


Python data cleaning: data merging, conversion, filtering and sorting
Earlier we used pandas to perform some basic operations. Next, we will learn more about data operations.
Data cleaning has always been an extremely important part of data analysis.
Data merging
In pandas, data can be merged through merge.
import numpy as np import pandas as pd data1 = pd.DataFrame({'level':['a','b','c','d'], 'numeber':[1,3,5,7]}) data2=pd.DataFrame({'level':['a','b','c','e'], 'numeber':[2,3,6,10]}) print(data1)
The result is:
##
print(data2)
The result is:
print(pd.merge(data1,data2))
The result is:
You can see that the fields used for the same label in data1 and data2 are displayed, while other fields are discarded. This is equivalent to the inner join connection operation in SQL.
data3 = pd.DataFrame({'level1':['a','b','c','d'], 'numeber1':[1,3,5,7]}) data4=pd.DataFrame({'level2':['a','b','c','e'], 'numeber2':[2,3,6,10]}) print(pd.merge(data3,data4,left_on='level1',right_on='level2'))
The result is:
If the column in the two data frames When the names are different, we can connect the data together by specifying the two parameters letf_on and right_on
print(pd.merge(data3,data4,left_on='level1',right_on='level2',how='left'))
The result is:
Other detailed parameter description
Sometimes We will encounter overlapping data that needs to be merged. In this case, we can use the comebine_first function.
data3 = pd.DataFrame({'level':['a','b','c','d'], 'numeber1':[1,3,5,np.nan]}) data4=pd.DataFrame({'level':['a','b','c','e'], 'numeber2':[2,np.nan,6,10]}) print(data3.combine_first(data4))
The result is:
You can see the results under the same tag The content of data3 is displayed first. If a certain data in a data frame is missing, the elements in another data frame will be filled in.
The usage here is similar to np.where (isnull(a),b,a)
We mentioned this content in the previous pandas article. Data reshaping mainly uses the reshape function, and rotation mainly uses the unstack and stack functions.
data=pd.DataFrame(np.arange(12).reshape(3,4), columns=['a','b','c','d'], index=['wang','li','zhang']) print(data)
The result is:
##
print(data.unstack())
The result is:
Data conversion
Delete duplicate row data
data=pd.DataFrame({'a':[1,3,3,4], 'b':[1,3,3,5]}) print(data)
print(data.duplicated())
It can be seen that the third row repeats the data of the second row, so the displayed result is True
另外用drop_duplicates方法可以去除重复行
print(data.drop_duplicates())
结果为:
替换值
除了使用我们上一篇文章中提到的fillna的方法外,还可以用replace方法,而且更简单快捷
data=pd.DataFrame({'a':[1,3,3,4], 'b':[1,3,3,5]}) print(data.replace(1,2))
结果为:
多个数据一起换
print(data.replace([1,4],np.nan))
数据分段
data=[11,15,18,20,25,26,27,24] bins=[15,20,25] print(data) print(pd.cut(data,bins))
结果为:
[11, 15, 18, 20, 25, 26, 27, 24][NaN, NaN, (15, 20], (15, 20], (20, 25], NaN, NaN, (20, 25]]
Categories (2, object): [(15, 20] < (20, 25]]
可以看出分段后的结果,不在分段内的数据显示为na值,其他则显示数据所在的分段。
print(pd.cut(data,bins).labels)
结果为:
[-1 -1 0 0 1 -1 -1 1]
显示所在分段排序标签
print(pd.cut(data,bins).levels)
结果为:
Index([‘(15, 20]', ‘(20, 25]'], dtype='object')
显示所以分段标签
print(value_counts(pd.cut(data,bins)))
结果为:
显示每个分段值得个数
此外还有一个qcut的函数可以对数据进行4分位切割,用法和cut类似。
排列和采样
我们知道排序的方法有好几个,比如sort,order,rank等函数都能对数据进行排序
现在要说的这个是对数据进行随机排序(permutation)
data=np.random.permutation(5) print(data)
结果为:
[1 0 4 2 3]
这里的peemutation函数对0-4的数据进行随机排序的结果。
也可以对数据进行采样
df=pd.DataFrame(np.arange(12).reshape(4,3)) samp=np.random.permutation(3) print(df)
结果为:
print(samp)
结果为:
[1 0 2]
print(df.take(samp))
结果为:
这里使用take的结果是,按照samp的顺序从df中提取样本。
更多python 数据清洗之数据合并、转换、过滤、排序相关文章请关注PHP中文网!

Hot AI Tools

Undresser.AI Undress
AI-powered app for creating realistic nude photos

AI Clothes Remover
Online AI tool for removing clothes from photos.

Undress AI Tool
Undress images for free

Clothoff.io
AI clothes remover

Video Face Swap
Swap faces in any video effortlessly with our completely free AI face swap tool!

Hot Article

Hot Tools

Notepad++7.3.1
Easy-to-use and free code editor

SublimeText3 Chinese version
Chinese version, very easy to use

Zend Studio 13.0.1
Powerful PHP integrated development environment

Dreamweaver CS6
Visual web development tools

SublimeText3 Mac version
God-level code editing software (SublimeText3)

Hot Topics










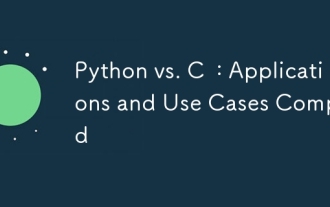
Python is suitable for data science, web development and automation tasks, while C is suitable for system programming, game development and embedded systems. Python is known for its simplicity and powerful ecosystem, while C is known for its high performance and underlying control capabilities.
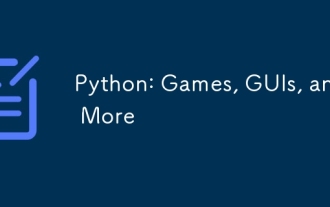
Python excels in gaming and GUI development. 1) Game development uses Pygame, providing drawing, audio and other functions, which are suitable for creating 2D games. 2) GUI development can choose Tkinter or PyQt. Tkinter is simple and easy to use, PyQt has rich functions and is suitable for professional development.
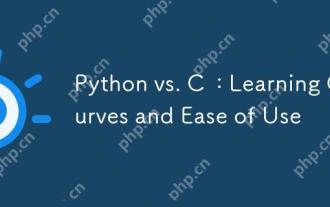
Python is easier to learn and use, while C is more powerful but complex. 1. Python syntax is concise and suitable for beginners. Dynamic typing and automatic memory management make it easy to use, but may cause runtime errors. 2.C provides low-level control and advanced features, suitable for high-performance applications, but has a high learning threshold and requires manual memory and type safety management.
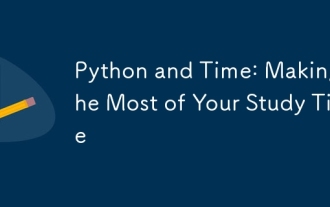
To maximize the efficiency of learning Python in a limited time, you can use Python's datetime, time, and schedule modules. 1. The datetime module is used to record and plan learning time. 2. The time module helps to set study and rest time. 3. The schedule module automatically arranges weekly learning tasks.
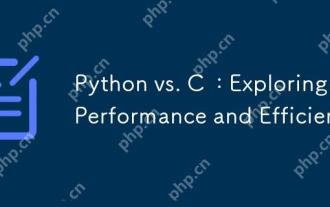
Python is better than C in development efficiency, but C is higher in execution performance. 1. Python's concise syntax and rich libraries improve development efficiency. 2.C's compilation-type characteristics and hardware control improve execution performance. When making a choice, you need to weigh the development speed and execution efficiency based on project needs.
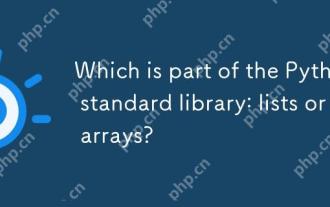
Pythonlistsarepartofthestandardlibrary,whilearraysarenot.Listsarebuilt-in,versatile,andusedforstoringcollections,whereasarraysareprovidedbythearraymoduleandlesscommonlyusedduetolimitedfunctionality.
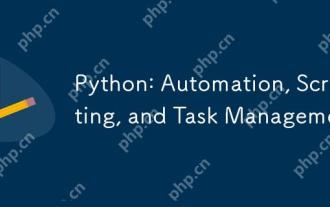
Python excels in automation, scripting, and task management. 1) Automation: File backup is realized through standard libraries such as os and shutil. 2) Script writing: Use the psutil library to monitor system resources. 3) Task management: Use the schedule library to schedule tasks. Python's ease of use and rich library support makes it the preferred tool in these areas.
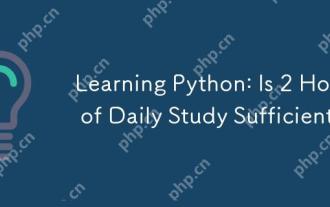
Is it enough to learn Python for two hours a day? It depends on your goals and learning methods. 1) Develop a clear learning plan, 2) Select appropriate learning resources and methods, 3) Practice and review and consolidate hands-on practice and review and consolidate, and you can gradually master the basic knowledge and advanced functions of Python during this period.
