Exploring Embedding Models with Vertex AI
Vector embeddings are fundamental to many advanced AI applications, including semantic search and anomaly detection. This article provides a foundational understanding of embeddings, focusing on sentence embeddings and vector representations. We'll explore practical techniques like mean pooling and cosine similarity, delve into the architecture of dual encoders using BERT, and examine their application in anomaly detection with Vertex AI for tasks such as fraud detection and content moderation.
Key Learning Objectives
- Grasp the role of vector embeddings in representing words, sentences, and other data types within a continuous vector space.
- Understand tokenization and how token embeddings contribute to sentence-level embeddings.
- Learn key concepts and best practices for deploying embedding models using Vertex AI to address real-world AI challenges.
- Discover how to optimize and scale applications with Vertex AI by integrating embedding models for enhanced analytics and decision-making.
- Gain hands-on experience training a dual encoder model, defining its architecture and training process.
- Implement anomaly detection using methods like Isolation Forest to identify outliers based on embedding similarity.
*This article is part of the***Data Science Blogathon.
Table of Contents
- Understanding Vertex Embeddings
- Sentence Embeddings Explained
- Cosine Similarity in Sentence Embeddings
- Training a Dual Encoder Model
- Dual Encoders for Question-Answering
- The Dual Encoder Training Process
- Leveraging Embeddings with Vertex AI
- Dataset Creation from Stack Overflow
- Generating Text Embeddings
- Batch Embedding Generation
- Anomaly Identification
- Isolation Forest for Outlier Detection
- Conclusion
- Frequently Asked Questions
Understanding Vertex Embeddings
Vector embeddings represent words or sentences within a defined space. The proximity of these vectors signifies similarity; closer vectors indicate greater semantic similarity. While initially used primarily in NLP, their application extends to images, videos, audio, and graphs. CLIP, a prominent multimodal learning model, generates both image and text embeddings.
Key applications of vector embeddings include:
- LLMs utilize them as token embeddings after input token conversion.
- Semantic search employs them to find the most relevant answers to queries.
- In Retrieval Augmented Generation (RAG), sentence embeddings facilitate the retrieval of pertinent information chunks.
- Recommendation systems use them to represent products and identify related items.
Let's examine the importance of sentence embeddings in RAG pipelines.
The retrieval engine in the above diagram identifies database information relevant to user queries. Transformer-based cross-encoders can compare queries to all information, classifying relevance. However, this is slow. Vector databases offer a faster alternative by storing embeddings and using similarity searches, though accuracy may be slightly lower.
Understanding Sentence Embeddings
Sentence embeddings are created by applying mathematical operations to token embeddings, often generated by pre-trained models like BERT or GPT. The following code demonstrates mean pooling of BERT-generated token embeddings to create sentence embeddings:
model_name = "./models/bert-base-uncased" tokenizer = BertTokenizer.from_pretrained(model_name) model = BertModel.from_pretrained(model_name) def get_sentence_embedding(sentence): encoded_input = tokenizer(sentence, padding=True, truncation=True, return_tensors='pt') attention_mask = encoded_input['attention_mask'] with torch.no_grad(): output = model(**encoded_input) token_embeddings = output.last_hidden_state input_mask_expanded = attention_mask.unsqueeze(-1).expand(token_embeddings.size()).float() sentence_embedding = torch.sum(token_embeddings * input_mask_expanded, 1) / torch.clamp(input_mask_expanded.sum(1), min=1e-9) return sentence_embedding.flatten().tolist()
This code loads a BERT model and defines a function to compute sentence embeddings using mean pooling.
Cosine Similarity of Sentence Embeddings
Cosine similarity measures the similarity between two vectors, making it suitable for comparing sentence embeddings. The following code implements cosine similarity and visualization:
def cosine_similarity_matrix(features): norms = np.linalg.norm(features, axis=1, keepdims=True) normalized_features = features / norms similarity_matrix = np.inner(normalized_features, normalized_features) rounded_similarity_matrix = np.round(similarity_matrix, 4) return rounded_similarity_matrix def plot_similarity(labels, features, rotation): sim = cosine_similarity_matrix(features) sns.set_theme(font_scale=1.2) g = sns.heatmap(sim, xticklabels=labels, yticklabels=labels, vmin=0, vmax=1, cmap="YlOrRd") g.set_xticklabels(labels, rotation=rotation) g.set_title("Semantic Textual Similarity") return g messages = [ # Technology "I prefer using a MacBook for work.", "Is AI taking over human jobs?", "My laptop battery drains too quickly.", # Sports "Did you watch the World Cup finals last night?", "LeBron James is an incredible basketball player.", "I enjoy running marathons on weekends.", # Travel "Paris is a beautiful city to visit.", "What are the best places to travel in summer?", "I love hiking in the Swiss Alps.", # Entertainment "The latest Marvel movie was fantastic!", "Do you listen to Taylor Swift's songs?", "I binge-watched an entire season of my favorite series.", ] embeddings = [] for t in messages: emb = get_sentence_embedding(t) embeddings.append(emb) plot_similarity(messages, embeddings, 90)
This code defines sentences, generates embeddings, and plots a heatmap showing their cosine similarity. The results might show unexpectedly high similarity, motivating the exploration of more accurate methods like dual encoders.
(The remaining sections continue in a similar manner, paraphrasing and restructuring the original text while maintaining the core information and preserving the image locations and formats.)
The above is the detailed content of Exploring Embedding Models with Vertex AI. For more information, please follow other related articles on the PHP Chinese website!

Hot AI Tools

Undresser.AI Undress
AI-powered app for creating realistic nude photos

AI Clothes Remover
Online AI tool for removing clothes from photos.

Undress AI Tool
Undress images for free

Clothoff.io
AI clothes remover

Video Face Swap
Swap faces in any video effortlessly with our completely free AI face swap tool!

Hot Article

Hot Tools

Notepad++7.3.1
Easy-to-use and free code editor

SublimeText3 Chinese version
Chinese version, very easy to use

Zend Studio 13.0.1
Powerful PHP integrated development environment

Dreamweaver CS6
Visual web development tools

SublimeText3 Mac version
God-level code editing software (SublimeText3)

Hot Topics
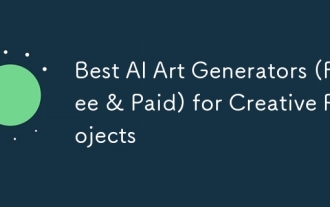
The article reviews top AI art generators, discussing their features, suitability for creative projects, and value. It highlights Midjourney as the best value for professionals and recommends DALL-E 2 for high-quality, customizable art.
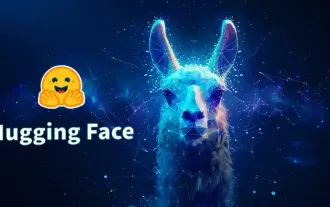
Meta's Llama 3.2: A Leap Forward in Multimodal and Mobile AI Meta recently unveiled Llama 3.2, a significant advancement in AI featuring powerful vision capabilities and lightweight text models optimized for mobile devices. Building on the success o
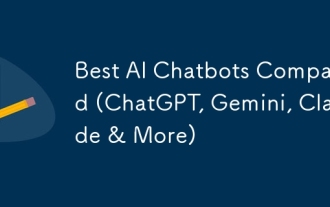
The article compares top AI chatbots like ChatGPT, Gemini, and Claude, focusing on their unique features, customization options, and performance in natural language processing and reliability.
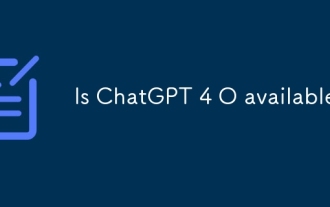
ChatGPT 4 is currently available and widely used, demonstrating significant improvements in understanding context and generating coherent responses compared to its predecessors like ChatGPT 3.5. Future developments may include more personalized interactions and real-time data processing capabilities, further enhancing its potential for various applications.
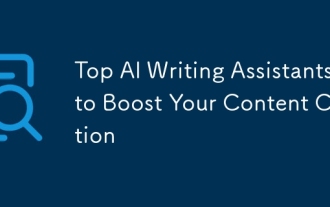
The article discusses top AI writing assistants like Grammarly, Jasper, Copy.ai, Writesonic, and Rytr, focusing on their unique features for content creation. It argues that Jasper excels in SEO optimization, while AI tools help maintain tone consist
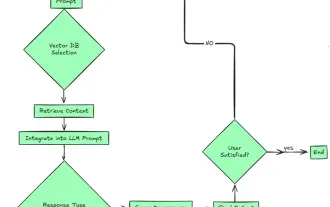
2024 witnessed a shift from simply using LLMs for content generation to understanding their inner workings. This exploration led to the discovery of AI Agents – autonomous systems handling tasks and decisions with minimal human intervention. Buildin
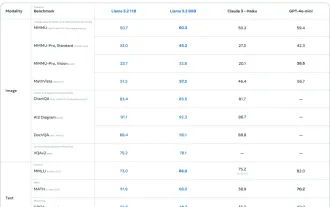
This week's AI landscape: A whirlwind of advancements, ethical considerations, and regulatory debates. Major players like OpenAI, Google, Meta, and Microsoft have unleashed a torrent of updates, from groundbreaking new models to crucial shifts in le
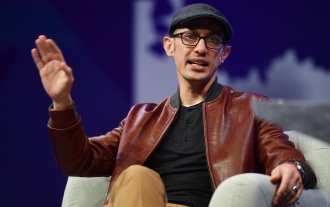
Shopify CEO Tobi Lütke's recent memo boldly declares AI proficiency a fundamental expectation for every employee, marking a significant cultural shift within the company. This isn't a fleeting trend; it's a new operational paradigm integrated into p
