


What happens if you try to store a value of the wrong data type in a Python array?
When you attempt to store a value of the wrong data type in a Python array, you'll encounter a TypeError. This is due to the array module's strict type enforcement, which requires all elements to be of the same type as specified by the typecode. For performance reasons, arrays are more efficient than lists but less flexible, as lists can hold mixed types. If flexibility is needed, consider using lists or NumPy arrays, which offer both performance and type conversion capabilities.
When you attempt to store a value of the wrong data type in a Python array, you'll encounter a TypeError
. Let's dive into why this happens and explore the nuances of Python's type system.
In Python, arrays are typically implemented using the array
module, which is more strict about types than Python lists. The array
module requires all elements to be of the same type, specified by a typecode when you create the array. For instance, if you create an array with the typecode 'i' for integers, trying to insert a string or a float will raise an error.
Here's an example to illustrate this:
import array # Create an array of integers int_array = array.array('i', [1, 2, 3]) # Attempt to append a string try: int_array.append('hello') except TypeError as e: print(f"TypeError: {e}")
The output will be something like:
TypeError: an integer is required (got type str)
This strictness is part of Python's philosophy of "duck typing," where the type of an object is less important than the methods it implements. However, in the case of the array
module, the type enforcement is necessary for performance reasons, as arrays are designed to be more efficient than lists for certain operations.
Now, let's delve deeper into the implications and explore some practical scenarios.
When working with arrays, understanding the type system is crucial. If you need a more flexible structure, you might opt for Python lists instead, which can hold mixed types. However, this flexibility comes at the cost of performance and memory efficiency.
Here's a comparison between arrays and lists:
import array import timeit # Array of integers int_array = array.array('i', range(1000000)) # List of integers int_list = list(range(1000000)) # Time array access array_time = timeit.timeit(lambda: int_array[500000], number=1000000) # Time list access list_time = timeit.timeit(lambda: int_list[500000], number=1000000) print(f"Array access time: {array_time:.6f} seconds") print(f"List access time: {list_time:.6f} seconds")
You'll likely see that the array access is faster, highlighting the performance benefits of using arrays when type consistency is guaranteed.
However, the strictness of arrays can sometimes be a pitfall. If your data evolves over time and you need to add different types, you'll be forced to either convert your array to a list or create a new array with a different typecode, which can be cumbersome.
In my experience, I've found that the choice between arrays and lists often depends on the specific requirements of the project. For data processing tasks where performance is critical and the data type is known and fixed, arrays are a great choice. But for more dynamic data structures, lists offer the needed flexibility.
If you're working with large datasets and need the performance of arrays but also the flexibility of lists, you might consider using NumPy arrays. NumPy arrays are more flexible than the standard array
module while still offering excellent performance.
Here's an example of using NumPy arrays:
import numpy as np # Create a NumPy array of integers np_array = np.array([1, 2, 3]) # Append a float (NumPy will convert it to the common type) np_array = np.append(np_array, 4.5) print(np_array) # Output: [1. 2. 3. 4.5]
NumPy arrays automatically handle type conversion, which can be both a blessing and a curse. While it provides flexibility, it can also lead to unexpected behavior if not managed carefully.
In conclusion, attempting to store a value of the wrong data type in a Python array will result in a TypeError
. This strictness is a double-edged sword: it ensures performance and type consistency but can limit flexibility. Understanding these trade-offs is essential for effective Python programming. Whether you choose arrays, lists, or NumPy arrays depends on your specific needs, and sometimes, a combination of these tools might be the best approach.
The above is the detailed content of What happens if you try to store a value of the wrong data type in a Python array?. For more information, please follow other related articles on the PHP Chinese website!

Hot AI Tools

Undresser.AI Undress
AI-powered app for creating realistic nude photos

AI Clothes Remover
Online AI tool for removing clothes from photos.

Undress AI Tool
Undress images for free

Clothoff.io
AI clothes remover

Video Face Swap
Swap faces in any video effortlessly with our completely free AI face swap tool!

Hot Article

Hot Tools

Notepad++7.3.1
Easy-to-use and free code editor

SublimeText3 Chinese version
Chinese version, very easy to use

Zend Studio 13.0.1
Powerful PHP integrated development environment

Dreamweaver CS6
Visual web development tools

SublimeText3 Mac version
God-level code editing software (SublimeText3)

Hot Topics










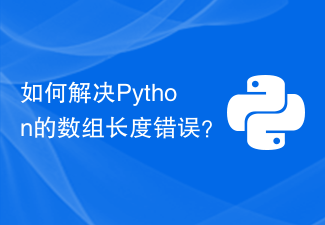
Python is a high-level programming language widely used in fields such as data analysis and machine learning. Among them, array is one of the commonly used data structures in Python, but during the development process, array length errors are often encountered. This article will detail how to solve Python's array length error. Length of Array First, we need to know the length of the array. In Python, the length of an array can vary, that is, we can modify the length of the array by adding or removing elements from the array. because
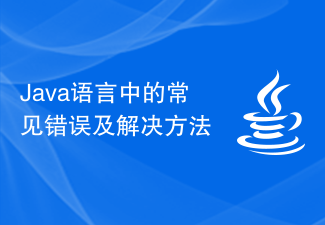
With the development and popularity of Java language, more and more people are beginning to learn and use Java language. However, in the process of learning and applying the Java language, we often encounter some errors and problems. This article will introduce common errors and solutions in the Java language to help readers use the Java language more smoothly. 1. The variable is not initialized. In the Java language, if the declared variable is not initialized, it cannot be used directly. Otherwise, the program will prompt an error that the variable is not initialized. This question can be answered by giving
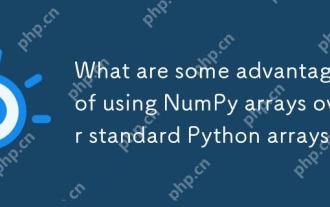
NumPyarrayshaveseveraladvantagesoverstandardPythonarrays:1)TheyaremuchfasterduetoC-basedimplementation,2)Theyaremorememory-efficient,especiallywithlargedatasets,and3)Theyofferoptimized,vectorizedfunctionsformathematicalandstatisticaloperations,making
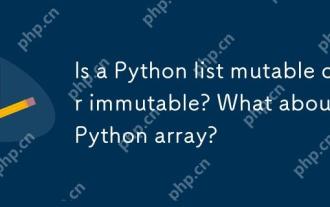
Pythonlistsandarraysarebothmutable.1)Listsareflexibleandsupportheterogeneousdatabutarelessmemory-efficient.2)Arraysaremorememory-efficientforhomogeneousdatabutlessversatile,requiringcorrecttypecodeusagetoavoiderrors.
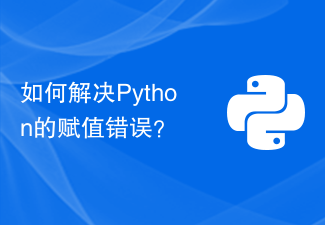
Python is a simple and easy-to-learn high-level programming language, and its flexibility and scalability are loved by programmers. But when writing Python code, we often encounter some assignment errors. These errors may cause our program to fail or even fail to compile. This article will discuss how to solve assignment errors in Python and help you write better Python code. Variables and Assignment in Python In Python, we use variables to store values. A variable is a dynamic entity that can store different types of
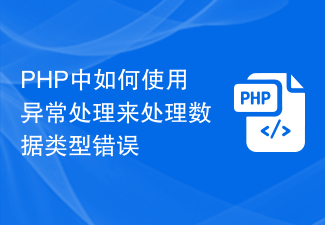
PHP is a commonly used server-side scripting language used to develop dynamic websites and web applications. When performing data processing, data type errors are often encountered. In order to handle these errors, PHP provides an exception handling mechanism. This article will share how to use exception handling to handle data type errors, with code examples. Exception handling is a mechanism for handling errors while a program is running. When an error occurs, an exception can be thrown to notify the code that an abnormal condition has occurred during execution. In PHP, exceptions are thrown via the slang
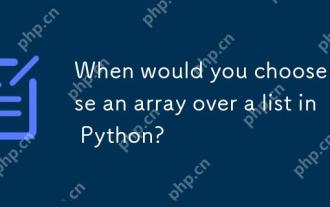
Useanarray.arrayoveralistinPythonwhendealingwithhomogeneousdata,performance-criticalcode,orinterfacingwithCcode.1)HomogeneousData:Arrayssavememorywithtypedelements.2)Performance-CriticalCode:Arraysofferbetterperformancefornumericaloperations.3)Interf
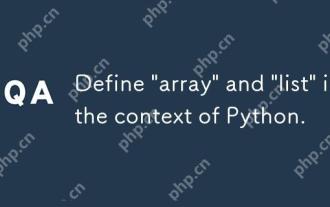
InPython,a"list"isaversatile,mutablesequencethatcanholdmixeddatatypes,whilean"array"isamorememory-efficient,homogeneoussequencerequiringelementsofthesametype.1)Listsareidealfordiversedatastorageandmanipulationduetotheirflexibility
