


How do you approach designing a new system or feature in Python?
How do you approach designing a new system or feature in Python?
When designing a new system or feature in Python, I follow a structured approach to ensure that the end result is both functional and maintainable. Here are the steps I typically take:
- Define Requirements: The first step is to clearly define the requirements of the system or feature. This involves understanding the problem domain, identifying the key functionalities, and setting clear objectives. I often use user stories or requirement documents to capture these details.
- Research and Planning: Once the requirements are clear, I conduct research to understand existing solutions, best practices, and any relevant libraries or frameworks that could be utilized. This phase also involves sketching out high-level designs and planning the overall architecture.
- Prototyping: I create a prototype to test the feasibility of the design. This could be a simple script or a more complex mock-up, depending on the complexity of the system. Prototyping helps in identifying potential issues early in the development process.
- Detailed Design: With the prototype in hand, I move on to the detailed design phase. This involves creating detailed diagrams (such as UML diagrams), defining the data structures, and outlining the algorithms to be used. I also consider the modularity of the code and how different components will interact.
- Implementation: The actual coding begins once the design is finalized. I follow best practices such as writing clean, modular code, and adhering to PEP 8 style guidelines. I also ensure that the code is well-documented and includes appropriate comments.
- Testing and Refinement: After the initial implementation, I conduct thorough testing to ensure that the system or feature meets the defined requirements. This includes unit testing, integration testing, and possibly user acceptance testing. Based on the test results, I refine the design and implementation as needed.
- Review and Iteration: Finally, I conduct a review of the entire process, gather feedback, and iterate on the design if necessary. This iterative approach helps in continuously improving the system or feature.
What are the key considerations when planning the architecture of a Python project?
When planning the architecture of a Python project, several key considerations come into play:
- Scalability: The architecture should be designed to handle growth in terms of data volume, user base, and functionality. This might involve using scalable data storage solutions, implementing efficient algorithms, and designing for horizontal scaling.
- Modularity: A modular architecture allows for easier maintenance and updates. This can be achieved by breaking down the system into smaller, independent components or modules that can be developed, tested, and maintained separately.
- Reusability: Designing for reusability helps in reducing redundancy and improving efficiency. This involves creating reusable components and libraries that can be used across different parts of the project or even in other projects.
- Performance: The architecture should be optimized for performance, considering factors such as response times, resource utilization, and throughput. This might involve choosing the right data structures, algorithms, and possibly using asynchronous programming techniques.
- Security: Security considerations are crucial, especially for systems that handle sensitive data. This includes implementing proper authentication and authorization mechanisms, securing data at rest and in transit, and following security best practices.
- Maintainability: The architecture should be easy to maintain and update. This involves writing clean, well-documented code, following design patterns, and using tools that support code quality and maintainability.
- Integration: Consider how the system will integrate with other systems or services. This might involve designing APIs, using microservices architecture, or ensuring compatibility with existing infrastructure.
- Testing: The architecture should facilitate testing, including unit testing, integration testing, and possibly automated testing. This involves designing the system in a way that makes it easy to isolate and test individual components.
How do you ensure your Python code remains maintainable and scalable as the project grows?
Ensuring that Python code remains maintainable and scalable as the project grows involves several strategies:
- Adherence to Best Practices: Following best practices such as writing clean, modular code, adhering to PEP 8 style guidelines, and using meaningful variable and function names helps in maintaining code quality.
- Modular Design: Breaking down the system into smaller, independent modules makes it easier to maintain and update individual components without affecting the entire system. This also facilitates parallel development and testing.
- Documentation: Writing comprehensive documentation, including docstrings and comments, helps other developers understand the code and its purpose. This is crucial for maintaining the codebase over time.
- Code Reviews: Regular code reviews help in identifying and fixing issues early, ensuring that the code adheres to the project's standards and best practices. This also promotes knowledge sharing among team members.
- Refactoring: Regularly refactoring the code to improve its structure and efficiency helps in keeping the codebase clean and maintainable. This involves removing redundant code, simplifying complex logic, and optimizing performance.
- Testing: Implementing a robust testing strategy, including unit tests, integration tests, and possibly automated tests, ensures that changes to the code do not introduce new bugs. This also helps in maintaining the scalability of the system.
- Continuous Integration and Deployment (CI/CD): Using CI/CD pipelines helps in automating the testing and deployment process, ensuring that changes are thoroughly tested before being deployed to production. This also helps in maintaining the scalability of the system.
- Performance Monitoring: Regularly monitoring the performance of the system helps in identifying bottlenecks and areas for improvement. This involves using tools to track metrics such as response times, resource utilization, and throughput.
What tools or methodologies do you use to test and refine your Python designs during development?
To test and refine Python designs during development, I use a combination of tools and methodologies:
-
Unit Testing: I use the
unittest
module or third-party frameworks likepytest
to write and run unit tests. Unit tests help in verifying that individual components of the system work as expected. -
Integration Testing: For testing how different components interact, I use integration tests. This can be done using frameworks like
pytest
with plugins such aspytest-django
for Django projects. - Automated Testing: I set up automated testing pipelines using tools like Jenkins, Travis CI, or GitHub Actions. These pipelines run tests automatically whenever code changes are pushed to the repository, ensuring that the system remains stable.
-
Code Coverage Tools: I use tools like
coverage.py
to measure the code coverage of my tests. This helps in identifying areas of the code that are not adequately tested and need more attention. -
Static Code Analysis: Tools like
pylint
,flake8
, andmypy
help in identifying potential issues in the code, such as style violations, bugs, and type errors. These tools help in maintaining code quality and catching issues early. -
Profiling and Performance Testing: For performance testing, I use tools like
cProfile
orline_profiler
to identify bottlenecks and optimize the code. This helps in refining the design to improve performance. - User Acceptance Testing (UAT): For systems that involve user interaction, I conduct UAT to ensure that the system meets the user's needs and expectations. This involves creating test scenarios and getting feedback from actual users.
- Agile Methodologies: I follow agile methodologies such as Scrum or Kanban to iteratively develop and refine the design. This involves regular sprints, stand-ups, and retrospectives to continuously improve the system.
- Design Patterns and Refactoring: I use design patterns and refactoring techniques to improve the design of the system. This involves applying patterns like Singleton, Factory, or Observer to solve common design problems and refactoring the code to improve its structure and efficiency.
By combining these tools and methodologies, I ensure that the Python designs are thoroughly tested and refined during development, leading to a robust and maintainable system.
The above is the detailed content of How do you approach designing a new system or feature in Python?. For more information, please follow other related articles on the PHP Chinese website!

Hot AI Tools

Undresser.AI Undress
AI-powered app for creating realistic nude photos

AI Clothes Remover
Online AI tool for removing clothes from photos.

Undress AI Tool
Undress images for free

Clothoff.io
AI clothes remover

Video Face Swap
Swap faces in any video effortlessly with our completely free AI face swap tool!

Hot Article

Hot Tools

Notepad++7.3.1
Easy-to-use and free code editor

SublimeText3 Chinese version
Chinese version, very easy to use

Zend Studio 13.0.1
Powerful PHP integrated development environment

Dreamweaver CS6
Visual web development tools

SublimeText3 Mac version
God-level code editing software (SublimeText3)

Hot Topics










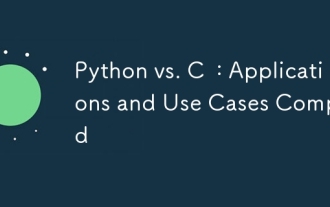
Python is suitable for data science, web development and automation tasks, while C is suitable for system programming, game development and embedded systems. Python is known for its simplicity and powerful ecosystem, while C is known for its high performance and underlying control capabilities.
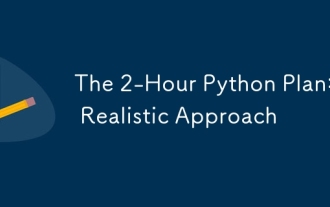
You can learn basic programming concepts and skills of Python within 2 hours. 1. Learn variables and data types, 2. Master control flow (conditional statements and loops), 3. Understand the definition and use of functions, 4. Quickly get started with Python programming through simple examples and code snippets.
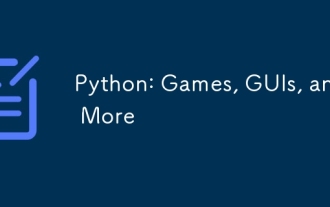
Python excels in gaming and GUI development. 1) Game development uses Pygame, providing drawing, audio and other functions, which are suitable for creating 2D games. 2) GUI development can choose Tkinter or PyQt. Tkinter is simple and easy to use, PyQt has rich functions and is suitable for professional development.
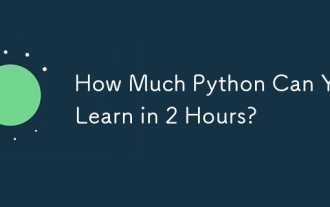
You can learn the basics of Python within two hours. 1. Learn variables and data types, 2. Master control structures such as if statements and loops, 3. Understand the definition and use of functions. These will help you start writing simple Python programs.
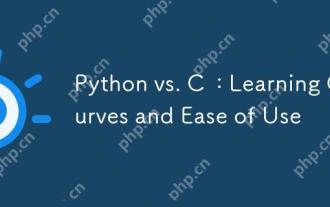
Python is easier to learn and use, while C is more powerful but complex. 1. Python syntax is concise and suitable for beginners. Dynamic typing and automatic memory management make it easy to use, but may cause runtime errors. 2.C provides low-level control and advanced features, suitable for high-performance applications, but has a high learning threshold and requires manual memory and type safety management.
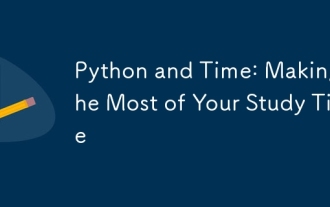
To maximize the efficiency of learning Python in a limited time, you can use Python's datetime, time, and schedule modules. 1. The datetime module is used to record and plan learning time. 2. The time module helps to set study and rest time. 3. The schedule module automatically arranges weekly learning tasks.
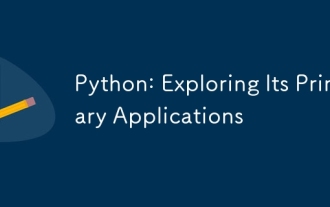
Python is widely used in the fields of web development, data science, machine learning, automation and scripting. 1) In web development, Django and Flask frameworks simplify the development process. 2) In the fields of data science and machine learning, NumPy, Pandas, Scikit-learn and TensorFlow libraries provide strong support. 3) In terms of automation and scripting, Python is suitable for tasks such as automated testing and system management.
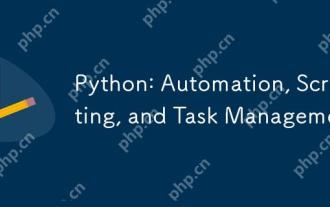
Python excels in automation, scripting, and task management. 1) Automation: File backup is realized through standard libraries such as os and shutil. 2) Script writing: Use the psutil library to monitor system resources. 3) Task management: Use the schedule library to schedule tasks. Python's ease of use and rich library support makes it the preferred tool in these areas.
