What is the process of sorting performed by the system called?
MapReduce ensures that the input of each reducer is sorted by key, and the system performs the sorting process called shuffle. The shuffle phase mainly includes combine, group, sort, partition in the map phase and merge sorting in the reducer phase.
The operating environment of this tutorial: Windows 7 system, Dell G3 computer.
MapReduce ensures that the input of each reducer is sorted by key, and the system performs the sorting process called shuffle. We can understand it as the entire project where map generates output and digests input to reduce.
Map side: Each mapperTask has a ring memory buffer used to store the output of the map task. Once the threshold is reached, a background thread writes the content to a new overflow write file in the specified directory on the disk. , partition, sort, and combiner must be passed before writing to disk. After the last record is written, merge all overflow written files into one partitioned and sorted file.
Reduce side: can be divided into copy phase, sorting phase, reduce phase
Copy phase: The map output file is located on the local disk of the tasktracker running the map task, and reduce obtains the output through http For the partition of the file, tasktracker runs the reduce task for the partition file. As long as a map task is completed, the reduce task starts to copy the output.
Sorting phase: A more appropriate term is the merging phase, because sorting is performed on the map side. This stage will merge the map output, maintain its order, and loop.
The final stage is the reduce stage. The reduce function is called for each key in the sorted output. The output of this stage is written directly to the output file system, usually HDFS. ,
Shuffle phase description
The shuffle phase mainly includes combine, group, sort, partition in the map phase and merge sorting in the reducer phase. After shuffling in the Map stage, the output data will be saved in files according to the reduce partitions, and the file contents will be sorted according to the defined sort. After the Map phase is completed, the ApplicationMaster will be notified, and then the AM will notify the Reduce to pull the data, and perform the shuffle process on the reduce side during the pulling process.
Note: The output data of the Map stage is stored on the disk running the Map node. It is a temporary file and does not exist on HDFS. After Reduce pulls the data, the temporary file will be deleted. If it exists on HDFS, it will be deleted. This causes a waste of storage space (three copies will be generated).
-
User-defined Combiner
Combiner can reduce the number of intermediate output results in the Map stage and reduce network overhead. By default, there is no Combiner. The user-defined Combiner is required to be a subclass of Reducer. The output
of the Map is used as the input and output of the Combiner. That is to say, the input and output of the Combiner must it's the same. You can set the combiner processing class through job.setCombinerClass. The MapReduce framework does not guarantee that the method of this class will be called.
Note: If the input and output of reduce are the same, you can directly use the reduce class as combiner
-
User-defined Partitioner
Partitioner is used Determine which node is the processing reducer corresponding to the
output by the map. The default MapReduce task reduce number is 1. At this time, the Partitioner actually has no effect. However, when we change the number of reduce to multiple, the Partitioner will determine the node number of the reduce corresponding to the key (starting from 0). You can specify the Partitioner class through the job.setPartitionerClass method. By default, HashPartitioner is used (the key's hashCode method is called by default).
-
User-defined Group
GroupingComparator is used to group the
output by Map into > The key class, to put it bluntly, is used to determine whether key1 and key2 belong to the same group. If they are the same group, the output values of the map are combined. Our custom class is required to implement the self-interface RawComparator, and the comparison class can be specified through the job.setGroupingComparatorClass method. By default a WritableComparator is used, but the key's compareTo method is ultimately called for comparison.
-
User-defined Sort
SortComparator is the key class used to key sort the
output by Map. To put it bluntly, it is used for Determine which group key1 belongs to and which group key2 belongs to comes first and which one comes last. Our custom class is required to implement the self-interface RawComparator, and the comparison class can be specified through the job.setSortComparatorClass method. By default a WritableComparator is used, but the key's compareTo method is ultimately called for comparison.
-
User-defined Reducer's Shuffle
When the reduce side pulls the output data of the map, shuffle (merge sort) will be performed, and the MapReduce framework is provided in plug-in mode In a customized way, we can specify custom shuffle rules by implementing the interface ShuffleConsumerPlugin and specifying the parameter mapreduce.job.reduce.shuffle.consumer.plugin.class, but in general, the default class org is used directly. apache.hadoop.mapreduce.task.reduce.Shuffle.
For more programming-related knowledge, please visit: Programming Video! !
The above is the detailed content of What is the process of sorting performed by the system called?. For more information, please follow other related articles on the PHP Chinese website!

Hot AI Tools

Undresser.AI Undress
AI-powered app for creating realistic nude photos

AI Clothes Remover
Online AI tool for removing clothes from photos.

Undress AI Tool
Undress images for free

Clothoff.io
AI clothes remover

Video Face Swap
Swap faces in any video effortlessly with our completely free AI face swap tool!

Hot Article

Hot Tools

Notepad++7.3.1
Easy-to-use and free code editor

SublimeText3 Chinese version
Chinese version, very easy to use

Zend Studio 13.0.1
Powerful PHP integrated development environment

Dreamweaver CS6
Visual web development tools

SublimeText3 Mac version
God-level code editing software (SublimeText3)

Hot Topics
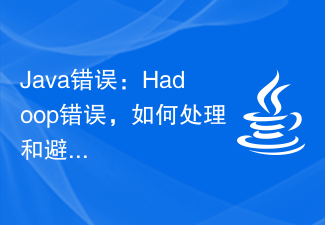
Java Errors: Hadoop Errors, How to Handle and Avoid When using Hadoop to process big data, you often encounter some Java exception errors, which may affect the execution of tasks and cause data processing to fail. This article will introduce some common Hadoop errors and provide ways to deal with and avoid them. Java.lang.OutOfMemoryErrorOutOfMemoryError is an error caused by insufficient memory of the Java virtual machine. When Hadoop is
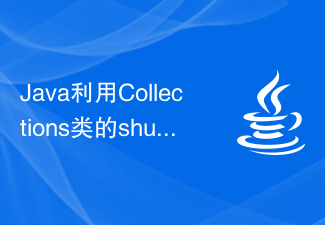
Java uses the shuffle() function of the Collections class to disrupt the order of elements in the collection. In the Java programming language, the Collections class is a tool class that provides various static methods for operating collections. One of them is the shuffle() function, which can be used to shuffle the order of elements in a collection. This article demonstrates how to use this function and provides corresponding code examples. First, we need to import the Collections class in the java.util package,
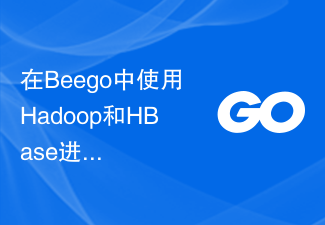
With the advent of the big data era, data processing and storage have become more and more important, and how to efficiently manage and analyze large amounts of data has become a challenge for enterprises. Hadoop and HBase, two projects of the Apache Foundation, provide a solution for big data storage and analysis. This article will introduce how to use Hadoop and HBase in Beego for big data storage and query. 1. Introduction to Hadoop and HBase Hadoop is an open source distributed storage and computing system that can
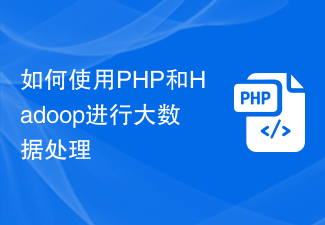
As the amount of data continues to increase, traditional data processing methods can no longer handle the challenges brought by the big data era. Hadoop is an open source distributed computing framework that solves the performance bottleneck problem caused by single-node servers in big data processing through distributed storage and processing of large amounts of data. PHP is a scripting language that is widely used in web development and has the advantages of rapid development and easy maintenance. This article will introduce how to use PHP and Hadoop for big data processing. What is HadoopHadoop is
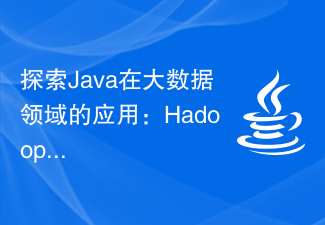
Java big data technology stack: Understand the application of Java in the field of big data, such as Hadoop, Spark, Kafka, etc. As the amount of data continues to increase, big data technology has become a hot topic in today's Internet era. In the field of big data, we often hear the names of Hadoop, Spark, Kafka and other technologies. These technologies play a vital role, and Java, as a widely used programming language, also plays a huge role in the field of big data. This article will focus on the application of Java in large
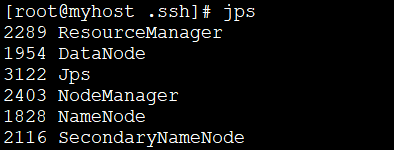
1: Install JDK1. Execute the following command to download the JDK1.8 installation package. wget--no-check-certificatehttps://repo.huaweicloud.com/java/jdk/8u151-b12/jdk-8u151-linux-x64.tar.gz2. Execute the following command to decompress the downloaded JDK1.8 installation package. tar-zxvfjdk-8u151-linux-x64.tar.gz3. Move and rename the JDK package. mvjdk1.8.0_151//usr/java84. Configure Java environment variables. echo'
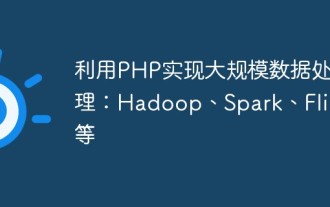
As the amount of data continues to increase, large-scale data processing has become a problem that enterprises must face and solve. Traditional relational databases can no longer meet this demand. For the storage and analysis of large-scale data, distributed computing platforms such as Hadoop, Spark, and Flink have become the best choices. In the selection process of data processing tools, PHP is becoming more and more popular among developers as a language that is easy to develop and maintain. In this article, we will explore how to leverage PHP for large-scale data processing and how
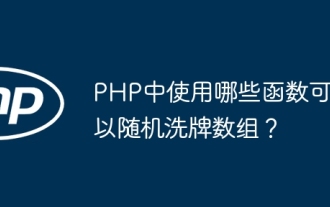
There are the following functions in PHP that can shuffle an array randomly: shuffle() directly changes the order of array elements. array_rand() returns a random key, which can rearrange the array order based on the key.