Face Detection and Recognition with Keras
This tutorial demonstrates face detection and recognition using Python, leveraging the MTCNN and VGGFace2 models. We'll learn to identify faces in images, extract them, and compare them to determine if they belong to the same individual.
This process involves several key steps:
-
Face Detection with MTCNN: We utilize the pre-trained MTCNN model in Keras to locate and extract faces from images. This model efficiently identifies facial features and bounding boxes.
-
Face Recognition with VGGFace2: Once faces are extracted, the VGGFace2 model compares their features to assess similarity. This model, developed by Oxford's Visual Geometry Group, excels at recognizing individuals.
-
Image Preprocessing and Handling: The tutorial covers essential image handling using
matplotlib
for reading and manipulating images andPIL
for resizing. -
Model Comparison and Thresholds: We'll learn how to compare faces using cosine similarity, a metric well-suited for comparing feature vectors. The importance of setting appropriate thresholds for accurate face recognition will be highlighted.
Prerequisites:
Before beginning, install the necessary Python libraries:
pip3 install matplotlib keras mtcnn keras_vggface
Step-by-Step Guide:
The tutorial demonstrates the process using images of Lee Iacocca and Chelsea Football Club players. It covers:
-
Retrieving External Images: A
store_image
function downloads images from URLs and saves them locally. -
Face Detection: The
MTCNN
model is used to detect faces, providing bounding boxes and confidence scores. - Highlighting Faces: A visualization function draws rectangles around detected faces for verification.
- Face Extraction: Faces are extracted from images and resized to 224x224 pixels for compatibility with VGGFace2.
-
Face Comparison: The
VGGFace2
model generates feature vectors for each face, and cosine similarity is used to compare them. A threshold determines whether faces are considered a match.
Key Considerations:
- Threshold Selection: The choice of similarity threshold significantly impacts accuracy. Experimentation and careful consideration of factors like lighting and pose are crucial.
- Model Limitations: The accuracy of face recognition can be affected by variations in pose, expression, and image quality.
Conclusion:
This tutorial provides a practical introduction to face detection and recognition using readily available pre-trained models. While building and training your own model requires significant resources, this approach offers a quick and effective solution for many applications. Remember to adjust thresholds and consider model limitations for optimal results.
Frequently Asked Questions (FAQs):
The FAQs section addresses common questions about CNNs, VGGFace2, model accuracy improvement, Keras' role, comparisons with ML Kit and FaceNet, real-world applications, and challenges in face detection and recognition. These FAQs provide a comprehensive understanding of the underlying concepts and techniques.
The above is the detailed content of Face Detection and Recognition with Keras. For more information, please follow other related articles on the PHP Chinese website!

Hot AI Tools

Undresser.AI Undress
AI-powered app for creating realistic nude photos

AI Clothes Remover
Online AI tool for removing clothes from photos.

Undress AI Tool
Undress images for free

Clothoff.io
AI clothes remover

Video Face Swap
Swap faces in any video effortlessly with our completely free AI face swap tool!

Hot Article

Hot Tools

Notepad++7.3.1
Easy-to-use and free code editor

SublimeText3 Chinese version
Chinese version, very easy to use

Zend Studio 13.0.1
Powerful PHP integrated development environment

Dreamweaver CS6
Visual web development tools

SublimeText3 Mac version
God-level code editing software (SublimeText3)

Hot Topics










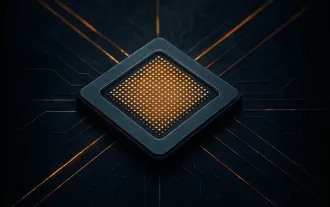
This pilot program, a collaboration between the CNCF (Cloud Native Computing Foundation), Ampere Computing, Equinix Metal, and Actuated, streamlines arm64 CI/CD for CNCF GitHub projects. The initiative addresses security concerns and performance lim
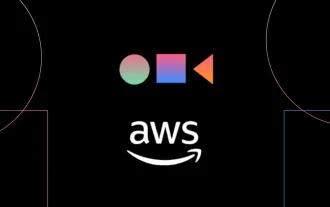
This tutorial guides you through building a serverless image processing pipeline using AWS services. We'll create a Next.js frontend deployed on an ECS Fargate cluster, interacting with an API Gateway, Lambda functions, S3 buckets, and DynamoDB. Th
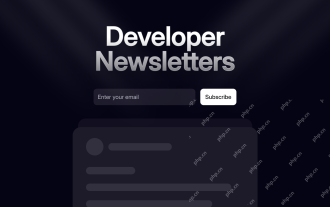
Stay informed about the latest tech trends with these top developer newsletters! This curated list offers something for everyone, from AI enthusiasts to seasoned backend and frontend developers. Choose your favorites and save time searching for rel
