Optimizing AI Performance: A Guide to Efficient LLM Deployment
Mastering Large Language Model (LLM) Serving for High-Performance AI Applications
The rise of artificial intelligence (AI) necessitates efficient LLM deployment for optimal innovation and productivity. Imagine AI-powered customer service anticipating your needs or data analysis tools delivering instant insights. This requires mastering LLM serving – transforming LLMs into high-performance, real-time applications. This article explores efficient LLM serving and deployment, covering optimal platforms, optimization strategies, and practical examples for creating powerful and responsive AI solutions.
Key Learning Objectives:
- Grasp the concept of LLM deployment and its importance in real-time applications.
- Examine various LLM serving frameworks, including their features and use cases.
- Gain practical experience with code examples for deploying LLMs using different frameworks.
- Learn to compare and benchmark LLM serving frameworks based on latency and throughput.
- Identify ideal scenarios for using specific LLM serving frameworks in various applications.
This article is part of the Data Science Blogathon.
Table of Contents:
- Introduction
- Triton Inference Server: A Deep Dive
- Optimizing HuggingFace Models for Production Text Generation
- vLLM: Revolutionizing Batch Processing for Language Models
- DeepSpeed-MII: Leveraging DeepSpeed for Efficient LLM Deployment
- OpenLLM: Adaptable Framework Integration
- Scaling Model Deployment with Ray Serve
- Accelerating Inference with CTranslate2
- Latency and Throughput Comparison
- Conclusion
- Frequently Asked Questions
Triton Inference Server: A Deep Dive
Triton Inference Server is a robust platform for deploying and scaling machine learning models in production. Developed by NVIDIA, it supports TensorFlow, PyTorch, ONNX, and custom backends.
Key Features:
- Model Management: Dynamic loading/unloading, version control.
- Inference Optimization: Multi-model ensembles, batching, dynamic batching.
- Metrics and Logging: Prometheus integration for monitoring.
- Accelerator Support: GPU, CPU, and DLA support.
Setup and Configuration:
Triton setup can be intricate, requiring Docker and Kubernetes familiarity. However, NVIDIA provides comprehensive documentation and community support.
Use Case:
Ideal for large-scale deployments demanding performance, scalability, and multi-framework support.
Demo Code and Explanation: (Code remains the same as in the original input)
Optimizing HuggingFace Models for Production Text Generation
This section focuses on using HuggingFace models for text generation, emphasizing native support without extra adapters. It uses model sharding for parallel processing, buffering for request management, and batching for efficiency. gRPC ensures fast communication between components.
Key Features:
- User-Friendliness: Seamless HuggingFace integration.
- Customization: Allows fine-tuning and custom configurations.
- Transformers Support: Leverages the Transformers library.
Use Cases:
Suitable for applications requiring direct HuggingFace model integration, such as chatbots and content generation.
Demo Code and Explanation: (Code remains the same as in the original input)
vLLM: Revolutionizing Batch Processing for Language Models
vLLM prioritizes speed in batched prompt delivery, optimizing latency and throughput. It uses vectorized operations and parallel processing for efficient batched text generation.
Key Features:
- High Performance: Optimized for low latency and high throughput.
- Batch Processing: Efficient handling of batched requests.
- Scalability: Suitable for large-scale deployments.
Use Cases:
Best for speed-critical applications, such as real-time translation and interactive AI systems.
Demo Code and Explanation: (Code remains the same as in the original input)
DeepSpeed-MII: Harnessing DeepSpeed for Efficient LLM Deployment
DeepSpeed-MII is for users experienced with DeepSpeed, focusing on efficient LLM deployment and scaling through model parallelism, memory efficiency, and speed optimization.
Key Features:
- Efficiency: Memory and computational efficiency.
- Scalability: Handles very large models.
- Integration: Seamless with DeepSpeed workflows.
Use Cases:
Ideal for researchers and developers familiar with DeepSpeed, prioritizing high-performance training and deployment.
Demo Code and Explanation: (Code remains the same as in the original input)
OpenLLM: Flexible Adapter Integration
OpenLLM connects adapters to the core model and uses HuggingFace Agents. It supports multiple frameworks, including PyTorch.
Key Features:
- Framework Agnostic: Supports multiple deep learning frameworks.
- Agent Integration: Leverages HuggingFace Agents.
- Adapter Support: Flexible integration with model adapters.
Use Cases:
Great for projects needing framework flexibility and extensive HuggingFace tool use.
Demo Code and Explanation: (Code remains the same as in the original input)
Leveraging Ray Serve for Scalable Model Deployment
Ray Serve provides a stable pipeline and flexible deployment for mature projects needing reliable and scalable solutions.
Key Features:
- Flexibility: Supports multiple deployment architectures.
- Scalability: Handles high-load applications.
- Integration: Works well with Ray’s ecosystem.
Use Cases:
Ideal for established projects requiring a robust and scalable serving infrastructure.
Demo Code and Explanation: (Code remains the same as in the original input)
Speeding Up Inference with CTranslate2
CTranslate2 prioritizes speed, especially for CPU-based inference. It’s optimized for translation models and supports various architectures.
Key Features:
- CPU Optimization: High performance for CPU inference.
- Compatibility: Supports popular model architectures.
- Lightweight: Minimal dependencies.
Use Cases:
Suitable for applications prioritizing CPU speed and efficiency, such as translation services.
Demo Code and Explanation: (Code remains the same as in the original input)
Latency and Throughput Comparison
(The table and image comparing latency and throughput remain the same as in the original input)
Conclusion
Efficient LLM serving is crucial for responsive AI applications. This article explored various platforms, each with unique advantages. The best choice depends on specific needs.
Key Takeaways:
- Model serving deploys trained models for inference.
- Different platforms excel in different performance aspects.
- Framework selection depends on the use case.
- Some frameworks are better for scalable deployments in mature projects.
Frequently Asked Questions:
(The FAQs remain the same as in the original input)
Note: The media shown in this article is not owned by [mention the relevant entity] and is used at the author's discretion.
The above is the detailed content of Optimizing AI Performance: A Guide to Efficient LLM Deployment. For more information, please follow other related articles on the PHP Chinese website!

Hot AI Tools

Undresser.AI Undress
AI-powered app for creating realistic nude photos

AI Clothes Remover
Online AI tool for removing clothes from photos.

Undress AI Tool
Undress images for free

Clothoff.io
AI clothes remover

Video Face Swap
Swap faces in any video effortlessly with our completely free AI face swap tool!

Hot Article

Hot Tools

Notepad++7.3.1
Easy-to-use and free code editor

SublimeText3 Chinese version
Chinese version, very easy to use

Zend Studio 13.0.1
Powerful PHP integrated development environment

Dreamweaver CS6
Visual web development tools

SublimeText3 Mac version
God-level code editing software (SublimeText3)

Hot Topics
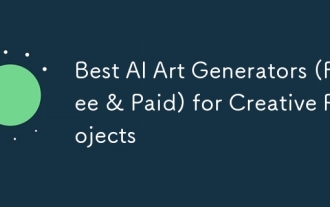
The article reviews top AI art generators, discussing their features, suitability for creative projects, and value. It highlights Midjourney as the best value for professionals and recommends DALL-E 2 for high-quality, customizable art.
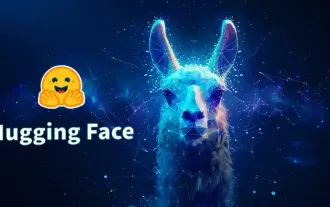
Meta's Llama 3.2: A Leap Forward in Multimodal and Mobile AI Meta recently unveiled Llama 3.2, a significant advancement in AI featuring powerful vision capabilities and lightweight text models optimized for mobile devices. Building on the success o
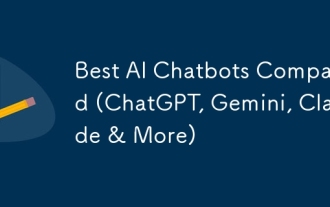
The article compares top AI chatbots like ChatGPT, Gemini, and Claude, focusing on their unique features, customization options, and performance in natural language processing and reliability.
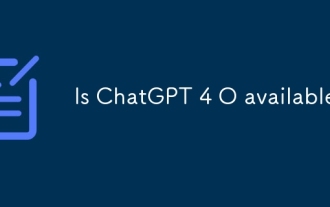
ChatGPT 4 is currently available and widely used, demonstrating significant improvements in understanding context and generating coherent responses compared to its predecessors like ChatGPT 3.5. Future developments may include more personalized interactions and real-time data processing capabilities, further enhancing its potential for various applications.
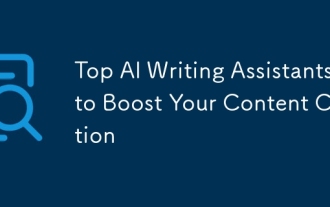
The article discusses top AI writing assistants like Grammarly, Jasper, Copy.ai, Writesonic, and Rytr, focusing on their unique features for content creation. It argues that Jasper excels in SEO optimization, while AI tools help maintain tone consist
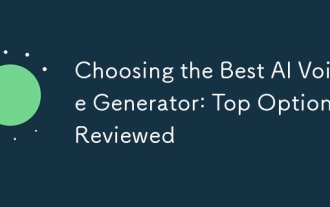
The article reviews top AI voice generators like Google Cloud, Amazon Polly, Microsoft Azure, IBM Watson, and Descript, focusing on their features, voice quality, and suitability for different needs.
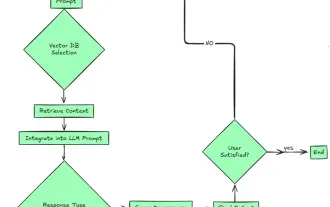
2024 witnessed a shift from simply using LLMs for content generation to understanding their inner workings. This exploration led to the discovery of AI Agents – autonomous systems handling tasks and decisions with minimal human intervention. Buildin
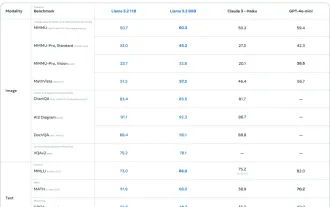
This week's AI landscape: A whirlwind of advancements, ethical considerations, and regulatory debates. Major players like OpenAI, Google, Meta, and Microsoft have unleashed a torrent of updates, from groundbreaking new models to crucial shifts in le
