Convert Text Documents to a TF-IDF Matrix with tfidfvectorizer
This article explains the Term Frequency-Inverse Document Frequency (TF-IDF) technique, a crucial tool in Natural Language Processing (NLP) for analyzing textual data. TF-IDF surpasses the limitations of basic bag-of-words approaches by weighting terms based on their frequency within a document and their rarity across a collection of documents. This enhanced weighting improves text classification and boosts the analytical capabilities of machine learning models. We'll demonstrate how to construct a TF-IDF model from scratch in Python and perform numerical calculations.
Table of Contents
- Key Terms in TF-IDF
- Term Frequency (TF) Explained
- Document Frequency (DF) Explained
- Inverse Document Frequency (IDF) Explained
- Understanding TF-IDF
- Numerical TF-IDF Calculation
- Step 1: Calculating Term Frequency (TF)
- Step 2: Calculating Inverse Document Frequency (IDF)
- Step 3: Calculating TF-IDF
- Python Implementation using a Built-in Dataset
- Step 1: Installing Necessary Libraries
- Step 2: Importing Libraries
- Step 3: Loading the Dataset
- Step 4: Initializing
TfidfVectorizer
- Step 5: Fitting and Transforming Documents
- Step 6: Examining the TF-IDF Matrix
- Conclusion
- Frequently Asked Questions
Key Terms in TF-IDF
Before proceeding, let's define key terms:
- t: term (individual word)
- d: document (a set of words)
- N: total number of documents in the corpus
- corpus: the entire collection of documents
Term Frequency (TF) Explained
Term Frequency (TF) quantifies how often a term appears in a specific document. A higher TF indicates greater importance within that document. The formula is:
Document Frequency (DF) Explained
Document Frequency (DF) measures the number of documents within the corpus containing a particular term. Unlike TF, it counts the presence of a term, not its occurrences. The formula is:
DF(t) = Number of documents containing term t
Inverse Document Frequency (IDF) Explained
Inverse Document Frequency (IDF) assesses the informativeness of a word. While TF treats all terms equally, IDF downweights common words (like stop words) and upweights rarer terms. The formula is:
where N is the total number of documents and DF(t) is the number of documents containing term t.
Understanding TF-IDF
TF-IDF combines Term Frequency and Inverse Document Frequency to determine a term's significance within a document relative to the entire corpus. The formula is:
Numerical TF-IDF Calculation
Let's illustrate numerical TF-IDF calculation with example documents:
Documents:
- “The sky is blue.”
- “The sun is bright today.”
- “The sun in the sky is bright.”
- “We can see the shining sun, the bright sun.”
Following the steps outlined in the original text, we calculate TF, IDF, and then TF-IDF for each term in each document. (The detailed calculations are omitted here for brevity, but they mirror the original example.)
Python Implementation using a Built-in Dataset
This section demonstrates TF-IDF calculation using scikit-learn's TfidfVectorizer
and the 20 Newsgroups dataset.
Step 1: Installing Necessary Libraries
pip install scikit-learn
Step 2: Importing Libraries
import pandas as pd from sklearn.datasets import fetch_20newsgroups from sklearn.feature_extraction.text import TfidfVectorizer
Step 3: Loading the Dataset
newsgroups = fetch_20newsgroups(subset='train')
Step 4: Initializing TfidfVectorizer
vectorizer = TfidfVectorizer(stop_words='english', max_features=1000)
Step 5: Fitting and Transforming Documents
tfidf_matrix = vectorizer.fit_transform(newsgroups.data)
Step 6: Examining the TF-IDF Matrix
df_tfidf = pd.DataFrame(tfidf_matrix.toarray(), columns=vectorizer.get_feature_names_out()) df_tfidf.head()
Conclusion
Using the 20 Newsgroups dataset and TfidfVectorizer
, we efficiently transform text documents into a TF-IDF matrix. This matrix represents the importance of each term, enabling various NLP tasks like text classification and clustering. Scikit-learn's TfidfVectorizer
simplifies this process significantly.
Frequently Asked Questions
The FAQs section remains largely unchanged, addressing the logarithmic nature of IDF, scalability to large datasets, limitations of TF-IDF (ignoring word order and context), and common applications (search engines, text classification, clustering, summarization).
The above is the detailed content of Convert Text Documents to a TF-IDF Matrix with tfidfvectorizer. For more information, please follow other related articles on the PHP Chinese website!

Hot AI Tools

Undresser.AI Undress
AI-powered app for creating realistic nude photos

AI Clothes Remover
Online AI tool for removing clothes from photos.

Undress AI Tool
Undress images for free

Clothoff.io
AI clothes remover

Video Face Swap
Swap faces in any video effortlessly with our completely free AI face swap tool!

Hot Article

Hot Tools

Notepad++7.3.1
Easy-to-use and free code editor

SublimeText3 Chinese version
Chinese version, very easy to use

Zend Studio 13.0.1
Powerful PHP integrated development environment

Dreamweaver CS6
Visual web development tools

SublimeText3 Mac version
God-level code editing software (SublimeText3)

Hot Topics
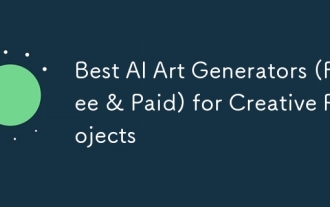
The article reviews top AI art generators, discussing their features, suitability for creative projects, and value. It highlights Midjourney as the best value for professionals and recommends DALL-E 2 for high-quality, customizable art.
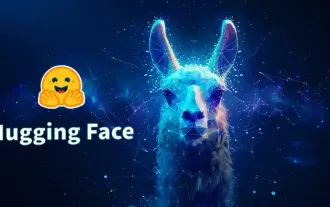
Meta's Llama 3.2: A Leap Forward in Multimodal and Mobile AI Meta recently unveiled Llama 3.2, a significant advancement in AI featuring powerful vision capabilities and lightweight text models optimized for mobile devices. Building on the success o
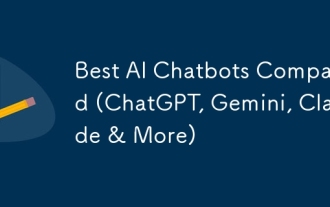
The article compares top AI chatbots like ChatGPT, Gemini, and Claude, focusing on their unique features, customization options, and performance in natural language processing and reliability.
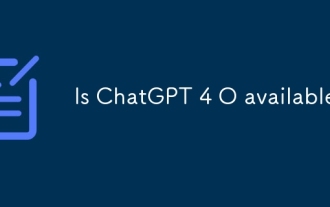
ChatGPT 4 is currently available and widely used, demonstrating significant improvements in understanding context and generating coherent responses compared to its predecessors like ChatGPT 3.5. Future developments may include more personalized interactions and real-time data processing capabilities, further enhancing its potential for various applications.
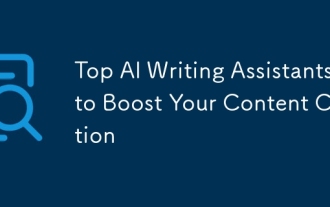
The article discusses top AI writing assistants like Grammarly, Jasper, Copy.ai, Writesonic, and Rytr, focusing on their unique features for content creation. It argues that Jasper excels in SEO optimization, while AI tools help maintain tone consist
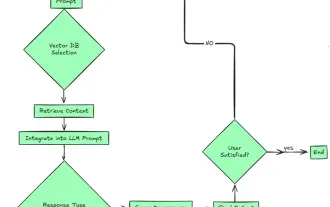
2024 witnessed a shift from simply using LLMs for content generation to understanding their inner workings. This exploration led to the discovery of AI Agents – autonomous systems handling tasks and decisions with minimal human intervention. Buildin
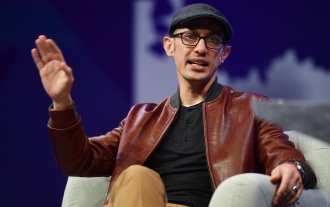
Shopify CEO Tobi Lütke's recent memo boldly declares AI proficiency a fundamental expectation for every employee, marking a significant cultural shift within the company. This isn't a fleeting trend; it's a new operational paradigm integrated into p
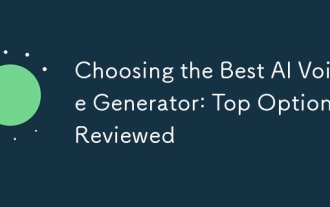
The article reviews top AI voice generators like Google Cloud, Amazon Polly, Microsoft Azure, IBM Watson, and Descript, focusing on their features, voice quality, and suitability for different needs.
