


Scaling XML/RSS Processing: Performance Optimization Techniques
When processing XML and RSS data, you can optimize performance through the following steps: 1) Use efficient parsers such as lxml to improve parsing speed; 2) Use SAX parsers to reduce memory usage; 3) Use XPath expressions to improve data extraction efficiency; 4) implement multi-process parallel processing to improve processing speed.
introduction
Performance optimization becomes a key challenge when dealing with large-scale XML and RSS data. Whether you are developing a news aggregator or need to process large amounts of XML data for data analysis, how to process this data efficiently is crucial. This article will explore in-depth various performance optimization techniques that can be used when processing XML and RSS data. By reading this article, you will learn how to improve the performance of your XML/RSS handlers, avoid common performance bottlenecks, and master some practical best practices.
Review of basic knowledge
Processing XML and RSS data usually involves the process of parsing, transforming and extracting information. XML is a markup language used to store and transfer data, while RSS is an XML-based format used to publish frequently updated content, such as blog posts, news titles, etc. Common tools for processing this data include SAX (Simple API for XML) and DOM (Document Object Model) parsers, as well as specialized RSS parsing libraries.
When working with large-scale data, it is crucial to choose the right analytics method. The SAX parser processes data in a streaming manner and is suitable for handling large files because it does not require the entire document to be loaded into memory. The DOM parser loads the entire XML document into memory and forms a tree structure, suitable for situations where documents need to be frequently accessed and modified.
Core concept or function analysis
Performance optimization of XML/RSS processing
Performance optimization mainly involves the following aspects in XML/RSS processing: parsing speed, memory usage, data extraction efficiency and parallel processing capabilities.
Analysis speed
Parse speed is one of the core indicators of XML/RSS processing. Using efficient parsers such as Expat or libxml2 can significantly improve parsing speed. Here is an example of XML parsing using Python's lxml library:
from lxml import etree # Read XML file with open('example.xml', 'r') as file: xml_content = file.read() # parse XML root = etree.fromstring(xml_content) # Extract data for element in root.findall('.//item'): title = element.find('title').text print(title)
This example shows how to quickly parse XML files and extract data from them using the lxml library. The lxml library is based on libxml2 and has efficient parsing performance.
Memory usage
Memory usage is a special issue when dealing with large-scale XML files. Using a SAX parser can effectively reduce memory footprint, as it does not require the entire document to be loaded into memory. Here is an example using the SAX parser:
import xml.sax class MyHandler(xml.sax.ContentHandler): def __init__(self): self.current_data = "" self.title = "" def startElement(self, tag, attributes): self.current_data = tag def endElement(self, tag): if self.current_data == "title": print(self.title) self.current_data = "" def characters(self, content): if self.current_data == "title": self.title = content parser = xml.sax.make_parser() parser.setContentHandler(MyHandler()) parser.parse("example.xml")
This example shows how to use the SAX parser to process XML files, avoiding the risk of memory overflow.
Data extraction efficiency
When extracting data, selecting the appropriate XPath expression can significantly improve efficiency. XPath is a language used to navigate in XML documents that can quickly locate the required data. Here is an example of extracting data using XPath:
from lxml import etree # Read XML file with open('example.xml', 'r') as file: xml_content = file.read() # parse XML root = etree.fromstring(xml_content) # Use XPath to extract data titles = root.xpath('//item/title/text()') for title in titles: print(title)
This example shows how to use XPath to quickly extract data from XML, improving the efficiency of data extraction.
Parallel processing
When processing large-scale data, utilizing multi-threading or multi-processing can significantly improve processing speed. Here is an example of parallel processing using Python's multiprocessing library:
import multiprocessing from lxml import etree def process_chunk(chunk): root = etree.fromstring(chunk) titles = root.xpath('//item/title/text()') Return titles if __name__ == '__main__': with open('example.xml', 'r') as file: xml_content = file.read() # Divide XML files into multiple chunks chunks = [xml_content[i:i 100000] for i in range(0, len(xml_content), 100000)] # Use multiprocessing with multiprocessing.Pool(processes=4) as pool: results = pool.map(process_chunk, chunks) # Merge result all_titles = [title for chunk_result in results for title in chunk_result] for title in all_titles: print(title)
This example shows how to use multiple processes to process XML files in parallel, which improves processing speed.
Example of usage
Basic usage
When processing XML/RSS data, the most basic usage is to use a parser to read files and extract data. Here is an example of basic parsing using Python's xml.etree.ElementTree library:
import xml.etree.ElementTree as ET # Read XML file tree = ET.parse('example.xml') root = tree.getroot() # Extract data for item in root.findall('item'): title = item.find('title').text print(title)
This example shows how to use the ElementTree library for basic XML parsing and data extraction.
Advanced Usage
When dealing with complex XML/RSS data, more advanced techniques may be required, such as XPath expressions and namespace processing. Here is an example of processing using XPath and namespace:
from lxml import etree # Read XML file with open('example.xml', 'r') as file: xml_content = file.read() # parse XML root = etree.fromstring(xml_content) # Define namespace ns = {'atom': 'http://www.w3.org/2005/Atom'} # Use XPath to extract data titles = root.xpath('//atom:entry/atom:title/text()', namespaces=ns) for title in titles: print(title)
This example shows how to use XPath and namespace to process complex XML data, improving the flexibility of data extraction.
Common Errors and Debugging Tips
Common errors when processing XML/RSS data include parsing errors, namespace conflicts, and memory overflow. Here are some common errors and their debugging tips:
- Parse error : Use the try-except statement to capture the parse error and print the detailed error message. For example:
try: tree = etree.parse('example.xml') except etree.XMLSyntaxError as e: print(f"Parse error: {e}")
- Namespace conflict : Ensure that namespaces are correctly defined and used to avoid namespace conflicts. For example:
ns = {'atom': 'http://www.w3.org/2005/Atom'} titles = root.xpath('//atom:entry/atom:title/text()', namespaces=ns)
- Memory overflow : Use SAX parser to process large files to avoid memory overflow. For example:
import xml.sax class MyHandler(xml.sax.ContentHandler): def __init__(self): self.current_data = "" self.title = "" def startElement(self, tag, attributes): self.current_data = tag def endElement(self, tag): if self.current_data == "title": print(self.title) self.current_data = "" def characters(self, content): if self.current_data == "title": self.title = content parser = xml.sax.make_parser() parser.setContentHandler(MyHandler()) parser.parse("example.xml")
Performance optimization and best practices
In practical applications, the following aspects need to be considered for optimizing XML/RSS processing code:
Choose the right parser : Choose SAX or DOM parser according to the specific needs. SAX parsers are suitable for handling large files, while DOM parsers are suitable for situations where frequent access and modification of documents are required.
Using XPath Expression : XPath Expression can significantly improve the efficiency of data extraction and reduce code complexity.
Parallel processing : Use multi-threading or multi-processing to process data in parallel to improve processing speed.
Memory management : When processing large files, pay attention to memory usage to avoid memory overflow.
Code readability and maintenance : Write clear and readable code to facilitate subsequent maintenance and extension.
Here is an example that combines the above optimization techniques:
import multiprocessing from lxml import etree def process_chunk(chunk): root = etree.fromstring(chunk) titles = root.xpath('//item/title/text()') Return titles if __name__ == '__main__': with open('example.xml', 'r') as file: xml_content = file.read() # Divide XML files into multiple chunks chunks = [xml_content[i:i 100000] for i in range(0, len(xml_content), 100000)] # Use multiprocessing with multiprocessing.Pool(processes=4) as pool: results = pool.map(process_chunk, chunks) # Merge result all_titles = [title for chunk_result in results for title in chunk_result] for title in all_titles: print(title)
This example shows how to use multi-process, XPath expressions and memory management techniques to improve the performance of XML/RSS processing.
In practical applications, performance optimization is a continuous process that requires continuous adjustment and optimization according to specific needs and data characteristics. Hopefully, the techniques and practices provided in this article can help you achieve better performance when processing XML/RSS data.
The above is the detailed content of Scaling XML/RSS Processing: Performance Optimization Techniques. For more information, please follow other related articles on the PHP Chinese website!

Hot AI Tools

Undresser.AI Undress
AI-powered app for creating realistic nude photos

AI Clothes Remover
Online AI tool for removing clothes from photos.

Undress AI Tool
Undress images for free

Clothoff.io
AI clothes remover

Video Face Swap
Swap faces in any video effortlessly with our completely free AI face swap tool!

Hot Article

Hot Tools

Notepad++7.3.1
Easy-to-use and free code editor

SublimeText3 Chinese version
Chinese version, very easy to use

Zend Studio 13.0.1
Powerful PHP integrated development environment

Dreamweaver CS6
Visual web development tools

SublimeText3 Mac version
God-level code editing software (SublimeText3)

Hot Topics
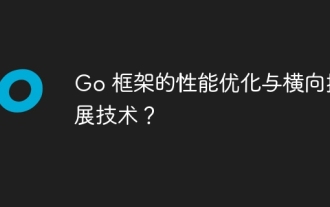
In order to improve the performance of Go applications, we can take the following optimization measures: Caching: Use caching to reduce the number of accesses to the underlying storage and improve performance. Concurrency: Use goroutines and channels to execute lengthy tasks in parallel. Memory Management: Manually manage memory (using the unsafe package) to further optimize performance. To scale out an application we can implement the following techniques: Horizontal Scaling (Horizontal Scaling): Deploying application instances on multiple servers or nodes. Load balancing: Use a load balancer to distribute requests to multiple application instances. Data sharding: Distribute large data sets across multiple databases or storage nodes to improve query performance and scalability.
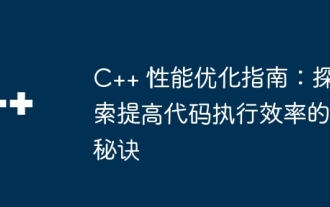
C++ performance optimization involves a variety of techniques, including: 1. Avoiding dynamic allocation; 2. Using compiler optimization flags; 3. Selecting optimized data structures; 4. Application caching; 5. Parallel programming. The optimization practical case shows how to apply these techniques when finding the longest ascending subsequence in an integer array, improving the algorithm efficiency from O(n^2) to O(nlogn).
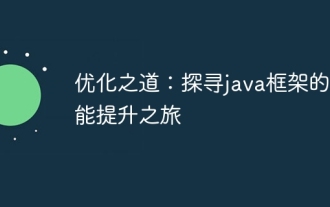
The performance of Java frameworks can be improved by implementing caching mechanisms, parallel processing, database optimization, and reducing memory consumption. Caching mechanism: Reduce the number of database or API requests and improve performance. Parallel processing: Utilize multi-core CPUs to execute tasks simultaneously to improve throughput. Database optimization: optimize queries, use indexes, configure connection pools, and improve database performance. Reduce memory consumption: Use lightweight frameworks, avoid leaks, and use analysis tools to reduce memory consumption.
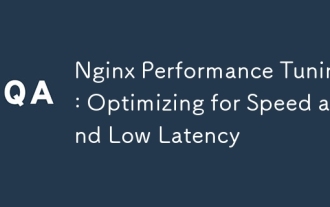
Nginx performance tuning can be achieved by adjusting the number of worker processes, connection pool size, enabling Gzip compression and HTTP/2 protocols, and using cache and load balancing. 1. Adjust the number of worker processes and connection pool size: worker_processesauto; events{worker_connections1024;}. 2. Enable Gzip compression and HTTP/2 protocol: http{gzipon;server{listen443sslhttp2;}}. 3. Use cache optimization: http{proxy_cache_path/path/to/cachelevels=1:2k
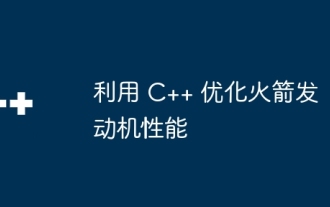
By building mathematical models, conducting simulations and optimizing parameters, C++ can significantly improve rocket engine performance: Build a mathematical model of a rocket engine and describe its behavior. Simulate engine performance and calculate key parameters such as thrust and specific impulse. Identify key parameters and search for optimal values using optimization algorithms such as genetic algorithms. Engine performance is recalculated based on optimized parameters to improve its overall efficiency.
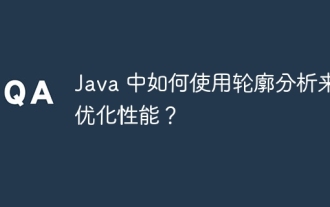
Profiling in Java is used to determine the time and resource consumption in application execution. Implement profiling using JavaVisualVM: Connect to the JVM to enable profiling, set the sampling interval, run the application, stop profiling, and the analysis results display a tree view of the execution time. Methods to optimize performance include: identifying hotspot reduction methods and calling optimization algorithms
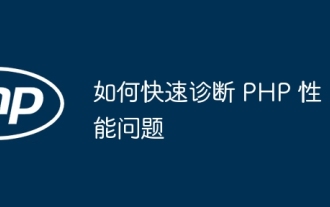
Effective techniques for quickly diagnosing PHP performance issues include using Xdebug to obtain performance data and then analyzing the Cachegrind output. Use Blackfire to view request traces and generate performance reports. Examine database queries to identify inefficient queries. Analyze memory usage, view memory allocations and peak usage.
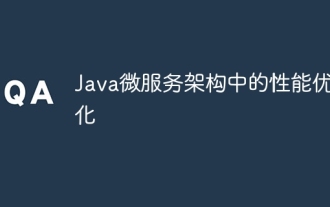
Performance optimization for Java microservices architecture includes the following techniques: Use JVM tuning tools to identify and adjust performance bottlenecks. Optimize the garbage collector and select and configure a GC strategy that matches your application's needs. Use a caching service such as Memcached or Redis to improve response times and reduce database load. Employ asynchronous programming to improve concurrency and responsiveness. Split microservices, breaking large monolithic applications into smaller services to improve scalability and performance.
