for front-end development, with a steep learning curve and flexible syntax. 1. Python syntax is intuitive and suitable for data science and back-end development. 2. JavaScript is flexible and widely used in front-end and server-side programming.
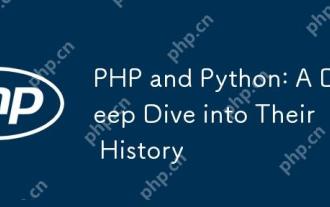
PHP originated in 1994 and was developed by RasmusLerdorf. It was originally used to track website visitors and gradually evolved into a server-side scripting language and was widely used in web development. Python was developed by Guidovan Rossum in the late 1980s and was first released in 1991. It emphasizes code readability and simplicity, and is suitable for scientific computing, data analysis and other fields.
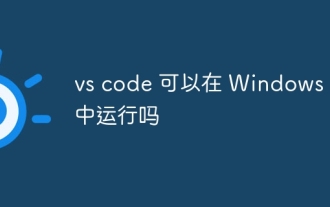
VS Code can run on Windows 8, but the experience may not be great. First make sure the system has been updated to the latest patch, then download the VS Code installation package that matches the system architecture and install it as prompted. After installation, be aware that some extensions may be incompatible with Windows 8 and need to look for alternative extensions or use newer Windows systems in a virtual machine. Install the necessary extensions to check whether they work properly. Although VS Code is feasible on Windows 8, it is recommended to upgrade to a newer Windows system for a better development experience and security.
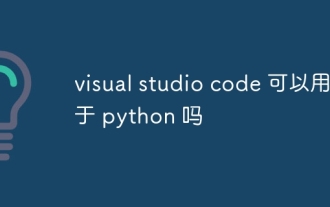
VS Code can be used to write Python and provides many features that make it an ideal tool for developing Python applications. It allows users to: install Python extensions to get functions such as code completion, syntax highlighting, and debugging. Use the debugger to track code step by step, find and fix errors. Integrate Git for version control. Use code formatting tools to maintain code consistency. Use the Linting tool to spot potential problems ahead of time.
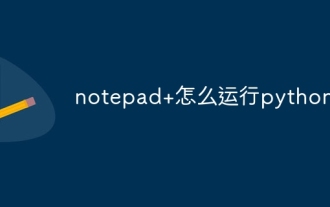
Running Python code in Notepad requires the Python executable and NppExec plug-in to be installed. After installing Python and adding PATH to it, configure the command "python" and the parameter "{CURRENT_DIRECTORY}{FILE_NAME}" in the NppExec plug-in to run Python code in Notepad through the shortcut key "F6".
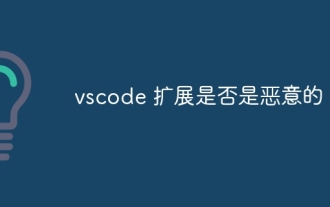
VS Code extensions pose malicious risks, such as hiding malicious code, exploiting vulnerabilities, and masturbating as legitimate extensions. Methods to identify malicious extensions include: checking publishers, reading comments, checking code, and installing with caution. Security measures also include: security awareness, good habits, regular updates and antivirus software.
