


How do I optimize memory usage when working with large data structures in Go?
How to Optimize Memory Usage When Working with Large Data Structures in Go?
Optimizing memory usage when dealing with large data structures in Go requires a multifaceted approach. The key is to minimize allocations and reuse memory whenever possible. Here's a breakdown of effective strategies:
- Use Value Types When Possible: Prefer value types (structs, ints, floats, etc.) over reference types (interfaces, maps, slices) when the data is relatively small. Value types are copied directly, avoiding the overhead of pointer manipulation and garbage collection. However, be mindful of the cost of copying large value types; in those cases, consider using pointers.
-
Choose Appropriate Data Structures: Select data structures that are optimized for the specific task. For example, if you need fast lookups, a
map
might be ideal, but if you need ordered data and frequent insertions/deletions, alist
might be better. Consider the trade-offs between memory usage and performance characteristics. - Avoid Unnecessary Allocations: Allocate memory only when absolutely necessary. Reuse buffers and temporary variables whenever possible. Utilize techniques like object pooling to recycle objects instead of constantly allocating new ones.
-
Use
sync.Pool
for Object Reuse: Thesync.Pool
allows for reusing objects that are frequently created and destroyed. It's particularly beneficial for short-lived objects. However, be aware thatsync.Pool
is not a guaranteed performance boost and may even negatively impact performance in certain scenarios. It's crucial to profile your application to determine if it offers a real benefit. - Use Memory-Mapped Files: For extremely large datasets that don't fit comfortably in RAM, consider using memory-mapped files. This allows you to access data directly from disk, minimizing the amount of data loaded into memory at any given time.
-
Profiling and Benchmarking: Crucially, use Go's profiling tools (
pprof
) to identify memory bottlenecks in your code. This will give you concrete data on where memory is being consumed and guide your optimization efforts. Benchmarking helps you quantify the impact of your changes.
What Are the Best Practices for Minimizing Garbage Collection Pauses When Handling Large Datasets in Go?
Garbage collection (GC) pauses can be a significant performance issue when working with large datasets. Here are best practices to minimize their impact:
- Reduce Allocation Rate: The primary way to reduce GC pauses is to reduce the rate at which memory is allocated. By minimizing allocations, you lessen the workload on the garbage collector. The techniques mentioned in the previous section (using value types, reusing buffers, etc.) directly contribute to this goal.
- Use Larger Objects: Allocating fewer, larger objects is often more efficient than allocating many small objects. The garbage collector is more efficient when dealing with fewer objects.
- Tune GC Parameters (with caution): Go's garbage collector offers some tunable parameters. However, tweaking these parameters requires a deep understanding of the GC and the specific characteristics of your application. Incorrect tuning can often lead to worse performance. Profiling is essential before and after any changes to these parameters.
- Consider Goroutines and Concurrency: Break down large tasks into smaller, concurrent units of work using goroutines. This can improve throughput and reduce the impact of GC pauses by spreading the workload. However, be mindful of potential synchronization overhead.
- Use Escape Analysis: The Go compiler performs escape analysis to determine whether objects allocated on the stack can remain there or need to be moved to the heap. Optimizing your code to avoid heap allocations wherever possible improves performance and reduces GC pressure.
Are There Specific Go Data Structures Better Suited for Memory Efficiency Than Others When Dealing with Massive Amounts of Data?
Yes, some Go data structures are inherently more memory-efficient than others for massive datasets.
- Arrays and Slices (with caution): Arrays have fixed sizes and are allocated contiguously in memory. Slices are dynamic, but they hold a pointer to an underlying array, a length, and a capacity. While offering flexibility, slices can incur overhead due to the extra metadata. For extremely large datasets, consider carefully whether the dynamic nature of slices is truly necessary or if a fixed-size array would suffice.
- Maps: Maps offer fast lookups but can consume significant memory, especially if the keys are large or complex. Consider using smaller, more efficient key types if possible.
- Channels (for inter-goroutine communication): Channels are memory-efficient for inter-goroutine communication, especially when used with buffered channels. The buffer helps avoid blocking and reduces the need for frequent context switches.
- Custom Data Structures: For truly massive datasets, consider implementing custom data structures tailored to your specific needs and memory constraints. This might involve techniques like using memory pools or specialized tree structures that minimize memory overhead.
What Techniques Can I Use to Reduce Memory Allocation in Go Programs Processing Large Data Structures?
Reducing memory allocation is crucial for efficiency. Here are some techniques:
- Object Pooling: Reuse objects instead of repeatedly allocating and deallocating them. This is especially effective for frequently used objects.
- Pre-allocation: Allocate memory upfront for arrays or slices if you know the approximate size in advance. This avoids the overhead of repeatedly resizing the data structure as it grows.
- Memory Recycling: Design your code to recycle memory where possible. For instance, instead of creating a new object every time, reuse existing objects by clearing or resetting their contents.
- Slice Reslicing (with caution): While convenient, frequently reslicing a slice can lead to unnecessary allocations. If possible, try to work with the slice's underlying array directly or use a different approach that avoids repeated reslicing.
-
Use
unsafe
Package (with extreme caution): Theunsafe
package allows for low-level memory manipulation, but it should be used with extreme caution. Incorrect use can easily lead to memory corruption and program crashes. It's generally only recommended for highly specialized scenarios and experienced Go developers.
By employing these strategies, you can significantly improve the memory efficiency and performance of your Go programs when handling large data structures. Remember that profiling and benchmarking are crucial for identifying bottlenecks and verifying the effectiveness of your optimizations.
The above is the detailed content of How do I optimize memory usage when working with large data structures in Go?. For more information, please follow other related articles on the PHP Chinese website!

Hot AI Tools

Undresser.AI Undress
AI-powered app for creating realistic nude photos

AI Clothes Remover
Online AI tool for removing clothes from photos.

Undress AI Tool
Undress images for free

Clothoff.io
AI clothes remover

Video Face Swap
Swap faces in any video effortlessly with our completely free AI face swap tool!

Hot Article

Hot Tools

Notepad++7.3.1
Easy-to-use and free code editor

SublimeText3 Chinese version
Chinese version, very easy to use

Zend Studio 13.0.1
Powerful PHP integrated development environment

Dreamweaver CS6
Visual web development tools

SublimeText3 Mac version
God-level code editing software (SublimeText3)

Hot Topics
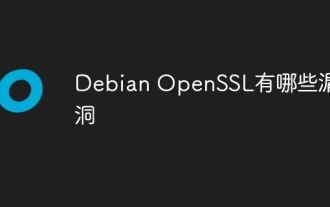
OpenSSL, as an open source library widely used in secure communications, provides encryption algorithms, keys and certificate management functions. However, there are some known security vulnerabilities in its historical version, some of which are extremely harmful. This article will focus on common vulnerabilities and response measures for OpenSSL in Debian systems. DebianOpenSSL known vulnerabilities: OpenSSL has experienced several serious vulnerabilities, such as: Heart Bleeding Vulnerability (CVE-2014-0160): This vulnerability affects OpenSSL 1.0.1 to 1.0.1f and 1.0.2 to 1.0.2 beta versions. An attacker can use this vulnerability to unauthorized read sensitive information on the server, including encryption keys, etc.
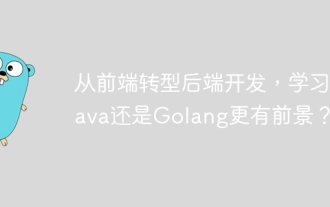
Backend learning path: The exploration journey from front-end to back-end As a back-end beginner who transforms from front-end development, you already have the foundation of nodejs,...
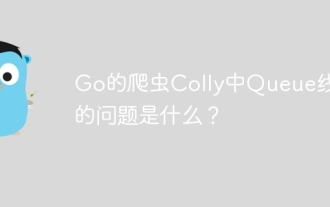
Queue threading problem in Go crawler Colly explores the problem of using the Colly crawler library in Go language, developers often encounter problems with threads and request queues. �...
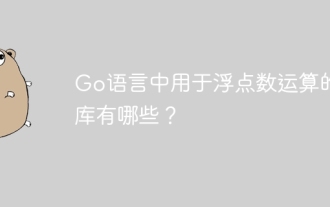
The library used for floating-point number operation in Go language introduces how to ensure the accuracy is...
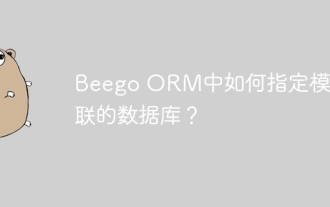
Under the BeegoORM framework, how to specify the database associated with the model? Many Beego projects require multiple databases to be operated simultaneously. When using Beego...
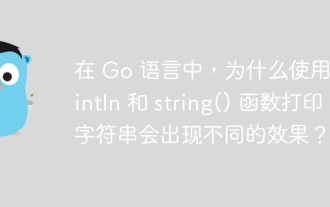
The difference between string printing in Go language: The difference in the effect of using Println and string() functions is in Go...
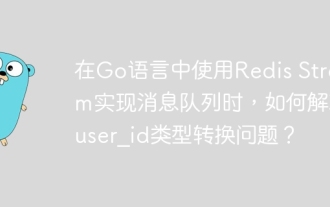
The problem of using RedisStream to implement message queues in Go language is using Go language and Redis...
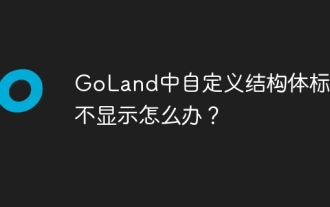
What should I do if the custom structure labels in GoLand are not displayed? When using GoLand for Go language development, many developers will encounter custom structure tags...
