Violin Plots: A Tool for Visualizing Data Distributions
Violin Plots: A Powerful Data Visualization Tool
This article delves into violin plots, a compelling data visualization technique merging box plots and density plots. We'll explore how these plots unveil data patterns, making them invaluable for data scientists and machine learning professionals. This guide offers practical techniques and Python examples to empower informed decisions and effective communication of complex datasets.
Key Learning Outcomes:
- Understand the core components and characteristics of violin plots.
- Differentiate violin plots from box plots and density plots.
- Explore the applications of violin plots in machine learning and data analysis.
- Gain hands-on experience creating and comparing these plots using Python code.
- Recognize the importance of violin plots in exploratory data analysis (EDA) and model assessment.
Table of Contents:
- Introduction
- Understanding Violin Plots
- Applications in Data Analysis and Machine Learning
- Comparing Violin, Box, and Density Plots
- Conclusion
- Frequently Asked Questions
Deciphering Violin Plots:
Violin plots offer a sophisticated way to visualize data by combining box plots and density plots. The foundation lies in kernel density estimation (KDE), a non-parametric method for estimating the probability density function (PDF) of a random variable. KDE smooths data points, providing a continuous representation of the data distribution.
Key KDE concepts include:
- Kernel Function: A function that smooths data points by assigning weights based on distance from a target point. Gaussian kernels are common, but others (linear, Epanechnikov) exist.
- Bandwidth: Controls the smoothness of the KDE. A large bandwidth over-smooths, while a small bandwidth overfits.
- Estimation: KDE involves placing a kernel on each data point and summing them for the overall density estimate. The mathematical representation is shown below:
A violin plot mirrors the KDE on both sides of a central box plot, creating the violin shape. The key components are:
- Central Box Plot: Shows the median and interquartile range (IQR).
- Density Plot: Illustrates data probability density, highlighting high-concentration areas.
- Axes: The x-axis represents categories/groups, and the y-axis shows the data distribution.
Violin plots excel with complex distributions, revealing multi-modality and outliers. However, their complexity might reduce intuitiveness for those unfamiliar with data visualization.
Applications in Data Science and Machine Learning:
Violin plots find use in various scenarios:
- Feature Analysis: Understanding feature distributions, identifying outliers, and comparing distributions across categories.
- Model Evaluation: Comparing predicted and actual values to detect bias and variance.
- Hyperparameter Tuning: Comparing model performance across different hyperparameter settings.
Comparing Visualization Methods:
Let's compare violin, box, and density plots using Seaborn, a popular Python library.
Step 1: Install Libraries
!pip install seaborn matplotlib pandas numpy print('Importing Libraries...',end='') import seaborn as sns import matplotlib.pyplot as plt import pandas as pd import numpy as np print('Done')
Step 2: Generate Synthetic Data
np.random.seed(11) data = pd.DataFrame({ 'Category': np.random.choice(['A', 'B', 'C'], size=100), 'Value': np.random.randn(100) })
Step 3: Data Summary
print(data.head()) print(data.describe(include='all')) print(data['Category'].value_counts()) print(data.isnull().sum())
Step 4: Generate Plots
fig, axes = plt.subplots(1, 3, figsize=(18, 6)) sns.violinplot(x='Category', y='Value', data=data, ax=axes[0]) axes[0].set_title('Violin Plot') sns.boxplot(x='Category', y='Value', data=data, ax=axes[1]) axes[1].set_title('Box Plot') for category in data['Category'].unique(): sns.kdeplot(data[data['Category'] == category]['Value'], label=category, ax=axes[2]) axes[2].set_title('Density Plot') axes[2].legend(title='Category') plt.tight_layout() plt.show()
Output:
Conclusion:
Effective data visualization is crucial in machine learning. Violin plots offer a powerful combination of detail and summary statistics, enhancing feature engineering and selection. Their versatility allows analysis of various data types, revealing hidden patterns and anomalies for improved decision-making and communication.
Key Takeaways:
- Violin plots combine density plot detail and box plot summary statistics.
- They are versatile, working with various data types.
- They assist in feature analysis, model evaluation, and hyperparameter optimization.
- Libraries like Seaborn simplify their creation in Python.
- They effectively communicate complex data distributions.
Frequently Asked Questions:
Q1: How do violin plots aid in feature analysis? A1: They reveal data distribution shapes, highlighting trends and outliers, facilitating feature selection by comparing distributions.
Q2: Can they handle large datasets? A2: Yes, but adjust KDE bandwidth to maintain clarity.
Q3: How to interpret multiple peaks? A3: Multiple peaks indicate distinct data clusters or modes, suggesting subgroups.
Q4: How to customize appearance in Python? A4: Seaborn and Matplotlib offer parameters for color, width, and KDE bandwidth.
(Note: The image used in this article is not owned by [Your Name/Organization] and is used with the author's permission.)
The above is the detailed content of Violin Plots: A Tool for Visualizing Data Distributions. For more information, please follow other related articles on the PHP Chinese website!

Hot AI Tools

Undresser.AI Undress
AI-powered app for creating realistic nude photos

AI Clothes Remover
Online AI tool for removing clothes from photos.

Undress AI Tool
Undress images for free

Clothoff.io
AI clothes remover

Video Face Swap
Swap faces in any video effortlessly with our completely free AI face swap tool!

Hot Article

Hot Tools

Notepad++7.3.1
Easy-to-use and free code editor

SublimeText3 Chinese version
Chinese version, very easy to use

Zend Studio 13.0.1
Powerful PHP integrated development environment

Dreamweaver CS6
Visual web development tools

SublimeText3 Mac version
God-level code editing software (SublimeText3)

Hot Topics
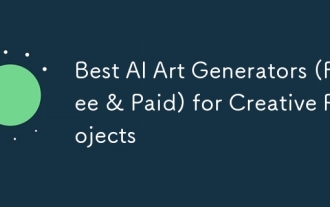
The article reviews top AI art generators, discussing their features, suitability for creative projects, and value. It highlights Midjourney as the best value for professionals and recommends DALL-E 2 for high-quality, customizable art.
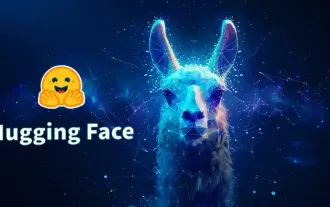
Meta's Llama 3.2: A Leap Forward in Multimodal and Mobile AI Meta recently unveiled Llama 3.2, a significant advancement in AI featuring powerful vision capabilities and lightweight text models optimized for mobile devices. Building on the success o
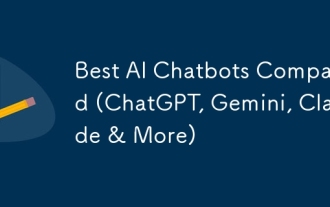
The article compares top AI chatbots like ChatGPT, Gemini, and Claude, focusing on their unique features, customization options, and performance in natural language processing and reliability.
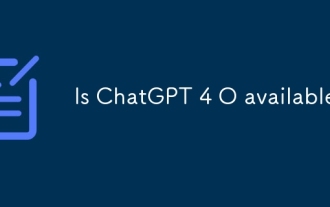
ChatGPT 4 is currently available and widely used, demonstrating significant improvements in understanding context and generating coherent responses compared to its predecessors like ChatGPT 3.5. Future developments may include more personalized interactions and real-time data processing capabilities, further enhancing its potential for various applications.
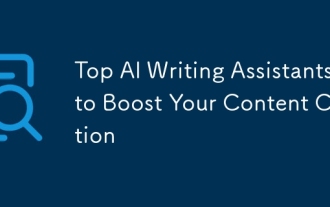
The article discusses top AI writing assistants like Grammarly, Jasper, Copy.ai, Writesonic, and Rytr, focusing on their unique features for content creation. It argues that Jasper excels in SEO optimization, while AI tools help maintain tone consist
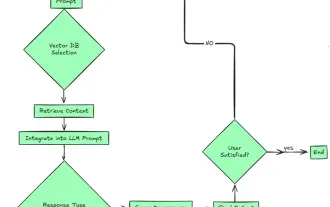
2024 witnessed a shift from simply using LLMs for content generation to understanding their inner workings. This exploration led to the discovery of AI Agents – autonomous systems handling tasks and decisions with minimal human intervention. Buildin
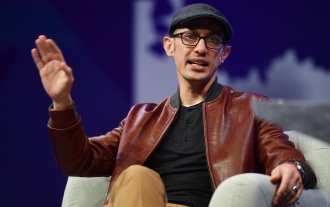
Shopify CEO Tobi Lütke's recent memo boldly declares AI proficiency a fundamental expectation for every employee, marking a significant cultural shift within the company. This isn't a fleeting trend; it's a new operational paradigm integrated into p
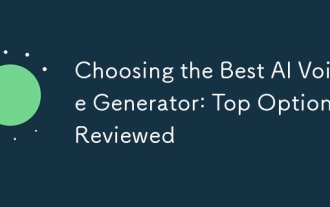
The article reviews top AI voice generators like Google Cloud, Amazon Polly, Microsoft Azure, IBM Watson, and Descript, focusing on their features, voice quality, and suitability for different needs.
