


5 Statistical Tests Every Data Scientist Should Know - Analytics Vidhya
Data Science's Essential Statistical Tests: A Comprehensive Guide
Unlocking valuable insights from data is paramount in data science. Mastering statistical tests is fundamental to achieving this. These tests empower data scientists to rigorously validate hypotheses, compare diverse groups, uncover hidden relationships, and make confident predictions. Whether you're analyzing market trends, refining machine learning algorithms, or conducting scientific research, a strong grasp of statistical testing is indispensable. This article delves into the key statistical tests every data scientist should know.
Table of Contents:
- Introduction
- The Crucial Role of Statistical Tests in Data Science
- Five Must-Know Statistical Tests for Data Scientists
- Z-Test
- T-Test
- ANOVA (Analysis of Variance)
- F-Test
- Chi-Square Test
- Conclusion
The Importance of Statistical Tests in Data Science:
Statistical tests provide the objective framework for:
- Hypothesis Testing: Objectively determine if observed data patterns are genuine or merely random fluctuations.
- Data-Driven Decisions: Replace subjective opinions with quantitative evidence for informed decision-making.
- Group Comparisons: Enable meaningful comparisons across different datasets or experimental conditions.
- Relationship Discovery: Uncover and quantify relationships between variables within a dataset.
- Model Evaluation: Assess the accuracy and reliability of predictive models.
- Data Quality Assurance: Identify anomalies or significant shifts in data trends.
Five Essential Statistical Tests:
Z-Test
The z-test assesses whether a significant difference exists between a sample mean and a population mean, or between two sample means when population variances are known and sample sizes are large (generally, n > 30). It relies on the standard normal distribution (mean = 0, standard deviation = 1).
Formula (One-Sample Z-Test):
<code>z = (x̅ - μ) / (σ / √n)</code>
Where:
- x̅ = sample mean
- μ = hypothesized population mean
- σ = population standard deviation
- n = sample size
Conducting a Z-Test:
- Define Hypotheses: State the null (H₀: no significant difference) and alternative (H₁: a significant difference) hypotheses.
- Significance Level (α): Set the probability of rejecting a true null hypothesis (e.g., α = 0.05).
- Z-Test Type: Choose the appropriate test (one-sample, two-sample, or proportion).
- Calculate Z-Statistic: Use the relevant formula.
- Critical Value (z_critical): Determine the critical z-value from the standard normal distribution table based on α.
- Interpret Results: Compare the absolute value of the calculated z-statistic (|z|) to z_critical. Reject H₀ if |z| > z_critical.
T-Test
The t-test determines if there's a significant difference between the means of two groups. Unlike the z-test, it's used when population variances are unknown.
Types of T-Tests:
- One-Sample T-Test: Compares a sample mean to a hypothesized population mean.
- Independent Samples T-Test: Compares the means of two independent groups.
- Paired Samples T-Test: Compares the means of two related groups (e.g., before-and-after measurements).
Conducting a T-Test:
The steps are similar to the z-test, but use the appropriate t-test formula and consult the t-distribution table (considering degrees of freedom) to find the critical t-value.
ANOVA (Analysis of Variance)
ANOVA compares the means of three or more groups to identify statistically significant differences.
Types of ANOVA:
- One-Way ANOVA: Compares means across groups based on one factor.
- Two-Way ANOVA: Compares means based on two factors and their interaction.
- Repeated Measures ANOVA: Used when the same subjects are measured under multiple conditions.
Conducting ANOVA: ANOVA involves calculating sums of squares (SST, SSB, SSW), degrees of freedom, mean squares (MSB, MSW), and the F-statistic. The F-statistic is then compared to a critical F-value from the F-distribution table.
F-Test
The F-test compares the variances of two normally distributed populations. It determines if there's a statistically significant difference in data spread between the two groups.
Formula:
<code>F = σ₁² / σ₂²</code>
Where:
- σ₁² = variance of population 1
- σ₂² = variance of population 2
Conducting an F-Test: Calculate sample variances, compute the F-statistic, determine degrees of freedom, and compare the F-statistic to the critical F-value from the F-distribution table.
Chi-Square Test
The Chi-Square test assesses the association between two categorical variables.
Types of Chi-Square Tests:
- Chi-Square Test of Independence: Determines if there's a relationship between two categorical variables.
- Chi-Square Goodness-of-Fit Test: Evaluates how well a sample distribution matches a hypothesized distribution.
Conducting a Chi-Square Test: Both tests involve calculating the Chi-Square statistic (Χ²) using observed and expected frequencies. The Χ² value is then compared to a critical value from the Chi-Square distribution table.
Conclusion:
Statistical tests are indispensable tools for data scientists. Understanding their application and interpretation is crucial for drawing valid conclusions from data. By mastering these five tests—z-test, t-test, ANOVA, F-test, and Chi-Square test—data scientists can confidently analyze data, validate hypotheses, and make informed decisions. The choice of test depends on the research question, data type, and assumptions about the data.
The above is the detailed content of 5 Statistical Tests Every Data Scientist Should Know - Analytics Vidhya. For more information, please follow other related articles on the PHP Chinese website!

Hot AI Tools

Undresser.AI Undress
AI-powered app for creating realistic nude photos

AI Clothes Remover
Online AI tool for removing clothes from photos.

Undress AI Tool
Undress images for free

Clothoff.io
AI clothes remover

Video Face Swap
Swap faces in any video effortlessly with our completely free AI face swap tool!

Hot Article

Hot Tools

Notepad++7.3.1
Easy-to-use and free code editor

SublimeText3 Chinese version
Chinese version, very easy to use

Zend Studio 13.0.1
Powerful PHP integrated development environment

Dreamweaver CS6
Visual web development tools

SublimeText3 Mac version
God-level code editing software (SublimeText3)

Hot Topics
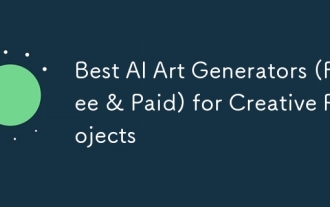
The article reviews top AI art generators, discussing their features, suitability for creative projects, and value. It highlights Midjourney as the best value for professionals and recommends DALL-E 2 for high-quality, customizable art.
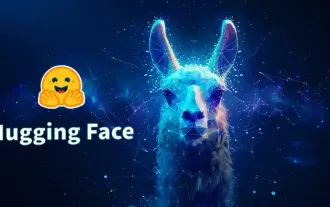
Meta's Llama 3.2: A Leap Forward in Multimodal and Mobile AI Meta recently unveiled Llama 3.2, a significant advancement in AI featuring powerful vision capabilities and lightweight text models optimized for mobile devices. Building on the success o
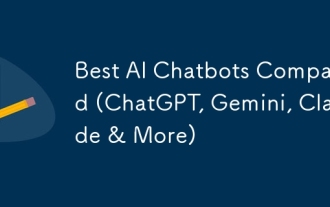
The article compares top AI chatbots like ChatGPT, Gemini, and Claude, focusing on their unique features, customization options, and performance in natural language processing and reliability.
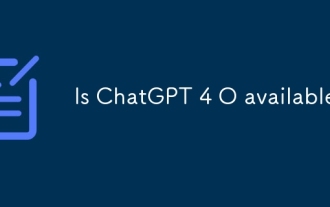
ChatGPT 4 is currently available and widely used, demonstrating significant improvements in understanding context and generating coherent responses compared to its predecessors like ChatGPT 3.5. Future developments may include more personalized interactions and real-time data processing capabilities, further enhancing its potential for various applications.
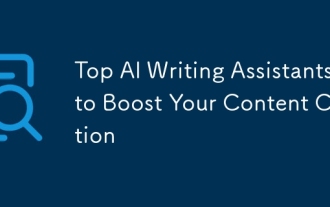
The article discusses top AI writing assistants like Grammarly, Jasper, Copy.ai, Writesonic, and Rytr, focusing on their unique features for content creation. It argues that Jasper excels in SEO optimization, while AI tools help maintain tone consist
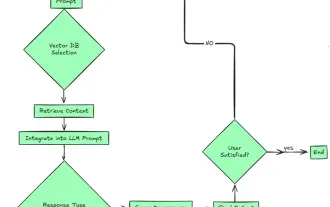
2024 witnessed a shift from simply using LLMs for content generation to understanding their inner workings. This exploration led to the discovery of AI Agents – autonomous systems handling tasks and decisions with minimal human intervention. Buildin
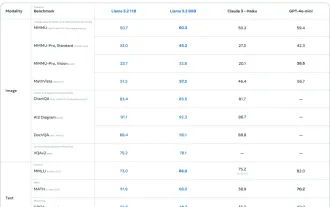
This week's AI landscape: A whirlwind of advancements, ethical considerations, and regulatory debates. Major players like OpenAI, Google, Meta, and Microsoft have unleashed a torrent of updates, from groundbreaking new models to crucial shifts in le
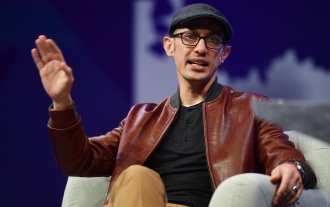
Shopify CEO Tobi Lütke's recent memo boldly declares AI proficiency a fundamental expectation for every employee, marking a significant cultural shift within the company. This isn't a fleeting trend; it's a new operational paradigm integrated into p
