


Python automated operation and maintenance and deployment project tool Fabric usage examples
Fabric is a Python library. As long as the target machine supports ssh access, you can use fabric to perform remote operations (such as running shell commands remotely on host1 on host2). Obviously, since fabric is a Python package, other Python packages are It can be imported into fabric's unique fabfile.py script
Fabric is a good tool for automated operation and maintenance and deployment projects developed using Python. It can automatically interact with remote servers through SSH, such as The local file is transferred to the server and the shell command is executed on the server.
The following is an example of automated deployment of a Django project
# -*- coding: utf-8 -*- # 文件名要保存为 fabfile.py from __future__ import unicode_literals from fabric.api import * # 登录用户和主机名: env.user = 'root' # 如果没有设置,在需要登录的时候,fabric 会提示输入 env.password = 'youpassword' # 如果有多个主机,fabric会自动依次部署 env.hosts = ['www.example.com'] TAR_FILE_NAME = 'deploy.tar.gz' def pack(): """ 定义一个pack任务, 打一个tar包 :return: """ tar_files = ['*.py', 'static/*', 'templates/*', 'vue_app/', '*/*.py', 'requirements.txt'] exclude_files = ['fabfile.py', 'deploy/*', '*.tar.gz', '.DS_Store', '*/.DS_Store', '*/.*.py', '__pycache__/*'] exclude_files = ['--exclude=\'%s\'' % t for t in exclude_files] local('rm -f %s' % TAR_FILE_NAME) local('tar -czvf %s %s %s' % (TAR_FILE_NAME, ' '.join(exclude_files), ' '.join(tar_files))) print('在当前目录创建一个打包文件: %s' % TAR_FILE_NAME) def deploy(): """ 定义一个部署任务 :return: """ # 先进行打包 pack() # 远程服务器的临时文件 remote_tmp_tar = '/tmp/%s' % TAR_FILE_NAME run('rm -f %s' % remote_tmp_tar) # 上传tar文件至远程服务器, local_path, remote_path put(TAR_FILE_NAME, remote_tmp_tar) # 解压 remote_dist_base_dir = '/home/python/django_app' # 如果不存在, 则创建文件夹 run('mkdir -p %s' % remote_dist_dir) # cd 命令将远程主机的工作目录切换到指定目录 with cd(remote_dist_dir): print('解压文件到到目录: %s' % remote_dist_dir) run('tar -xzvf %s' % remote_tmp_tar) print('安装 requirements.txt 中的依赖包') # 我使用的是 python3 来开发 run('pip3 install -r requirements.txt') remote_settings_file = '%s/django_app/settings.py' % remote_dist_dir settings_file = 'deploy/settings.py' % name print('上传 settings.py 文件 %s' % settings_file) put(settings_file, remote_settings_file) nginx_file = 'deploy/django_app.conf' remote_nginx_file = '/etc/nginx/conf.d/django_app.conf' print('上传 nginx 配置文件 %s' % nginx_file) put(nginx_file, remote_nginx_file) # 在当前目录的子目录 deploy 中的 supervisor 配置文件上传至服务器 supervisor_file = 'deploy/django_app.ini' remote_supervisor_file = '/etc/supervisord.d/django_app.ini' print('上传 supervisor 配置文件 %s' % supervisor_file) put(supervisor_file, remote_supervisor_file) # 重新加载 nginx 的配置文件 run('nginx -s reload') run('nginx -t') # 删除本地的打包文件 local('rm -f %s' % TAR_FILE_NAME) # 载入最新的配置文件,停止原有进程并按新的配置启动所有进程 run('supervisorctl reload') # 执行 restart all,start 或者 stop fabric 都会提示错误,然后中止运行 # 但是服务器上查看日志,supervisor 有重启 # run('supervisorctl restart all')
Execute pack task
fab pack<br/>
Execute deploy task
fab deploy
Let me share with you an automated deployment of code using Fabric
#coding=utf-8 from fabric.api import local, abort, settings, env, cd, run from fabric.colors import * from fabric.contrib.console import confirm env.hosts = ["root@115.28.×××××"] env.password = "×××××" def get_git_status(): git_status_result = local("git status", capture=True) if "无文件要提交,干净的工作区" not in git_status_result: print red("****当前分支还有文件没有提交") print git_status_result abort("****已经终止") def local_unit_test(): with settings(warn_only=True): test_result = local("python manage.py test") if test_result.failed: print test_result if not confirm(red("****单元测试失败,是否继续?")): abort("****已经终止") def server_unit_test(): with settings(warn_only=True): test_result = run("python manage.py test") if test_result.failed: print test_result if not confirm(red("****单元测试失败,是否继续?")): abort("****已经终止") def upload_code(): local("git push origin dev") print green("****代码上传成功") def deploy_at_server(): print green("****ssh到服务器进行下列操作") with cd("/var/www/××××××"): #print run("pwd") print green("****将在远程仓库下载代码") run("git checkout dev") get_git_status() run("git pull origin dev") print green("****将在服务器上运行单元测试") server_unit_test() run("service apache2 restart", pty=False) print green("****重启apache2成功") print green("********代码部署成功********") def deploy(): get_git_status() local("git checkout dev", capture=False) print green("****切换到dev分支") get_git_status() print green("****将开始运行单元测试") local_unit_test() print green("****单元测试完成,开始上传代码") upload_code() deploy_at_server()
fabric can solidify the commands for automated deployment or multi-machine operation into a script, thereby reducing manual operations. The above is written after I came into contact with this thing for the first time today. It is indeed very practical. Just run fab deploy
.
The main logic is to run the unit test on the local dev branch, then submit it to the server, log in to the server via ssh, pull it down, run the unit test again, and then restart apache2. The first time I write it, it may be relatively simple, and I will continue to improve it.
For more Python automated operation and maintenance and deployment project tool Fabric usage examples related articles, please pay attention to the PHP Chinese website!

Hot AI Tools

Undresser.AI Undress
AI-powered app for creating realistic nude photos

AI Clothes Remover
Online AI tool for removing clothes from photos.

Undress AI Tool
Undress images for free

Clothoff.io
AI clothes remover

Video Face Swap
Swap faces in any video effortlessly with our completely free AI face swap tool!

Hot Article

Hot Tools

Notepad++7.3.1
Easy-to-use and free code editor

SublimeText3 Chinese version
Chinese version, very easy to use

Zend Studio 13.0.1
Powerful PHP integrated development environment

Dreamweaver CS6
Visual web development tools

SublimeText3 Mac version
God-level code editing software (SublimeText3)

Hot Topics










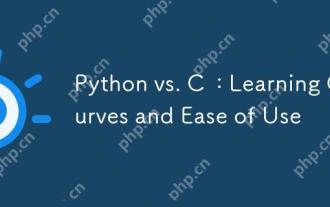
Python is easier to learn and use, while C is more powerful but complex. 1. Python syntax is concise and suitable for beginners. Dynamic typing and automatic memory management make it easy to use, but may cause runtime errors. 2.C provides low-level control and advanced features, suitable for high-performance applications, but has a high learning threshold and requires manual memory and type safety management.
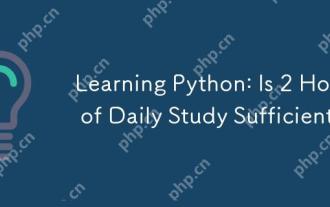
Is it enough to learn Python for two hours a day? It depends on your goals and learning methods. 1) Develop a clear learning plan, 2) Select appropriate learning resources and methods, 3) Practice and review and consolidate hands-on practice and review and consolidate, and you can gradually master the basic knowledge and advanced functions of Python during this period.
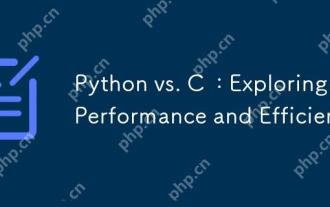
Python is better than C in development efficiency, but C is higher in execution performance. 1. Python's concise syntax and rich libraries improve development efficiency. 2.C's compilation-type characteristics and hardware control improve execution performance. When making a choice, you need to weigh the development speed and execution efficiency based on project needs.
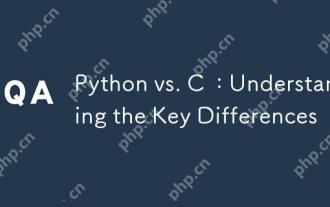
Python and C each have their own advantages, and the choice should be based on project requirements. 1) Python is suitable for rapid development and data processing due to its concise syntax and dynamic typing. 2)C is suitable for high performance and system programming due to its static typing and manual memory management.
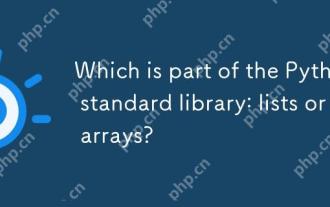
Pythonlistsarepartofthestandardlibrary,whilearraysarenot.Listsarebuilt-in,versatile,andusedforstoringcollections,whereasarraysareprovidedbythearraymoduleandlesscommonlyusedduetolimitedfunctionality.
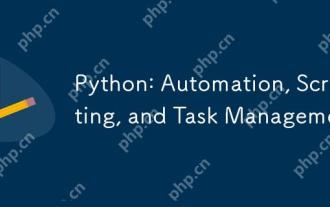
Python excels in automation, scripting, and task management. 1) Automation: File backup is realized through standard libraries such as os and shutil. 2) Script writing: Use the psutil library to monitor system resources. 3) Task management: Use the schedule library to schedule tasks. Python's ease of use and rich library support makes it the preferred tool in these areas.
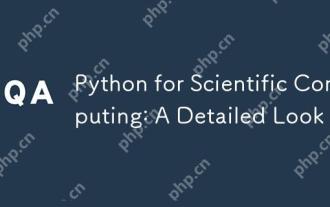
Python's applications in scientific computing include data analysis, machine learning, numerical simulation and visualization. 1.Numpy provides efficient multi-dimensional arrays and mathematical functions. 2. SciPy extends Numpy functionality and provides optimization and linear algebra tools. 3. Pandas is used for data processing and analysis. 4.Matplotlib is used to generate various graphs and visual results.
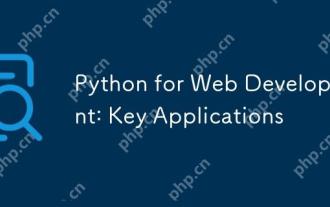
Key applications of Python in web development include the use of Django and Flask frameworks, API development, data analysis and visualization, machine learning and AI, and performance optimization. 1. Django and Flask framework: Django is suitable for rapid development of complex applications, and Flask is suitable for small or highly customized projects. 2. API development: Use Flask or DjangoRESTFramework to build RESTfulAPI. 3. Data analysis and visualization: Use Python to process data and display it through the web interface. 4. Machine Learning and AI: Python is used to build intelligent web applications. 5. Performance optimization: optimized through asynchronous programming, caching and code
