What are mocking and patching in Python?
What are mocking and patching in Python?
In Python, mocking and patching are advanced techniques used predominantly in unit testing to isolate components or dependencies of a program for better and more focused testing. Mocking involves creating mock objects that mimic the behavior of real objects in a controlled way, which is particularly useful when the real objects are either difficult to create or slow to use in a testing environment. The unittest.mock
module in Python's standard library provides a powerful framework for creating mock objects.
Patching, on the other hand, is a specific type of mocking that involves temporarily replacing an object or a module's attribute during the test execution. This is typically used to replace dependencies with mock objects. The unittest.mock.patch
decorator or context manager is commonly used for this purpose. Patching allows you to alter the behavior of imported modules and classes during the test, without modifying the code being tested.
How can mocking improve the efficiency of unit testing in Python?
Mocking can significantly improve the efficiency of unit testing in several ways:
- Isolation of Dependencies: Mocking helps isolate the unit being tested from its dependencies, which might be external services, databases, or other parts of the application. This isolation speeds up tests by avoiding the overhead of setting up and tearing down these dependencies for each test run.
- Speed and Consistency: By using mocks, tests can run faster because they no longer need to wait for slow or unpredictable systems (like network requests or database queries). Moreover, mocks ensure that tests are consistent and repeatable, as they always behave the same way.
- Test Complex Scenarios: Mocking allows testers to easily simulate complex scenarios or error conditions that would be difficult to replicate with real objects. For example, you can easily mock a service to return an error or a specific value to test how the system handles these scenarios.
- Focus on Code Logic: With dependencies mocked out, the tester can focus more directly on the logic of the unit being tested. This makes it easier to identify issues within the code itself rather than problems caused by external factors.
What are some common use cases for patching in Python development?
Patching in Python development is commonly used in the following scenarios:
- Testing External APIs: When testing code that interacts with external APIs, patching can be used to mock the API responses, allowing the test to run quickly and reliably without actual network calls.
- Replacing Modules: If a module used by the code under test has side effects or depends on external resources, you can patch the entire module or specific functions within it to control its behavior during tests.
-
Testing Time-Sensitive Code: Patching can be used to control the time (e.g., using
unittest.mock.patch
ontime.time()
ordatetime.datetime.now()
) to ensure that tests involving time-dependent logic work as expected. - Configuration and Environment Variables: Patching is useful for testing how code behaves with different configurations or environment variables, allowing you to change these values during the test.
- Error Handling: By patching methods to raise exceptions, you can test error handling paths in your code more effectively.
What are the best practices for using mocking and patching together in Python?
To use mocking and patching effectively together in Python, consider the following best practices:
- Use Mocking and Patching Sparingly: Only mock and patch what is absolutely necessary. Overuse can lead to tests that do not accurately reflect how the code will work in a real environment.
- Keep Tests Readable and Maintainable: Ensure that the use of mocks and patches does not make your tests harder to understand. Use clear and descriptive names for your mocks, and add comments or docstrings to explain complex patching scenarios.
- Test Real Interactions Where Possible: While mocking can simplify testing, it's beneficial to have some integration tests that test real interactions between components. This ensures that the mocks accurately reflect real-world behavior.
- Mock the Right Level: Prefer to mock at the smallest possible level (e.g., individual methods or functions rather than entire classes or modules) to keep your tests focused and easier to maintain.
- Verify Mock Behavior: Use assertions to verify that your mocks are being used as expected. This could include checking that a method was called with certain arguments or a certain number of times.
-
Use Context Managers or Decorators Appropriately: When using
patch
, choose between the context manager and decorator forms based on readability and the scope of the patch. Context managers (with patch(...)
) are great for short-lived patches, while decorators (@patch(...)
) can be more suitable for function-scoped patches. -
Clean Up: Ensure that any patches applied during a test are cleaned up properly after the test finishes, typically handled automatically by the
patch
decorator or context manager.
By following these best practices, you can leverage the power of mocking and patching to create efficient, effective, and reliable unit tests in Python.
The above is the detailed content of What are mocking and patching in Python?. For more information, please follow other related articles on the PHP Chinese website!

Hot AI Tools

Undresser.AI Undress
AI-powered app for creating realistic nude photos

AI Clothes Remover
Online AI tool for removing clothes from photos.

Undress AI Tool
Undress images for free

Clothoff.io
AI clothes remover

Video Face Swap
Swap faces in any video effortlessly with our completely free AI face swap tool!

Hot Article

Hot Tools

Notepad++7.3.1
Easy-to-use and free code editor

SublimeText3 Chinese version
Chinese version, very easy to use

Zend Studio 13.0.1
Powerful PHP integrated development environment

Dreamweaver CS6
Visual web development tools

SublimeText3 Mac version
God-level code editing software (SublimeText3)

Hot Topics










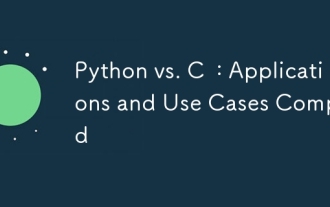
Python is suitable for data science, web development and automation tasks, while C is suitable for system programming, game development and embedded systems. Python is known for its simplicity and powerful ecosystem, while C is known for its high performance and underlying control capabilities.
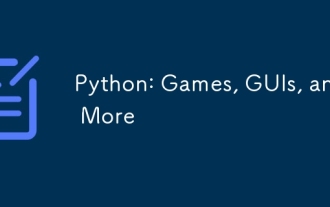
Python excels in gaming and GUI development. 1) Game development uses Pygame, providing drawing, audio and other functions, which are suitable for creating 2D games. 2) GUI development can choose Tkinter or PyQt. Tkinter is simple and easy to use, PyQt has rich functions and is suitable for professional development.
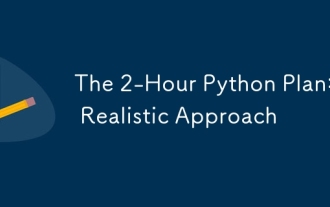
You can learn basic programming concepts and skills of Python within 2 hours. 1. Learn variables and data types, 2. Master control flow (conditional statements and loops), 3. Understand the definition and use of functions, 4. Quickly get started with Python programming through simple examples and code snippets.
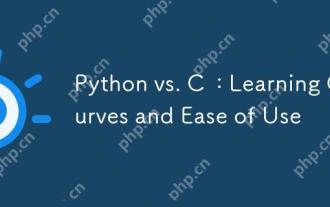
Python is easier to learn and use, while C is more powerful but complex. 1. Python syntax is concise and suitable for beginners. Dynamic typing and automatic memory management make it easy to use, but may cause runtime errors. 2.C provides low-level control and advanced features, suitable for high-performance applications, but has a high learning threshold and requires manual memory and type safety management.
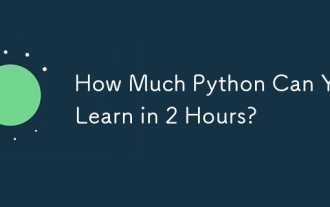
You can learn the basics of Python within two hours. 1. Learn variables and data types, 2. Master control structures such as if statements and loops, 3. Understand the definition and use of functions. These will help you start writing simple Python programs.
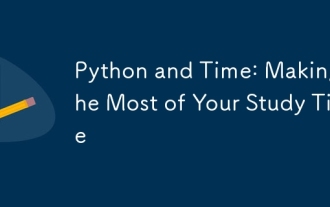
To maximize the efficiency of learning Python in a limited time, you can use Python's datetime, time, and schedule modules. 1. The datetime module is used to record and plan learning time. 2. The time module helps to set study and rest time. 3. The schedule module automatically arranges weekly learning tasks.
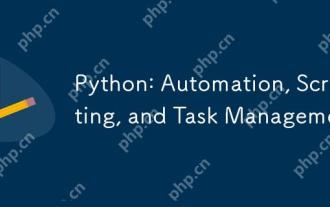
Python excels in automation, scripting, and task management. 1) Automation: File backup is realized through standard libraries such as os and shutil. 2) Script writing: Use the psutil library to monitor system resources. 3) Task management: Use the schedule library to schedule tasks. Python's ease of use and rich library support makes it the preferred tool in these areas.
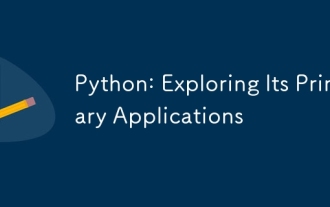
Python is widely used in the fields of web development, data science, machine learning, automation and scripting. 1) In web development, Django and Flask frameworks simplify the development process. 2) In the fields of data science and machine learning, NumPy, Pandas, Scikit-learn and TensorFlow libraries provide strong support. 3) In terms of automation and scripting, Python is suitable for tasks such as automated testing and system management.
