How python recognizes verification code
When python crawler crawls the verification code of some websites, you may encounter the problem of verification code recognition. Most of the current verification codes are divided into four categories: 1. Calculate verification code 2. Slider verification code 3. Recognition Picture verification code 4, voice verification code
The main purpose here is to identify the verification code. What is recognized is a simple verification code. If you want to make the recognition rate higher, the recognition will be more accurate. It takes a lot of effort to train your own font library.
Identification of verification codes usually involves these steps:
1. Grayscale processing
2. Binarization
3. Remove borders (if If yes)
4. Noise reduction
5. Character cutting or tilt correction
6. Training font library
7. Recognition
The first three steps of these 6 steps are basic. You can choose whether 4 or 5 is needed according to the actual situation. The verification code does not necessarily need to be cut. The recognition rate will increase a lot and sometimes it will decrease.
Several main python libraries used: Pillow (python image processing library), OpenCV (advanced image processing library), pytesseract (recognition library)
The following case Usage:
1. Put the verification code image to be recognized into the img folder at the same level as the script, and create the out_img folder
2. python3 filename
3. Images at various stages such as binarization and noise reduction will be stored in the out_img folder, and the final recognition result will be printed on the screen.
Complete QR code identification code:
from PIL import Image from pytesseract import * from fnmatch import fnmatch from queue import Queue import matplotlib.pyplot as plt import cv2 import time import os def clear_border(img,img_name): '''去除边框 ''' filename = './out_img/' + img_name.split('.')[0] + '-clearBorder.jpg' h, w = img.shape[:2] for y in range(0, w): for x in range(0, h): # if y ==0 or y == w -1 or y == w - 2: if y < 4 or y > w -4: img[x, y] = 255 # if x == 0 or x == h - 1 or x == h - 2: if x < 4 or x > h - 4: img[x, y] = 255 cv2.imwrite(filename,img) return img def interference_line(img, img_name): ''' 干扰线降噪 ''' filename = './out_img/' + img_name.split('.')[0] + '-interferenceline.jpg' h, w = img.shape[:2] # !!!opencv矩阵点是反的 # img[1,2] 1:图片的高度,2:图片的宽度 for y in range(1, w - 1): for x in range(1, h - 1): count = 0 if img[x, y - 1] > 245: count = count + 1 if img[x, y + 1] > 245: count = count + 1 if img[x - 1, y] > 245: count = count + 1 if img[x + 1, y] > 245: count = count + 1 if count > 2: img[x, y] = 255 cv2.imwrite(filename,img) return img def interference_point(img,img_name, x = 0, y = 0): """点降噪 9邻域框,以当前点为中心的田字框,黑点个数 :param x: :param y: :return: """ filename = './out_img/' + img_name.split('.')[0] + '-interferencePoint.jpg' # todo 判断图片的长宽度下限 cur_pixel = img[x,y]# 当前像素点的值 height,width = img.shape[:2] for y in range(0, width - 1): for x in range(0, height - 1): if y == 0: # 第一行 if x == 0: # 左上顶点,4邻域 # 中心点旁边3个点 sum = int(cur_pixel) \ + int(img[x, y + 1]) \ + int(img[x + 1, y]) \ + int(img[x + 1, y + 1]) if sum <= 2 * 245: img[x, y] = 0 elif x == height - 1: # 右上顶点 sum = int(cur_pixel) \ + int(img[x, y + 1]) \ + int(img[x - 1, y]) \ + int(img[x - 1, y + 1]) if sum <= 2 * 245: img[x, y] = 0 else: # 最上非顶点,6邻域 sum = int(img[x - 1, y]) \ + int(img[x - 1, y + 1]) \ + int(cur_pixel) \ + int(img[x, y + 1]) \ + int(img[x + 1, y]) \ + int(img[x + 1, y + 1]) if sum <= 3 * 245: img[x, y] = 0 elif y == width - 1: # 最下面一行 if x == 0: # 左下顶点 # 中心点旁边3个点 sum = int(cur_pixel) \ + int(img[x + 1, y]) \ + int(img[x + 1, y - 1]) \ + int(img[x, y - 1]) if sum <= 2 * 245: img[x, y] = 0 elif x == height - 1: # 右下顶点 sum = int(cur_pixel) \ + int(img[x, y - 1]) \ + int(img[x - 1, y]) \ + int(img[x - 1, y - 1]) if sum <= 2 * 245: img[x, y] = 0 else: # 最下非顶点,6邻域 sum = int(cur_pixel) \ + int(img[x - 1, y]) \ + int(img[x + 1, y]) \ + int(img[x, y - 1]) \ + int(img[x - 1, y - 1]) \ + int(img[x + 1, y - 1]) if sum <= 3 * 245: img[x, y] = 0 else: # y不在边界 if x == 0: # 左边非顶点 sum = int(img[x, y - 1]) \ + int(cur_pixel) \ + int(img[x, y + 1]) \ + int(img[x + 1, y - 1]) \ + int(img[x + 1, y]) \ + int(img[x + 1, y + 1]) if sum <= 3 * 245: img[x, y] = 0 elif x == height - 1: # 右边非顶点 sum = int(img[x, y - 1]) \ + int(cur_pixel) \ + int(img[x, y + 1]) \ + int(img[x - 1, y - 1]) \ + int(img[x - 1, y]) \ + int(img[x - 1, y + 1]) if sum <= 3 * 245: img[x, y] = 0 else: # 具备9领域条件的 sum = int(img[x - 1, y - 1]) \ + int(img[x - 1, y]) \ + int(img[x - 1, y + 1]) \ + int(img[x, y - 1]) \ + int(cur_pixel) \ + int(img[x, y + 1]) \ + int(img[x + 1, y - 1]) \ + int(img[x + 1, y]) \ + int(img[x + 1, y + 1]) if sum <= 4 * 245: img[x, y] = 0 cv2.imwrite(filename,img) return img def _get_dynamic_binary_image(filedir, img_name): ''' 自适应阀值二值化 ''' filename = './out_img/' + img_name.split('.')[0] + '-binary.jpg' img_name = filedir + '/' + img_name print('.....' + img_name) im = cv2.imread(img_name) im = cv2.cvtColor(im,cv2.COLOR_BGR2GRAY) th1 = cv2.adaptiveThreshold(im, 255, cv2.ADAPTIVE_THRESH_GAUSSIAN_C, cv2.THRESH_BINARY, 21, 1) cv2.imwrite(filename,th1) return th1 def _get_static_binary_image(img, threshold = 140): ''' 手动二值化 ''' img = Image.open(img) img = img.convert('L') pixdata = img.load() w, h = img.size for y in range(h): for x in range(w): if pixdata[x, y] < threshold: pixdata[x, y] = 0 else: pixdata[x, y] = 255 return img def cfs(im,x_fd,y_fd): '''用队列和集合记录遍历过的像素坐标代替单纯递归以解决cfs访问过深问题 ''' # print('**********') xaxis=[] yaxis=[] visited =set() q = Queue() q.put((x_fd, y_fd)) visited.add((x_fd, y_fd)) offsets=[(1, 0), (0, 1), (-1, 0), (0, -1)]#四邻域 while not q.empty(): x,y=q.get() for xoffset,yoffset in offsets: x_neighbor,y_neighbor = x+xoffset,y+yoffset if (x_neighbor,y_neighbor) in (visited): continue # 已经访问过了 visited.add((x_neighbor, y_neighbor)) try: if im[x_neighbor, y_neighbor] == 0: xaxis.append(x_neighbor) yaxis.append(y_neighbor) q.put((x_neighbor,y_neighbor)) except IndexError: pass # print(xaxis) if (len(xaxis) == 0 | len(yaxis) == 0): xmax = x_fd + 1 xmin = x_fd ymax = y_fd + 1 ymin = y_fd else: xmax = max(xaxis) xmin = min(xaxis) ymax = max(yaxis) ymin = min(yaxis) #ymin,ymax=sort(yaxis) return ymax,ymin,xmax,xmin def detectFgPix(im,xmax): '''搜索区块起点 ''' h,w = im.shape[:2] for y_fd in range(xmax+1,w): for x_fd in range(h): if im[x_fd,y_fd] == 0: return x_fd,y_fd def CFS(im): '''切割字符位置 ''' zoneL=[]#各区块长度L列表 zoneWB=[]#各区块的X轴[起始,终点]列表 zoneHB=[]#各区块的Y轴[起始,终点]列表 xmax=0#上一区块结束黑点横坐标,这里是初始化 for i in range(10): try: x_fd,y_fd = detectFgPix(im,xmax) # print(y_fd,x_fd) xmax,xmin,ymax,ymin=cfs(im,x_fd,y_fd) L = xmax - xmin H = ymax - ymin zoneL.append(L) zoneWB.append([xmin,xmax]) zoneHB.append([ymin,ymax]) except TypeError: return zoneL,zoneWB,zoneHB return zoneL,zoneWB,zoneHB def cutting_img(im,im_position,img,xoffset = 1,yoffset = 1): filename = './out_img/' + img.split('.')[0] # 识别出的字符个数 im_number = len(im_position[1]) # 切割字符 for i in range(im_number): im_start_X = im_position[1][i][0] - xoffset im_end_X = im_position[1][i][1] + xoffset im_start_Y = im_position[2][i][0] - yoffset im_end_Y = im_position[2][i][1] + yoffset cropped = im[im_start_Y:im_end_Y, im_start_X:im_end_X] cv2.imwrite(filename + '-cutting-' + str(i) + '.jpg',cropped) def main(): filedir = './easy_img' for file in os.listdir(filedir): if fnmatch(file, '*.jpeg'): img_name = file # 自适应阈值二值化 im = _get_dynamic_binary_image(filedir, img_name) # 去除边框 im = clear_border(im,img_name) # 对图片进行干扰线降噪 im = interference_line(im,img_name) # 对图片进行点降噪 im = interference_point(im,img_name) # 切割的位置 im_position = CFS(im) maxL = max(im_position[0]) minL = min(im_position[0]) # 如果有粘连字符,如果一个字符的长度过长就认为是粘连字符,并从中间进行切割 if(maxL > minL + minL * 0.7): maxL_index = im_position[0].index(maxL) minL_index = im_position[0].index(minL) # 设置字符的宽度 im_position[0][maxL_index] = maxL // 2 im_position[0].insert(maxL_index + 1, maxL // 2) # 设置字符X轴[起始,终点]位置 im_position[1][maxL_index][1] = im_position[1][maxL_index][0] + maxL // 2 im_position[1].insert(maxL_index + 1, [im_position[1][maxL_index][1] + 1, im_position[1][maxL_index][1] + 1 + maxL // 2]) # 设置字符的Y轴[起始,终点]位置 im_position[2].insert(maxL_index + 1, im_position[2][maxL_index]) # 切割字符,要想切得好就得配置参数,通常 1 or 2 就可以 cutting_img(im,im_position,img_name,1,1) # 识别验证码 cutting_img_num = 0 for file in os.listdir('./out_img'): str_img = '' if fnmatch(file, '%s-cutting-*.jpg' % img_name.split('.')[0]): cutting_img_num += 1 for i in range(cutting_img_num): try: file = './out_img/%s-cutting-%s.jpg' % (img_name.split('.')[0], i) # 识别验证码 str_img = str_img + image_to_string(Image.open(file),lang = 'eng', config='-psm 10') #单个字符是10,一行文本是7 except Exception as err: pass print('切图:%s' % cutting_img_num) print('识别为:%s' % str_img) if __name__ == '__main__': main()
The above is the detailed content of How python recognizes verification code. For more information, please follow other related articles on the PHP Chinese website!

Hot AI Tools

Undresser.AI Undress
AI-powered app for creating realistic nude photos

AI Clothes Remover
Online AI tool for removing clothes from photos.

Undress AI Tool
Undress images for free

Clothoff.io
AI clothes remover

Video Face Swap
Swap faces in any video effortlessly with our completely free AI face swap tool!

Hot Article

Hot Tools

Notepad++7.3.1
Easy-to-use and free code editor

SublimeText3 Chinese version
Chinese version, very easy to use

Zend Studio 13.0.1
Powerful PHP integrated development environment

Dreamweaver CS6
Visual web development tools

SublimeText3 Mac version
God-level code editing software (SublimeText3)

Hot Topics
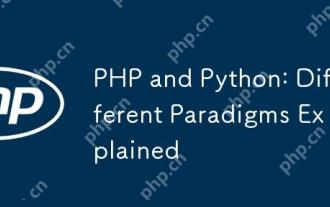
PHP is mainly procedural programming, but also supports object-oriented programming (OOP); Python supports a variety of paradigms, including OOP, functional and procedural programming. PHP is suitable for web development, and Python is suitable for a variety of applications such as data analysis and machine learning.
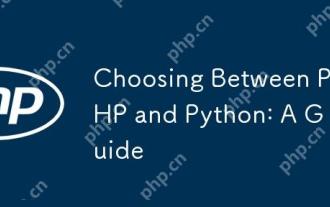
PHP is suitable for web development and rapid prototyping, and Python is suitable for data science and machine learning. 1.PHP is used for dynamic web development, with simple syntax and suitable for rapid development. 2. Python has concise syntax, is suitable for multiple fields, and has a strong library ecosystem.
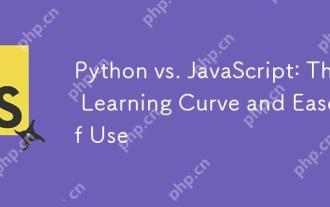
Python is more suitable for beginners, with a smooth learning curve and concise syntax; JavaScript is suitable for front-end development, with a steep learning curve and flexible syntax. 1. Python syntax is intuitive and suitable for data science and back-end development. 2. JavaScript is flexible and widely used in front-end and server-side programming.
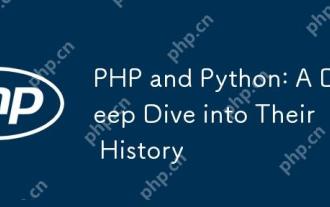
PHP originated in 1994 and was developed by RasmusLerdorf. It was originally used to track website visitors and gradually evolved into a server-side scripting language and was widely used in web development. Python was developed by Guidovan Rossum in the late 1980s and was first released in 1991. It emphasizes code readability and simplicity, and is suitable for scientific computing, data analysis and other fields.
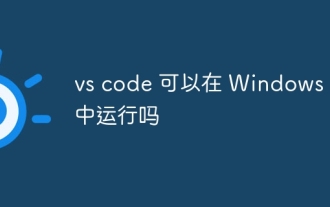
VS Code can run on Windows 8, but the experience may not be great. First make sure the system has been updated to the latest patch, then download the VS Code installation package that matches the system architecture and install it as prompted. After installation, be aware that some extensions may be incompatible with Windows 8 and need to look for alternative extensions or use newer Windows systems in a virtual machine. Install the necessary extensions to check whether they work properly. Although VS Code is feasible on Windows 8, it is recommended to upgrade to a newer Windows system for a better development experience and security.
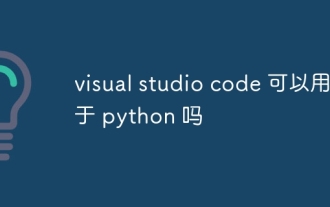
VS Code can be used to write Python and provides many features that make it an ideal tool for developing Python applications. It allows users to: install Python extensions to get functions such as code completion, syntax highlighting, and debugging. Use the debugger to track code step by step, find and fix errors. Integrate Git for version control. Use code formatting tools to maintain code consistency. Use the Linting tool to spot potential problems ahead of time.
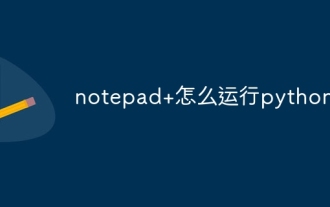
Running Python code in Notepad requires the Python executable and NppExec plug-in to be installed. After installing Python and adding PATH to it, configure the command "python" and the parameter "{CURRENT_DIRECTORY}{FILE_NAME}" in the NppExec plug-in to run Python code in Notepad through the shortcut key "F6".
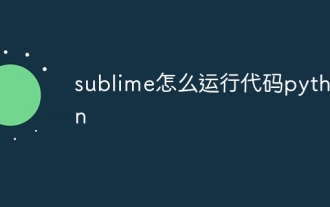
To run Python code in Sublime Text, you need to install the Python plug-in first, then create a .py file and write the code, and finally press Ctrl B to run the code, and the output will be displayed in the console.
