


How do you handle scalability challenges in Python applications?
How do you handle scalability challenges in Python applications?
Handling scalability challenges in Python applications involves a multi-faceted approach that addresses both the code and the infrastructure. Here are some key strategies:
- Code Optimization: Ensuring that the Python code is efficient and optimized is crucial. This includes using appropriate data structures, minimizing loops, and leveraging built-in functions and libraries that are optimized for performance.
-
Caching: Implementing caching mechanisms can significantly reduce the load on your application by storing the results of expensive operations and reusing them when needed. Python offers various caching libraries like
dogpile.cache
andRedis
. - Database Optimization: Efficient database queries and indexing can greatly improve the performance of your application. Consider using ORM tools like SQLAlchemy with care to avoid generating inefficient queries.
- Load Balancing: Distributing incoming requests across multiple servers can help manage high traffic. Tools like Nginx can be used to set up load balancing.
-
Asynchronous Programming: Using asynchronous programming models, such as those provided by
asyncio
, can help handle a large number of concurrent connections more efficiently. - Horizontal Scaling: Adding more machines to your infrastructure to handle increased load. This can be facilitated by containerization technologies like Docker and orchestration tools like Kubernetes.
- Microservices Architecture: Breaking down your application into smaller, independent services can improve scalability by allowing each service to scale independently based on demand.
By implementing these strategies, you can effectively manage and improve the scalability of your Python applications.
What are the best practices for optimizing Python code to improve scalability?
Optimizing Python code for scalability involves several best practices that can significantly enhance the performance and efficiency of your applications. Here are some key practices:
- Use Appropriate Data Structures: Choose the right data structure for your task. For example, use sets for membership testing and dictionaries for fast lookups.
-
Avoid Unnecessary Loops: Minimize the use of loops where possible. Use list comprehensions, generator expressions, or built-in functions like
map()
,filter()
, andreduce()
to process data more efficiently. - Leverage Built-in Functions and Libraries: Python's built-in functions and standard libraries are often optimized for performance. Use them instead of writing custom implementations.
-
Profile Your Code: Use profiling tools like
cProfile
orline_profiler
to identify bottlenecks in your code. This helps you focus your optimization efforts on the parts of the code that need it most. - Use Cython or Numba for Performance-Critical Code: For computationally intensive parts of your code, consider using Cython or Numba to compile Python code to C, which can significantly improve performance.
-
Implement Caching: Use caching to store the results of expensive operations. Libraries like
functools.lru_cache
can be used for simple caching needs. - Optimize Database Queries: Ensure that your database queries are efficient. Use indexing, avoid N 1 query problems, and consider using database-specific optimizations.
-
Asynchronous Programming: Use asynchronous programming techniques to handle I/O-bound operations more efficiently. Libraries like
asyncio
can help manage concurrent operations without blocking.
By following these best practices, you can optimize your Python code to improve its scalability and performance.
How can asynchronous programming in Python help with handling high loads?
Asynchronous programming in Python can significantly help with handling high loads by allowing your application to manage multiple tasks concurrently without blocking. Here's how it works and its benefits:
- Non-Blocking I/O Operations: Asynchronous programming allows your application to perform I/O operations (like reading from a database or making an API call) without waiting for the operation to complete. This means your application can continue processing other tasks while waiting for I/O operations to finish.
- Efficient Resource Utilization: By not blocking on I/O operations, asynchronous programming allows your application to make better use of system resources. This is particularly beneficial for handling a large number of concurrent connections, as it can keep more connections active at the same time.
- Scalability: Asynchronous programming can help your application scale more efficiently. Since it can handle more concurrent operations with fewer resources, you can serve more users without needing to add more hardware.
- Improved Responsiveness: Applications using asynchronous programming tend to be more responsive, as they can quickly switch between tasks and handle user requests more efficiently.
-
Use of
asyncio
: Python'sasyncio
library provides a robust framework for writing asynchronous code. It allows you to define coroutines usingasync
andawait
keywords, making it easier to write and maintain asynchronous code. -
Asynchronous Web Frameworks: Frameworks like
aiohttp
andFastAPI
leverage asynchronous programming to build scalable web applications. These frameworks can handle a high number of concurrent requests efficiently.
By leveraging asynchronous programming, you can build Python applications that are better equipped to handle high loads and scale more effectively.
What tools and frameworks can be used to monitor and manage scalability in Python applications?
Monitoring and managing scalability in Python applications requires the use of various tools and frameworks that can help you track performance, identify bottlenecks, and scale your application effectively. Here are some key tools and frameworks:
-
Monitoring Tools:
- Prometheus: An open-source monitoring and alerting toolkit that can be used to collect metrics from your Python applications. It integrates well with Grafana for visualization.
- New Relic: A comprehensive monitoring tool that provides detailed insights into your application's performance, including response times, throughput, and error rates.
- Datadog: Offers real-time monitoring and analytics for your applications, with support for custom metrics and dashboards.
-
Logging and Tracing:
- ELK Stack (Elasticsearch, Logstash, Kibana): A powerful combination for log analysis and visualization. It can help you track and analyze logs from your Python applications.
- Jaeger: An open-source, end-to-end distributed tracing system that can help you understand the flow of requests through your application and identify performance bottlenecks.
-
Profiling Tools:
- cProfile: A built-in Python profiler that can help you identify which parts of your code are consuming the most time.
- line_profiler: A more detailed profiler that can show you the time spent on each line of your code.
-
Load Testing Tools:
- Locust: An open-source load testing tool that allows you to define user behavior in Python code and simulate thousands of concurrent users.
- Apache JMeter: A popular open-source tool for load testing and performance measurement.
-
Containerization and Orchestration:
- Docker: Allows you to containerize your Python applications, making it easier to deploy and scale them.
- Kubernetes: An orchestration platform that can manage the deployment, scaling, and operation of containerized applications.
-
Scalability Frameworks:
- Celery: A distributed task queue that can help you offload and manage background tasks, improving the scalability of your application.
- Gunicorn: A WSGI HTTP server for Unix that can be used to run Python web applications, with support for multiple workers to handle concurrent requests.
By using these tools and frameworks, you can effectively monitor and manage the scalability of your Python applications, ensuring they can handle increased loads and perform optimally.
The above is the detailed content of How do you handle scalability challenges in Python applications?. For more information, please follow other related articles on the PHP Chinese website!

Hot AI Tools

Undresser.AI Undress
AI-powered app for creating realistic nude photos

AI Clothes Remover
Online AI tool for removing clothes from photos.

Undress AI Tool
Undress images for free

Clothoff.io
AI clothes remover

Video Face Swap
Swap faces in any video effortlessly with our completely free AI face swap tool!

Hot Article

Hot Tools

Notepad++7.3.1
Easy-to-use and free code editor

SublimeText3 Chinese version
Chinese version, very easy to use

Zend Studio 13.0.1
Powerful PHP integrated development environment

Dreamweaver CS6
Visual web development tools

SublimeText3 Mac version
God-level code editing software (SublimeText3)

Hot Topics










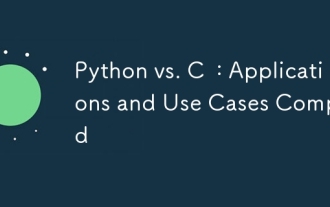
Python is suitable for data science, web development and automation tasks, while C is suitable for system programming, game development and embedded systems. Python is known for its simplicity and powerful ecosystem, while C is known for its high performance and underlying control capabilities.
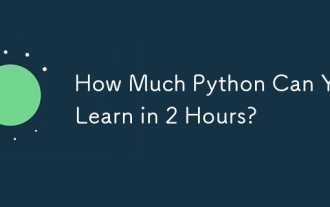
You can learn the basics of Python within two hours. 1. Learn variables and data types, 2. Master control structures such as if statements and loops, 3. Understand the definition and use of functions. These will help you start writing simple Python programs.
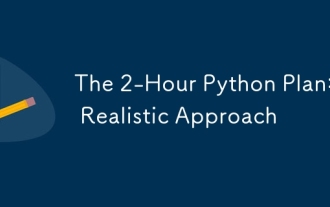
You can learn basic programming concepts and skills of Python within 2 hours. 1. Learn variables and data types, 2. Master control flow (conditional statements and loops), 3. Understand the definition and use of functions, 4. Quickly get started with Python programming through simple examples and code snippets.
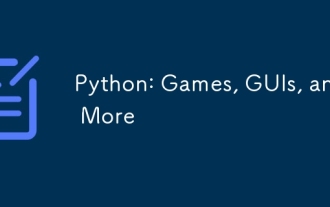
Python excels in gaming and GUI development. 1) Game development uses Pygame, providing drawing, audio and other functions, which are suitable for creating 2D games. 2) GUI development can choose Tkinter or PyQt. Tkinter is simple and easy to use, PyQt has rich functions and is suitable for professional development.
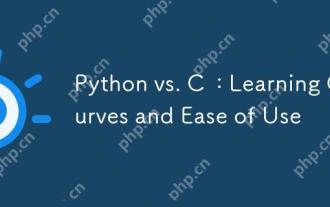
Python is easier to learn and use, while C is more powerful but complex. 1. Python syntax is concise and suitable for beginners. Dynamic typing and automatic memory management make it easy to use, but may cause runtime errors. 2.C provides low-level control and advanced features, suitable for high-performance applications, but has a high learning threshold and requires manual memory and type safety management.
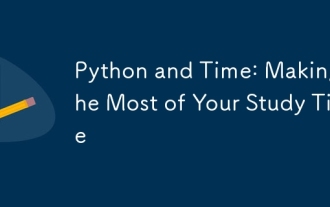
To maximize the efficiency of learning Python in a limited time, you can use Python's datetime, time, and schedule modules. 1. The datetime module is used to record and plan learning time. 2. The time module helps to set study and rest time. 3. The schedule module automatically arranges weekly learning tasks.
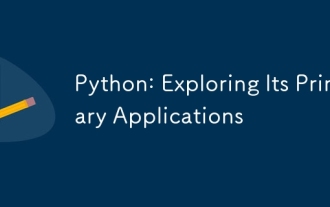
Python is widely used in the fields of web development, data science, machine learning, automation and scripting. 1) In web development, Django and Flask frameworks simplify the development process. 2) In the fields of data science and machine learning, NumPy, Pandas, Scikit-learn and TensorFlow libraries provide strong support. 3) In terms of automation and scripting, Python is suitable for tasks such as automated testing and system management.
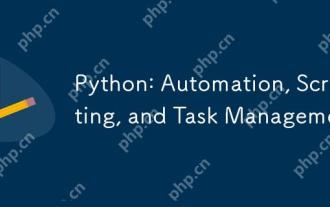
Python excels in automation, scripting, and task management. 1) Automation: File backup is realized through standard libraries such as os and shutil. 2) Script writing: Use the psutil library to monitor system resources. 3) Task management: Use the schedule library to schedule tasks. Python's ease of use and rich library support makes it the preferred tool in these areas.
