Can Quantum-Inspired AI Compete With Today's Large Language Models?
Dynex, a company from Liechtenstein, recently launched its Quantum Diffusion Large Language Model (qdLLM) in its SXSW 2025 Innovation Award final, becoming a compelling development. The company claims its qdLLM is able to generate generative AI output faster and more efficiently than traditional Transformer-based systems that rely on current technology infrastructure.
How does this compare to other emerging approaches? What does this mean for the broader future of AI?
The significance of quantum computing to AI
The core difference of quantum computing is that it uses qubits, which can exist in multiple states at the same time due to quantum superposition. This allows quantum computers to evaluate a large number of potential solutions in parallel, which may have advantages in tasks such as large-scale optimization, simulation, or pattern recognition.
In the field of AI, researchers have explored how quantum features can improve tasks such as natural language processing, machine learning optimization, and model training efficiency. However, most of these efforts are still in their early stages. For example, IBM and MIT have studied how hybrid quantum classical models reduce training time for specific deep learning tasks, while startups such as Zapata AI are experimenting with quantum enhancement models for sentiment analysis and prediction.
In this context, Dynex's approach introduces a new architecture that uses quantum heuristic algorithms to run LLM more efficiently through decentralized hardware.
Dynex's qdLLM: A diffusion-based parallel approach
Unlike Transformer-based models that use autoregression techniques to generate one tag at a time, Dynex's qdLLM is built on a diffusion model that creates output tags in parallel. According to Dynex, this approach is more computationally efficient and produces better contextual consistency.
“Traditional models like GPT-4 or DeepSeek work sequentially, word after word,” said Daniela Herrmann, Dynex co-founder and task leader at Dynex Moonshots. "qdLLM works in parallel. It thinks more like the human brain, processing all patterns at once. That's the power of quantum."
Several academic projects including Stanford University and Google DeepMind, as well as initiatives from major AI technology providers, have recently begun exploring the diffusion-based Transformer.
Dynex further differentiates itself by integrating quantum annealing, a quantum optimization form, to improve mark selection during text generation. This increases consistency and reduces computational overhead compared to traditional LLMs, the company claims.
Decentralized and analog quantum hardware
One unique feature of the Dynex model is that it relies on a decentralized GPU network that simulates quantum behavior rather than requiring access to actual quantum hardware. This design allows the system to scale to up to one million algorithmic qubits described by Dynex.
"Any quantum algorithm, such as qdLLM, is being computed on the decentralized network of the GPU, which effectively simulate quantum computing," Herrmann explained.
This type of simulation has some similarities with the work of TensorFlow Quantum (Google and X) which also simulates quantum circuits on classic hardware to create algorithm prototypes. Similarly, many tech startups and vendors are developing platforms to simulate quantum logic at scale before physical hardware is ready.
In addition to software, Dynex plans to launch its own neuromorphic quantum chip Apollo in 2025. Unlike superconducting quantum chips that require low temperature cooling, Apollo is designed to operate at room temperature and supports integration into edge devices.
"Using neuromorphic circuits allows Dynex to simulate quantum computing at scale, up to 1 million algorithmic qubits," Herrmann explained. “Dynex will start producing actual quantum chips that are also based on neuromorphic paradigms.”
Quantum impact on AI efficiency and environmental impact
Dynex says qdLLM achieves 90% smaller model sizes, 10 times faster, and uses only 10% of the GPU resources typically used for equivalent tasks. These are important statements, especially given the increasing concern about AI energy consumption.
"The efficiency and parallelism of quantum algorithms reduce energy consumption because it is 10 times faster and requires only 10% of the number of GPUs," Herrmann said.
While independent verification is still required, Dynex's approach echoes the efforts of Cerebras Systems, which has created wafer-level chips that use less energy for training tasks. Another example is Graphcore, whose Intelligent Processing Unit (IPU) is designed to reduce the energy footprint of AI workloads through a dedicated parallel architecture.
Dynex reports that qdLLM performs strongly in benchmarks requiring strong inference, outperforming leading models, including ChatGPT and Grok. While public benchmark data has not been released yet, the company said it will release a comparative study as it is closer to the 2025 market launch. Dynex's performance assertions remain anecdotal, but interesting until it is provided with peer-reviewed benchmarks.
“We publish qdLLM benchmarks regularly and have proven that certain questions that require strong reasoning cannot be answered correctly by ChatGPT, Grok or DeepSeek,” Herrmann noted.
A bigger picture: How will quantum affect AI?
In the long run, Dynex believes that quantum computing will become the core of the AI field.
"We think quantum will dominate AI for the next five years," Herrmann said.
This prediction remains speculative, although not without precedent. Analysts at McKinsey, Boston Consulting Group and Gartner all point out that quantum computing can greatly improve optimization and simulation tasks, but for most use cases, it may not be possible until after 2030. A more cautious view suggests that quantum-AI hybrids will first appear in niche applications such as drug discovery, financial risk modeling, or cybersecurity.
Currently, Dynex is in a growing field that is experimenting with quantum augmentation or quantum heuristic AI methods. Whether their decentralized, diffusion-based qdLLM can surpass benchmarks remains to be seen, but its emergence suggests that searching for new foundations of AI is far from over.
The above is the detailed content of Can Quantum-Inspired AI Compete With Today's Large Language Models?. For more information, please follow other related articles on the PHP Chinese website!

Hot AI Tools

Undresser.AI Undress
AI-powered app for creating realistic nude photos

AI Clothes Remover
Online AI tool for removing clothes from photos.

Undress AI Tool
Undress images for free

Clothoff.io
AI clothes remover

Video Face Swap
Swap faces in any video effortlessly with our completely free AI face swap tool!

Hot Article

Hot Tools

Notepad++7.3.1
Easy-to-use and free code editor

SublimeText3 Chinese version
Chinese version, very easy to use

Zend Studio 13.0.1
Powerful PHP integrated development environment

Dreamweaver CS6
Visual web development tools

SublimeText3 Mac version
God-level code editing software (SublimeText3)

Hot Topics
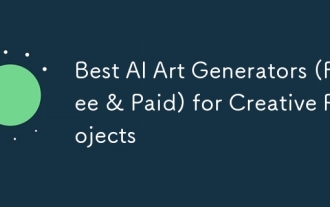
The article reviews top AI art generators, discussing their features, suitability for creative projects, and value. It highlights Midjourney as the best value for professionals and recommends DALL-E 2 for high-quality, customizable art.
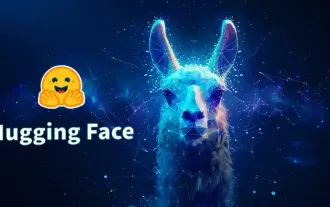
Meta's Llama 3.2: A Leap Forward in Multimodal and Mobile AI Meta recently unveiled Llama 3.2, a significant advancement in AI featuring powerful vision capabilities and lightweight text models optimized for mobile devices. Building on the success o
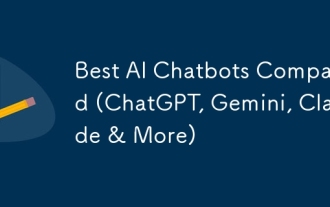
The article compares top AI chatbots like ChatGPT, Gemini, and Claude, focusing on their unique features, customization options, and performance in natural language processing and reliability.
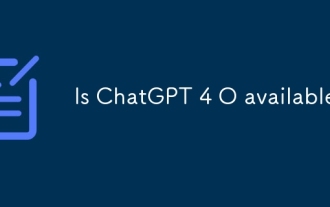
ChatGPT 4 is currently available and widely used, demonstrating significant improvements in understanding context and generating coherent responses compared to its predecessors like ChatGPT 3.5. Future developments may include more personalized interactions and real-time data processing capabilities, further enhancing its potential for various applications.
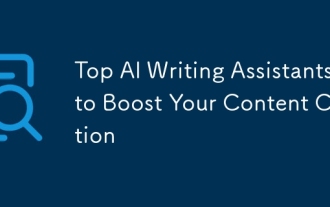
The article discusses top AI writing assistants like Grammarly, Jasper, Copy.ai, Writesonic, and Rytr, focusing on their unique features for content creation. It argues that Jasper excels in SEO optimization, while AI tools help maintain tone consist
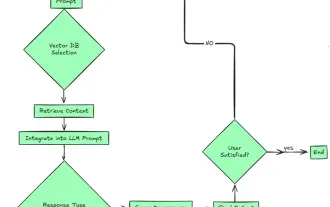
2024 witnessed a shift from simply using LLMs for content generation to understanding their inner workings. This exploration led to the discovery of AI Agents – autonomous systems handling tasks and decisions with minimal human intervention. Buildin
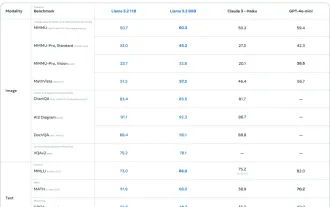
This week's AI landscape: A whirlwind of advancements, ethical considerations, and regulatory debates. Major players like OpenAI, Google, Meta, and Microsoft have unleashed a torrent of updates, from groundbreaking new models to crucial shifts in le
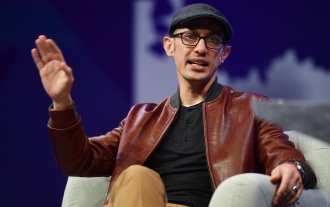
Shopify CEO Tobi Lütke's recent memo boldly declares AI proficiency a fundamental expectation for every employee, marking a significant cultural shift within the company. This isn't a fleeting trend; it's a new operational paradigm integrated into p
