


Explain the concepts of supervised learning, unsupervised learning, and reinforcement learning.
Explain the concepts of supervised learning, unsupervised learning, and reinforcement learning.
Supervised Learning:
Supervised learning is a type of machine learning where the algorithm is trained on a labeled dataset, meaning that the input data is accompanied by the correct output or label. The goal of supervised learning is to learn a function that maps input to output based on example input-output pairs. It can be divided into two types: classification and regression. In classification, the output is a category or class label, while in regression, the output is a continuous value. Common algorithms used in supervised learning include decision trees, support vector machines, and neural networks.
Unsupervised Learning:
Unsupervised learning, on the other hand, deals with unlabeled data. The goal here is to find hidden patterns or intrinsic structures in the input data without any explicit guidance on what the output should be. It is often used for exploratory data analysis, clustering, and dimensionality reduction. Common unsupervised learning techniques include k-means clustering, hierarchical clustering, and principal component analysis (PCA).
Reinforcement Learning:
Reinforcement learning (RL) is a type of machine learning where an agent learns to make decisions by performing actions in an environment to achieve a goal. The agent receives rewards or penalties based on the actions it takes, and the goal is to maximize the total reward over time. Unlike supervised learning, there is no labeled data to learn from, and unlike unsupervised learning, there is a clear objective (maximizing the reward). RL is used in various applications such as game playing, robotics, and autonomous driving. Common algorithms in RL include Q-learning and Deep Q-Networks (DQN).
What are the key differences between supervised and unsupervised learning algorithms?
The key differences between supervised and unsupervised learning algorithms revolve around the nature of the data and the learning objectives:
-
Data Labeling:
- Supervised Learning: Uses labeled data, where each input is associated with a known output or target.
- Unsupervised Learning: Uses unlabeled data, focusing on discovering patterns or structures without prior knowledge of the output.
-
Learning Objective:
- Supervised Learning: The objective is to predict the output based on the input, typically by learning a mapping function from input to output.
- Unsupervised Learning: The objective is to find underlying patterns or groupings in the data, often used for clustering or dimensionality reduction.
-
Examples and Applications:
- Supervised Learning: Used for tasks like email spam detection (classification) or house price prediction (regression).
- Unsupervised Learning: Applied in customer segmentation (clustering) or image compression (dimensionality reduction).
-
Performance Measurement:
- Supervised Learning: Performance is typically measured by accuracy, precision, recall, or mean squared error, depending on the task.
- Unsupervised Learning: Performance can be harder to measure and often involves subjective evaluation or specific metrics like the silhouette score for clustering.
How does reinforcement learning differ from traditional supervised and unsupervised learning methods?
Reinforcement learning (RL) differs from traditional supervised and unsupervised learning methods in several key ways:
-
Learning Mechanism:
- Supervised Learning: Learns from a fixed dataset of input-output pairs.
- Unsupervised Learning: Learns from data without any explicit output or labels.
- Reinforcement Learning: Learns by interacting with an environment and receiving feedback in the form of rewards or penalties.
-
Objective:
- Supervised Learning: The objective is to minimize the error between predicted and actual outputs.
- Unsupervised Learning: The objective is to discover hidden structures or patterns in the data.
- Reinforcement Learning: The objective is to maximize a cumulative reward over time through a sequence of actions.
-
Feedback and Interaction:
- Supervised Learning: Feedback is immediate and provided in the form of labeled data.
- Unsupervised Learning: There is no direct feedback; the algorithm explores the data on its own.
- Reinforcement Learning: Feedback is delayed and comes in the form of rewards or penalties after taking actions in an environment.
-
Use Cases:
- Supervised Learning: Typically used for tasks where the output is known, such as image classification or regression.
- Unsupervised Learning: Used for exploratory data analysis, clustering, and finding latent features in data.
- Reinforcement Learning: Often used for decision-making tasks in dynamic environments, such as game playing, robotics, and autonomous driving.
Can you provide examples of real-world applications for each type of machine learning?
Supervised Learning:
-
Email Spam Detection:
- Supervised learning is used to classify emails as spam or not spam based on historical data of labeled emails. Algorithms like Naive Bayes or Support Vector Machines are commonly used for this purpose.
-
Medical Diagnosis:
- Supervised learning models can predict whether a patient has a particular disease based on their medical history and test results. For example, logistic regression can be used to predict the likelihood of diabetes.
-
Stock Price Prediction:
- Regression models can be trained to predict future stock prices based on historical data, using features like past prices, trading volumes, and economic indicators.
Unsupervised Learning:
-
Customer Segmentation:
- Companies use unsupervised learning, such as k-means clustering, to group customers into segments based on their purchasing behavior, demographics, and other features. This helps in targeted marketing and product recommendations.
-
Anomaly Detection:
- Unsupervised learning can be used to detect unusual patterns or anomalies in data, such as fraudulent credit card transactions or network intrusions. Techniques like Isolation Forests or One-Class SVM are commonly used.
-
Image Compression:
- Principal Component Analysis (PCA) can be used to reduce the dimensionality of image data, thereby compressing the images while retaining most of the important information.
Reinforcement Learning:
-
Game Playing:
- RL has been successfully used to train agents to play complex games like Go, Chess, and video games. For example, AlphaGo used reinforcement learning to defeat world champions in Go.
-
Robotics:
- RL is used to train robots to perform tasks such as grasping objects or navigating through environments. The robot learns by trial and error, receiving rewards for successful actions.
-
Autonomous Driving:
- RL can be used to train autonomous vehicles to make driving decisions in real-time, such as when to change lanes or how to navigate through traffic, by maximizing a reward function based on safety and efficiency.
The above is the detailed content of Explain the concepts of supervised learning, unsupervised learning, and reinforcement learning.. For more information, please follow other related articles on the PHP Chinese website!

Hot AI Tools

Undresser.AI Undress
AI-powered app for creating realistic nude photos

AI Clothes Remover
Online AI tool for removing clothes from photos.

Undress AI Tool
Undress images for free

Clothoff.io
AI clothes remover

Video Face Swap
Swap faces in any video effortlessly with our completely free AI face swap tool!

Hot Article

Hot Tools

Notepad++7.3.1
Easy-to-use and free code editor

SublimeText3 Chinese version
Chinese version, very easy to use

Zend Studio 13.0.1
Powerful PHP integrated development environment

Dreamweaver CS6
Visual web development tools

SublimeText3 Mac version
God-level code editing software (SublimeText3)

Hot Topics










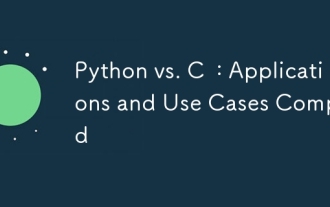
Python is suitable for data science, web development and automation tasks, while C is suitable for system programming, game development and embedded systems. Python is known for its simplicity and powerful ecosystem, while C is known for its high performance and underlying control capabilities.
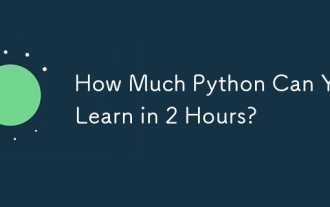
You can learn the basics of Python within two hours. 1. Learn variables and data types, 2. Master control structures such as if statements and loops, 3. Understand the definition and use of functions. These will help you start writing simple Python programs.
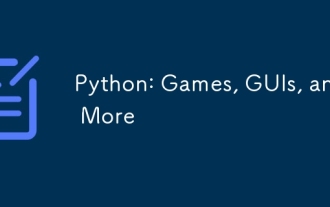
Python excels in gaming and GUI development. 1) Game development uses Pygame, providing drawing, audio and other functions, which are suitable for creating 2D games. 2) GUI development can choose Tkinter or PyQt. Tkinter is simple and easy to use, PyQt has rich functions and is suitable for professional development.
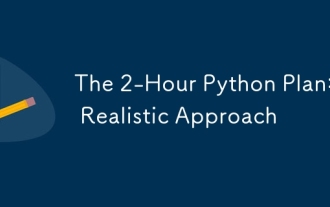
You can learn basic programming concepts and skills of Python within 2 hours. 1. Learn variables and data types, 2. Master control flow (conditional statements and loops), 3. Understand the definition and use of functions, 4. Quickly get started with Python programming through simple examples and code snippets.
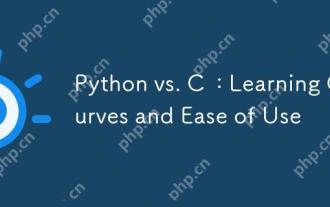
Python is easier to learn and use, while C is more powerful but complex. 1. Python syntax is concise and suitable for beginners. Dynamic typing and automatic memory management make it easy to use, but may cause runtime errors. 2.C provides low-level control and advanced features, suitable for high-performance applications, but has a high learning threshold and requires manual memory and type safety management.
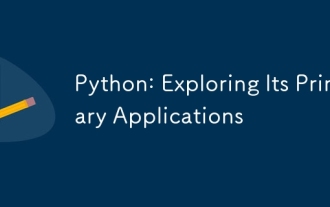
Python is widely used in the fields of web development, data science, machine learning, automation and scripting. 1) In web development, Django and Flask frameworks simplify the development process. 2) In the fields of data science and machine learning, NumPy, Pandas, Scikit-learn and TensorFlow libraries provide strong support. 3) In terms of automation and scripting, Python is suitable for tasks such as automated testing and system management.
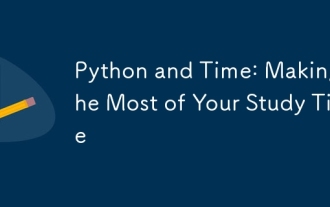
To maximize the efficiency of learning Python in a limited time, you can use Python's datetime, time, and schedule modules. 1. The datetime module is used to record and plan learning time. 2. The time module helps to set study and rest time. 3. The schedule module automatically arranges weekly learning tasks.
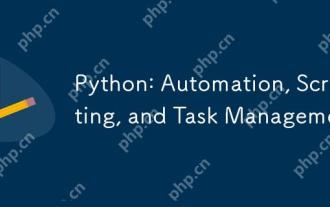
Python excels in automation, scripting, and task management. 1) Automation: File backup is realized through standard libraries such as os and shutil. 2) Script writing: Use the psutil library to monitor system resources. 3) Task management: Use the schedule library to schedule tasks. Python's ease of use and rich library support makes it the preferred tool in these areas.
