Python uses OpenCV for calibration
This article mainly introduces the use of OpenCV for calibration of Python. It has a certain reference value. Now I share it with you. Friends in need can refer to it.
This article combines the official OpenCV samples and provides an introduction to the official samples. Modify the code in the example so that it can run normally, and conduct experiments and explanations on the data you collected.
1. Preparation
OpenCV uses a checkerboard for calibration, as shown in the figure below. In order to calibrate the camera, we need to input a series of 3D points and their corresponding 2D image points. On a black and white checkerboard, two-dimensional image points are easily found through corner detection. What about three-dimensional points in the real world? During our collection, we placed the camera in one place, moved the checkerboard calibration plate to different positions, and then photographed it. So we need to know the value of (X,Y,Z). But to put it simply, we define the plane where the checkerboard is located as the XY plane, that is, Z=0. For the calibration board, we can know the square size of the checkerboard, such as 30mm, so we can define the corner point coordinates on the checkerboard as (0,0,0), (30,0,0), ( 60,0,0),..., the unit of this result is mm.
3D points are called object points, and 2D image points are called image points.
2. Detect checkerboard corner points
In order to find the checkerboard template, we use openCV The function cv2.findChessboardCorners(). We also need to tell the program what specifications the template we are using is, such as an 8*8 checkerboard or a 5*5 checkerboard. It is recommended to use a checkerboard template with unequal numbers in the x and y directions. In the following experiment, we use a 10*7 checkerboard. Each square has a side length of 20mm, which means it contains 9*6 internal corners. If this function detects the template, it will return the corresponding corner point and return true. Of course, not all images can find the required template, so we can use multiple images for calibration. In addition to using a checkerboard, we can also use a dot matrix, and the corresponding function is cv2.findCirclesGrid().
After finding the corner point, we can use cv2.cornerSubPix() to get more accurate corner pixel coordinates. We can also use cv2.drawChessboardCorners() to draw the corners onto the image for display. As shown in the figure below:
3. Calibration
Through the above steps, we The three-dimensional points used for calibration and the corresponding two-dimensional point pairs on the image are obtained. We use cv2.calibrateCamera() for calibration. This function returns the calibration result, the camera's intrinsic parameter matrix, distortion coefficient, rotation matrix and translation vector.
4. De-distortion
#In the third step, we have obtained the camera intrinsic parameters and distortion coefficients. Before de-distorting the image, we also You can use cv.getOptimalNewCameraMatrix() to optimize the internal parameters and distortion coefficients by setting the free scale factor alpha. When alpha is set to 0, a trimmed inner parameter and distortion coefficient that removes unwanted pixels after dedistortion will be returned; when alpha is set to 1, a trimmed inner parameter containing extra black pixels will be returned. parameters and distortion coefficients, and returns an ROI for cropping it out.
Then we can use the newly obtained internal parameter matrix and distortion coefficient to dedistort the image. There are two ways to remove distortion:
(1) Use cv2.undistort()
This is the most direct method, just call the function directly Obtain the dedistorted image, which can be cropped using the ROI above. The code is as follows:
# undistort dst = cv2.undistort(img, mtx, dist, None, newcameramtx) # crop the image x,y,w,h = roi dst = dst[y:y+h, x:x+w] cv2.imwrite('calibresult.png',dst)
The following picture shows the result of de-distorting a picture and retaining black pixels:
(2) Use remmaping
This is a two-step method, first calculate a mapping from the distorted image to the non-distorted image, and then use this mapping The relationship is used to dedistort the image.
The code is as follows:
# undistort mapx,mapy = cv2.initUndistortRectifyMap(mtx,dist,None,newcameramtx,(w,h),5) dst = cv2.remap(img,mapx,mapy,cv2.INTER_LINEAR) # crop the image x,y,w,h = roi dst = dst[y:y+h, x:x+w] cv2.imwrite('calibresult.png',dst)
5. Back-projection error
Through the reverse projection Projection error, we can evaluate the quality of the results. The closer to 0, the more ideal the result is. Using the previously calculated internal parameter matrix, distortion coefficient, rotation matrix and translation vector, use cv2.projectPoints() to calculate the projection of the three-dimensional point to the two-dimensional image, and then calculate the error between the point obtained by back-projection and the point detected on the image, Finally, calculate an average error for all calibration images, and this value is the back-projection error.
Code
The code for all steps is as follows:
#coding:utf-8 import cv2 import numpy as np import glob # 找棋盘格角点 # 阈值 criteria = (cv2.TERM_CRITERIA_EPS + cv2.TERM_CRITERIA_MAX_ITER, 30, 0.001) #棋盘格模板规格 w = 9 h = 6 # 世界坐标系中的棋盘格点,例如(0,0,0), (1,0,0), (2,0,0) ....,(8,5,0),去掉Z坐标,记为二维矩阵 objp = np.zeros((w*h,3), np.float32) objp[:,:2] = np.mgrid[0:w,0:h].T.reshape(-1,2) # 储存棋盘格角点的世界坐标和图像坐标对 objpoints = [] # 在世界坐标系中的三维点 imgpoints = [] # 在图像平面的二维点 images = glob.glob('calib/*.png') for fname in images: img = cv2.imread(fname) gray = cv2.cvtColor(img,cv2.COLOR_BGR2GRAY) # 找到棋盘格角点 ret, corners = cv2.findChessboardCorners(gray, (w,h),None) # 如果找到足够点对,将其存储起来 if ret == True: cv2.cornerSubPix(gray,corners,(11,11),(-1,-1),criteria) objpoints.append(objp) imgpoints.append(corners) # 将角点在图像上显示 cv2.drawChessboardCorners(img, (w,h), corners, ret) cv2.imshow('findCorners',img) cv2.waitKey(1) cv2.destroyAllWindows() # 标定 ret, mtx, dist, rvecs, tvecs = cv2.calibrateCamera(objpoints, imgpoints, gray.shape[::-1], None, None) # 去畸变 img2 = cv2.imread('calib/00169.png') h, w = img2.shape[:2] newcameramtx, roi=cv2.getOptimalNewCameraMatrix(mtx,dist,(w,h),0,(w,h)) # 自由比例参数 dst = cv2.undistort(img2, mtx, dist, None, newcameramtx) # 根据前面ROI区域裁剪图片 #x,y,w,h = roi #dst = dst[y:y+h, x:x+w] cv2.imwrite('calibresult.png',dst) # 反投影误差 total_error = 0 for i in xrange(len(objpoints)): imgpoints2, _ = cv2.projectPoints(objpoints[i], rvecs[i], tvecs[i], mtx, dist) error = cv2.norm(imgpoints[i],imgpoints2, cv2.NORM_L2)/len(imgpoints2) total_error += error print "total error: ", total_error/len(objpoints)
Related recommendations:
The data in OpenCVcv::Mat is written to the txt file
OpenCV cv.Mat and .txt file data reading and writing operations
The above is the detailed content of Python uses OpenCV for calibration. For more information, please follow other related articles on the PHP Chinese website!

Hot AI Tools

Undresser.AI Undress
AI-powered app for creating realistic nude photos

AI Clothes Remover
Online AI tool for removing clothes from photos.

Undress AI Tool
Undress images for free

Clothoff.io
AI clothes remover

Video Face Swap
Swap faces in any video effortlessly with our completely free AI face swap tool!

Hot Article

Hot Tools

Notepad++7.3.1
Easy-to-use and free code editor

SublimeText3 Chinese version
Chinese version, very easy to use

Zend Studio 13.0.1
Powerful PHP integrated development environment

Dreamweaver CS6
Visual web development tools

SublimeText3 Mac version
God-level code editing software (SublimeText3)

Hot Topics
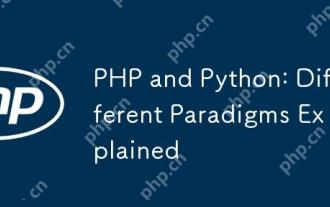
PHP is mainly procedural programming, but also supports object-oriented programming (OOP); Python supports a variety of paradigms, including OOP, functional and procedural programming. PHP is suitable for web development, and Python is suitable for a variety of applications such as data analysis and machine learning.
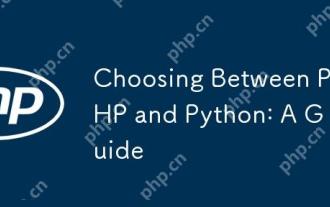
PHP is suitable for web development and rapid prototyping, and Python is suitable for data science and machine learning. 1.PHP is used for dynamic web development, with simple syntax and suitable for rapid development. 2. Python has concise syntax, is suitable for multiple fields, and has a strong library ecosystem.
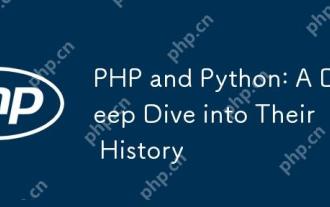
PHP originated in 1994 and was developed by RasmusLerdorf. It was originally used to track website visitors and gradually evolved into a server-side scripting language and was widely used in web development. Python was developed by Guidovan Rossum in the late 1980s and was first released in 1991. It emphasizes code readability and simplicity, and is suitable for scientific computing, data analysis and other fields.
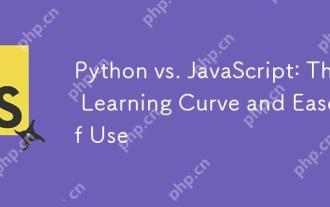
Python is more suitable for beginners, with a smooth learning curve and concise syntax; JavaScript is suitable for front-end development, with a steep learning curve and flexible syntax. 1. Python syntax is intuitive and suitable for data science and back-end development. 2. JavaScript is flexible and widely used in front-end and server-side programming.
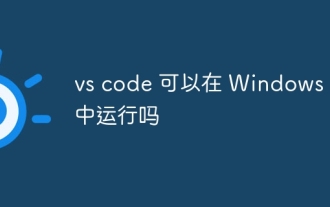
VS Code can run on Windows 8, but the experience may not be great. First make sure the system has been updated to the latest patch, then download the VS Code installation package that matches the system architecture and install it as prompted. After installation, be aware that some extensions may be incompatible with Windows 8 and need to look for alternative extensions or use newer Windows systems in a virtual machine. Install the necessary extensions to check whether they work properly. Although VS Code is feasible on Windows 8, it is recommended to upgrade to a newer Windows system for a better development experience and security.
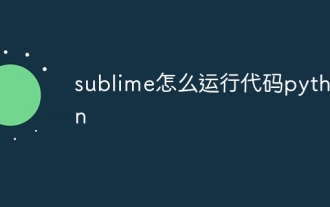
To run Python code in Sublime Text, you need to install the Python plug-in first, then create a .py file and write the code, and finally press Ctrl B to run the code, and the output will be displayed in the console.
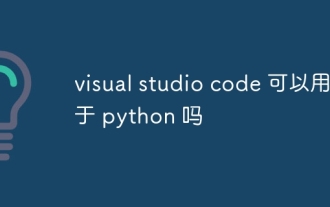
VS Code can be used to write Python and provides many features that make it an ideal tool for developing Python applications. It allows users to: install Python extensions to get functions such as code completion, syntax highlighting, and debugging. Use the debugger to track code step by step, find and fix errors. Integrate Git for version control. Use code formatting tools to maintain code consistency. Use the Linting tool to spot potential problems ahead of time.
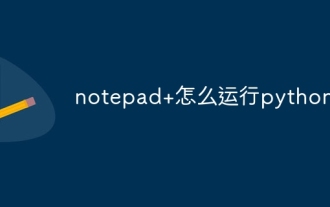
Running Python code in Notepad requires the Python executable and NppExec plug-in to be installed. After installing Python and adding PATH to it, configure the command "python" and the parameter "{CURRENT_DIRECTORY}{FILE_NAME}" in the NppExec plug-in to run Python code in Notepad through the shortcut key "F6".
