


Detailed introduction to how to use Naive Bayes algorithm in python
This article mainly introduces the relevant knowledge of how to use the Naive Bayes algorithm in Python. Has very good reference value. Let’s take a look at it with the editor
Here I’ll repeat why the title is “use” instead of “implementation”:
First of all, the algorithms provided by professionals are better than the algorithms we write ourselves. Both efficiency and accuracy are high.
Secondly, for people who are not good at mathematics, it is very painful to study a bunch of formulas in order to implement the algorithm.
Again, unless the algorithms provided by others cannot meet your needs, there is no need to "reinvent the wheel".
Let’s get back to the point. If you don’t know the Bayesian algorithm, you can check the relevant information. Here is just a brief introduction:
1. Bayesian formula:
P(A|B)=P(AB)/P(B)
2. Bayesian inference:
P(A|B)=P(A)×P(B|A)/P(B)
Express in words:
Posterior probability = prior probability × similarity/standardized constant
The problem that the Bayesian algorithm needs to solve is how to find the similarity, that is: P(B|A ) value
3. Three commonly used naive Bayes algorithms are provided in the scikit-learn package, which are explained in turn below:
1) Gaussian Naive Bayes: Assume that attributes/features obey the normal distribution (as shown below), and is mainly used for numerical features.
Use the data that comes with the scikit-learn package. The code and description are as follows:
>>>from sklearn import datasets ##导入包中的数据 >>> iris=datasets.load_iris() ##加载数据 >>> iris.feature_names ##显示特征名字 ['sepal length (cm)', 'sepal width (cm)', 'petal length (cm)', 'petal width (cm)'] >>> iris.data ##显示数据 array([[ 5.1, 3.5, 1.4, 0.2],[ 4.9, 3. , 1.4, 0.2],[ 4.7, 3.2, 1.3, 0.2]............ >>> iris.data.size ##数据大小 ---600个 >>> iris.target_names ##显示分类的名字 array(['setosa', 'versicolor', 'virginica'], dtype='<U10') >>> from sklearn.naive_bayes import GaussianNB ##导入高斯朴素贝叶斯算法 >>> clf = GaussianNB() ##给算法赋一个变量,主要是为了方便使用 >>> clf.fit(iris.data, iris.target) ##开始分类。对于量特别大的样本,可以使用函数partial_fit分类,避免一次加载过多数据到内存 >>> clf.predict(iris.data[0].reshape(1,-1)) ##验证分类。标红部分特别说明:因为predict的参数是数组,data[0]是列表,所以需要转换一下 array([0]) >>> data=np.array([6,4,6,2]) ##验证分类 >>> clf.predict(data.reshape(1,-1)) array([2])
There is a problem involved here: How to judge whether the data conforms to the normal distribution? There are related function judgments in the R language, or you can see it through direct drawing, but it is all a situation where P(x,y) can be drawn directly in the coordinate system. What about the data in the example? OK, I haven’t figured it out yet, this part will be added later.
2) Multinomial distribution Naive Bayes: often used for text classification, the feature is the word, and the value is the number of times the word appears. ##示例来在官方文档,详细说明见第一个例子
>>> import numpy as np
>>> X = np.random.randint(5, size=(6, 100)) ##返回随机整数值:范围[0,5) 大小6*100 6行100列
>>> y = np.array([1, 2, 3, 4, 5, 6])
>>> from sklearn.naive_bayes import MultinomialNB
>>> clf = MultinomialNB()
>>> clf.fit(X, y)
MultinomialNB(alpha=1.0, class_prior=None, fit_prior=True)
>>> print(clf.predict(X[2]))
[3]
3) Bernoulli Naive Bayes: Each feature is of Boolean type, and the result is 0 or 1, that is, it does not appear. The above is the detailed content of Detailed introduction to how to use Naive Bayes algorithm in python. For more information, please follow other related articles on the PHP Chinese website!##示例来在官方文档,详细说明见第一个例子
>>> import numpy as np
>>> X = np.random.randint(2, size=(6, 100))
>>> Y = np.array([1, 2, 3, 4, 4, 5])
>>> from sklearn.naive_bayes import BernoulliNB
>>> clf = BernoulliNB()
>>> clf.fit(X, Y)
BernoulliNB(alpha=1.0, binarize=0.0, class_prior=None, fit_prior=True)
>>> print(clf.predict(X[2]))
[3]

Hot AI Tools

Undresser.AI Undress
AI-powered app for creating realistic nude photos

AI Clothes Remover
Online AI tool for removing clothes from photos.

Undress AI Tool
Undress images for free

Clothoff.io
AI clothes remover

Video Face Swap
Swap faces in any video effortlessly with our completely free AI face swap tool!

Hot Article

Hot Tools

Notepad++7.3.1
Easy-to-use and free code editor

SublimeText3 Chinese version
Chinese version, very easy to use

Zend Studio 13.0.1
Powerful PHP integrated development environment

Dreamweaver CS6
Visual web development tools

SublimeText3 Mac version
God-level code editing software (SublimeText3)

Hot Topics










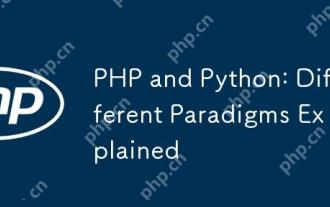
PHP is mainly procedural programming, but also supports object-oriented programming (OOP); Python supports a variety of paradigms, including OOP, functional and procedural programming. PHP is suitable for web development, and Python is suitable for a variety of applications such as data analysis and machine learning.
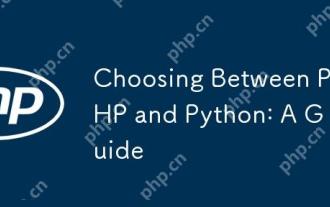
PHP is suitable for web development and rapid prototyping, and Python is suitable for data science and machine learning. 1.PHP is used for dynamic web development, with simple syntax and suitable for rapid development. 2. Python has concise syntax, is suitable for multiple fields, and has a strong library ecosystem.
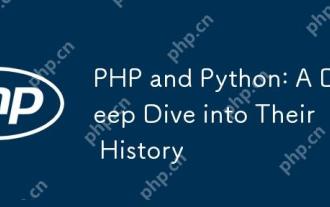
PHP originated in 1994 and was developed by RasmusLerdorf. It was originally used to track website visitors and gradually evolved into a server-side scripting language and was widely used in web development. Python was developed by Guidovan Rossum in the late 1980s and was first released in 1991. It emphasizes code readability and simplicity, and is suitable for scientific computing, data analysis and other fields.
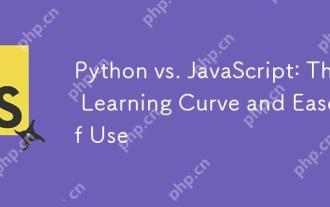
Python is more suitable for beginners, with a smooth learning curve and concise syntax; JavaScript is suitable for front-end development, with a steep learning curve and flexible syntax. 1. Python syntax is intuitive and suitable for data science and back-end development. 2. JavaScript is flexible and widely used in front-end and server-side programming.
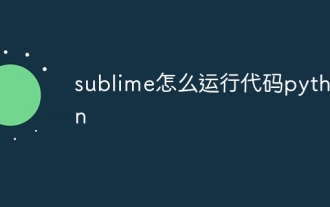
To run Python code in Sublime Text, you need to install the Python plug-in first, then create a .py file and write the code, and finally press Ctrl B to run the code, and the output will be displayed in the console.
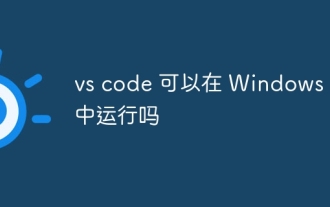
VS Code can run on Windows 8, but the experience may not be great. First make sure the system has been updated to the latest patch, then download the VS Code installation package that matches the system architecture and install it as prompted. After installation, be aware that some extensions may be incompatible with Windows 8 and need to look for alternative extensions or use newer Windows systems in a virtual machine. Install the necessary extensions to check whether they work properly. Although VS Code is feasible on Windows 8, it is recommended to upgrade to a newer Windows system for a better development experience and security.
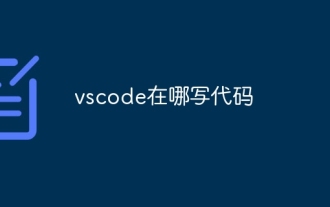
Writing code in Visual Studio Code (VSCode) is simple and easy to use. Just install VSCode, create a project, select a language, create a file, write code, save and run it. The advantages of VSCode include cross-platform, free and open source, powerful features, rich extensions, and lightweight and fast.
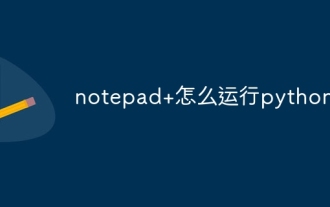
Running Python code in Notepad requires the Python executable and NppExec plug-in to be installed. After installing Python and adding PATH to it, configure the command "python" and the parameter "{CURRENT_DIRECTORY}{FILE_NAME}" in the NppExec plug-in to run Python code in Notepad through the shortcut key "F6".
