Understanding Hit Rate, MRR, and MMR Metrics
Introduction
Imagine browsing a bookstore for the perfect read. You crave genre-specific recommendations, yet desire variety to discover new authors. Retrieval-Augmented Generation (RAG) systems function similarly, blending information retrieval with creative response generation. Evaluating their performance requires metrics like Hit Rate (measuring successful recommendations) and Mean Reciprocal Rank (MRR, considering recommendation order). Maximum Marginal Relevance (MMR) ensures both relevance and diversity in suggestions. These metrics guarantee accurate, varied, and engaging recommendations.
Overview
This guide explores Hit Rate, MMR, and their roles in evaluating RAG systems. You'll learn to apply MMR for balancing relevance and diversity in retrieved results, calculate Hit Rate and MRR for assessing retrieval effectiveness, and analyze/improve RAG systems using these performance metrics.
Table of contents
- What is Hit Rate?
- Calculating Hit Rate
- Hit Rate Limitations
- Mean Reciprocal Rank (MRR)
- Calculating MRR
- Maximum Marginal Relevance (MMR)
- Calculating MMR
- MMR Example
- Frequently Asked Questions
What is Hit Rate?
Hit Rate assesses recommendation system performance by measuring how often the desired item appears within the top-N recommendations. In RAG, it signifies the frequency of successfully incorporating relevant data into the generated output.
Calculating Hit Rate?
Hit Rate is calculated by dividing the number of queries where the relevant item appears in the top-N recommendations by the total number of queries. Mathematically:
Example: Three queries (Q1, Q2, Q3) with corresponding target nodes (N1, N2, N3). Retrieved nodes are:
Hit Rate is 1 for Q1 and Q2, 0 for Q3. Therefore:
Hit Rate Limitations
Hit Rate's primary limitation is its disregard for the retrieved node's position. Consider two retrievers:
Both have the same Hit Rate (66.66%), yet Retriever 2 consistently ranks correct nodes higher (position 1 vs. position 3 for Q1). This highlights the need for a metric that considers ranking.
Mean Reciprocal Rank (MRR)
Mean Reciprocal Rank (MRR) evaluates information retrieval systems by considering the rank of the first relevant item in a ranked list. In RAG, it assesses the retrieval component's performance in finding relevant documents for accurate response generation.
Calculating MRR?
Where N is the number of queries, and ranki is the rank of the first relevant document for query i.
Example:
MRR for Q1 is 1/3. Therefore:
Note that despite a 66.66% Hit Rate, MRR is 44.4%, rewarding retrievers that place relevant items higher.
Maximum Marginal Relevance (MMR)
Maximum Marginal Relevance (MMR) re-ranks results to optimize both relevance and diversity. It balances novelty and relevance to ensure retrieved items comprehensively address query aspects.
Calculating MMR?
λ (mmr_threshold) controls the relevance/diversity trade-off. λ close to 1 prioritizes relevance; λ close to 0 prioritizes diversity.
MMR Example
Using the same example as Hit Rate, with assumed relevance and similarity scores, and λ = 0.5:
The detailed MMR calculation and re-ranking for Q1, Q2, and Q3 are provided in the original document. The final re-ranked nodes illustrate the balance MMR achieves between relevance and diversity.
Conclusion
Hit Rate, MRR, and MMR are crucial for evaluating and improving RAG systems. Hit Rate and MRR focus on relevant information retrieval frequency and ranking, while MMR balances relevance and diversity. Optimizing these metrics enhances RAG system response quality and user experience.
Frequently Asked Questions
Q1. What is Hit Rate? A. It's the ratio of queries with relevant items in the top-N recommendations to the total number of queries.
Q2. What is MMR? A. Maximum Marginal Relevance is a re-ranking technique balancing relevance and diversity in retrieved results.
Q3. Why is Hit Rate important for RAG? A. A higher Hit Rate indicates better retrieval of relevant information, leading to more accurate responses.
Q4. Why is MMR important for RAG? A. MMR ensures diverse and relevant results, providing comprehensive answers to queries.
The above is the detailed content of Understanding Hit Rate, MRR, and MMR Metrics. For more information, please follow other related articles on the PHP Chinese website!

Hot AI Tools

Undresser.AI Undress
AI-powered app for creating realistic nude photos

AI Clothes Remover
Online AI tool for removing clothes from photos.

Undress AI Tool
Undress images for free

Clothoff.io
AI clothes remover

Video Face Swap
Swap faces in any video effortlessly with our completely free AI face swap tool!

Hot Article

Hot Tools

Notepad++7.3.1
Easy-to-use and free code editor

SublimeText3 Chinese version
Chinese version, very easy to use

Zend Studio 13.0.1
Powerful PHP integrated development environment

Dreamweaver CS6
Visual web development tools

SublimeText3 Mac version
God-level code editing software (SublimeText3)

Hot Topics
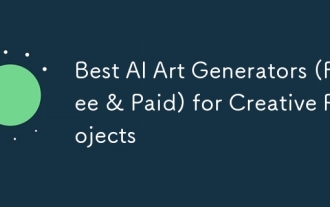
The article reviews top AI art generators, discussing their features, suitability for creative projects, and value. It highlights Midjourney as the best value for professionals and recommends DALL-E 2 for high-quality, customizable art.
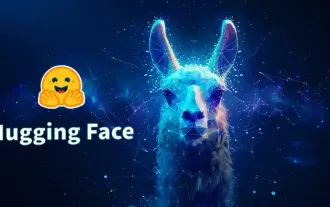
Meta's Llama 3.2: A Leap Forward in Multimodal and Mobile AI Meta recently unveiled Llama 3.2, a significant advancement in AI featuring powerful vision capabilities and lightweight text models optimized for mobile devices. Building on the success o
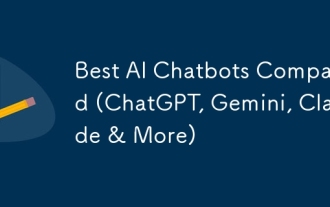
The article compares top AI chatbots like ChatGPT, Gemini, and Claude, focusing on their unique features, customization options, and performance in natural language processing and reliability.
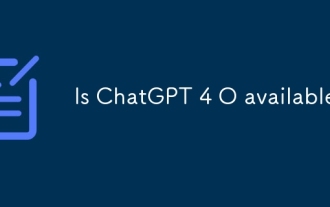
ChatGPT 4 is currently available and widely used, demonstrating significant improvements in understanding context and generating coherent responses compared to its predecessors like ChatGPT 3.5. Future developments may include more personalized interactions and real-time data processing capabilities, further enhancing its potential for various applications.
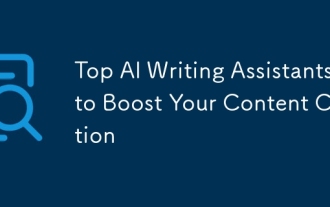
The article discusses top AI writing assistants like Grammarly, Jasper, Copy.ai, Writesonic, and Rytr, focusing on their unique features for content creation. It argues that Jasper excels in SEO optimization, while AI tools help maintain tone consist
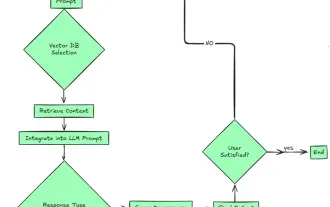
2024 witnessed a shift from simply using LLMs for content generation to understanding their inner workings. This exploration led to the discovery of AI Agents – autonomous systems handling tasks and decisions with minimal human intervention. Buildin
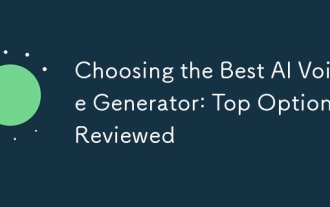
The article reviews top AI voice generators like Google Cloud, Amazon Polly, Microsoft Azure, IBM Watson, and Descript, focusing on their features, voice quality, and suitability for different needs.
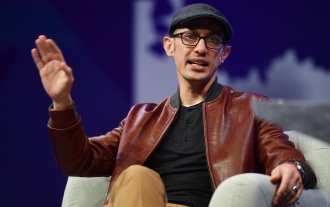
Shopify CEO Tobi Lütke's recent memo boldly declares AI proficiency a fundamental expectation for every employee, marking a significant cultural shift within the company. This isn't a fleeting trend; it's a new operational paradigm integrated into p
