


AI SDK by Vercel vs. Pydantic AI: A Tale of Two Frameworks for Modern AI Development
Introduction: A Framework Face-Off
The explosive growth of generative AI has fueled the development of specialized frameworks catering to diverse technological ecosystems. This comparison focuses on two prominent contenders: Vercel AI SDK (TypeScript/JavaScript) and Pydantic AI (Python), each offering unique approaches to integrating Large Language Models (LLMs) into applications. While both aim to streamline AI development, their design philosophies, strengths, and ideal use cases differ significantly.
Framework Overview
Vercel AI SDK
A TypeScript-centric toolkit designed for building high-performance, streaming AI user interfaces.
- Target Users: Web developers using React, Next.js, or Svelte.
-
Key Advantages:
- Effortless streaming UI integration with edge runtime for minimal latency.
- Broad LLM provider support (OpenAI, Google Vertex AI, Mistral, etc.).
- Built-in tool-calling capabilities with robust error handling.
- Efficient non-blocking data streaming for real-time interactions and Retrieval Augmented Generation (RAG).
-
Limitations:
- Close coupling with JavaScript frameworks limits backend flexibility.
- Relatively less emphasis on input/output validation compared to Pydantic AI.
Pydantic AI
A Python-based framework emphasizing type safety and production-ready AI agent development.
- Target Users: Python engineers prioritizing data integrity and agent orchestration.
-
Key Advantages:
- Type-safe workflows utilizing Pydantic models for enhanced reliability.
- Native dependency injection for creating modular and testable agents.
- Rigorous structured response validation for both static and streaming outputs.
- Seamless integration with established Python ecosystems (FastAPI, Logfire).
-
Limitations:
- Restricted to Python, excluding web-focused applications.
- Being in early beta (as of 2025) may mean API instability.
Shared Features
Both frameworks tackle core AI integration challenges:
- Multi-Model Compatibility: Both support multiple LLMs (OpenAI, Google Gemini, Anthropic, etc.).
- Structured Outputs: Vercel uses Zod-like schemas, while Pydantic AI leverages its namesake models for output validation.
- Tool Integration: Both enable LLMs to interact with external functions (e.g., database queries).
- Streaming Support: Vercel prioritizes real-time UI updates, whereas Pydantic AI validates streaming data chunks dynamically.
Distinguishing Features
Vercel AI SDK's Unique Strengths
-
Generative UI: Dynamically renders UI elements as LLM responses stream in, enhancing user experience. Example:
ui.done(<flightcard data="{...}"></flightcard>);
-
Edge Computing Architecture: Deploys on Vercel's edge network for ultra-low latency. Example:
export const runtime = 'edge';
- Tool Call Recovery: Automatically retries failed tool calls using more powerful models.
Pydantic AI's Standout Capabilities
-
Type-Safe Dependency Injection: Injects dependencies (e.g., database connections) with compile-time type checking. Example:
@dataclass class SupportDependencies: ...
-
Pythonic Workflow: Allows building agents using familiar Python syntax (async/await). Example:
@support_agent.tool async def customer_balance(...) -> float:
- Logfire Integration: Provides real-time monitoring and performance analysis.
LLM Provider Comparison
Provider | Vercel AI SDK | Pydantic AI |
---|---|---|
OpenAI | ✅ | ✅ |
Google Gemini | ✅ (via Vertex) | ✅ |
Anthropic | ✅ | ✅ (Beta) |
Groq | ✅ | ✅ |
Mistral | ✅ | ❌ |
Local Models | ❌ | ✅ |
Vercel excels with web-first providers, while Pydantic AI offers broader support for Python-native and local models.
Choosing the Right Framework
- Web Application Development (ChatGPT-style): Vercel AI SDK's React hooks and edge streaming are ideal.
- Enterprise-Grade Agent Development (e.g., fraud detection): Pydantic AI's type safety and auditability are paramount.
- Maximum LLM Flexibility: Both offer extensive support, but Pydantic AI simplifies local model integration.
Conclusion
Vercel AI SDK and Pydantic AI are not direct competitors but complementary tools. Vercel excels in the web UI space, while Pydantic AI dominates the Python backend. The best choice depends on your existing technology stack and project requirements. Consider using both for a truly comprehensive AI solution.
The above is the detailed content of AI SDK by Vercel vs. Pydantic AI: A Tale of Two Frameworks for Modern AI Development. For more information, please follow other related articles on the PHP Chinese website!

Hot AI Tools

Undresser.AI Undress
AI-powered app for creating realistic nude photos

AI Clothes Remover
Online AI tool for removing clothes from photos.

Undress AI Tool
Undress images for free

Clothoff.io
AI clothes remover

Video Face Swap
Swap faces in any video effortlessly with our completely free AI face swap tool!

Hot Article

Hot Tools

Notepad++7.3.1
Easy-to-use and free code editor

SublimeText3 Chinese version
Chinese version, very easy to use

Zend Studio 13.0.1
Powerful PHP integrated development environment

Dreamweaver CS6
Visual web development tools

SublimeText3 Mac version
God-level code editing software (SublimeText3)

Hot Topics










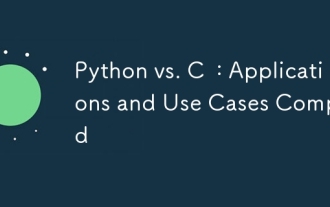
Python is suitable for data science, web development and automation tasks, while C is suitable for system programming, game development and embedded systems. Python is known for its simplicity and powerful ecosystem, while C is known for its high performance and underlying control capabilities.
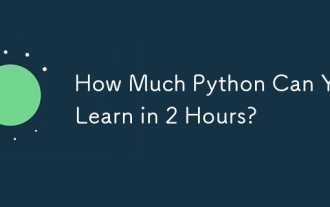
You can learn the basics of Python within two hours. 1. Learn variables and data types, 2. Master control structures such as if statements and loops, 3. Understand the definition and use of functions. These will help you start writing simple Python programs.
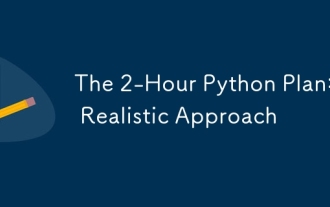
You can learn basic programming concepts and skills of Python within 2 hours. 1. Learn variables and data types, 2. Master control flow (conditional statements and loops), 3. Understand the definition and use of functions, 4. Quickly get started with Python programming through simple examples and code snippets.
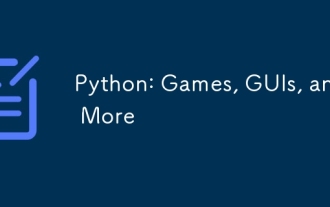
Python excels in gaming and GUI development. 1) Game development uses Pygame, providing drawing, audio and other functions, which are suitable for creating 2D games. 2) GUI development can choose Tkinter or PyQt. Tkinter is simple and easy to use, PyQt has rich functions and is suitable for professional development.
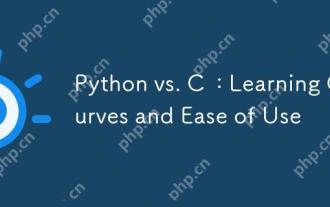
Python is easier to learn and use, while C is more powerful but complex. 1. Python syntax is concise and suitable for beginners. Dynamic typing and automatic memory management make it easy to use, but may cause runtime errors. 2.C provides low-level control and advanced features, suitable for high-performance applications, but has a high learning threshold and requires manual memory and type safety management.
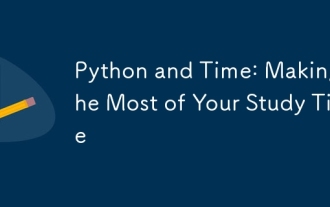
To maximize the efficiency of learning Python in a limited time, you can use Python's datetime, time, and schedule modules. 1. The datetime module is used to record and plan learning time. 2. The time module helps to set study and rest time. 3. The schedule module automatically arranges weekly learning tasks.
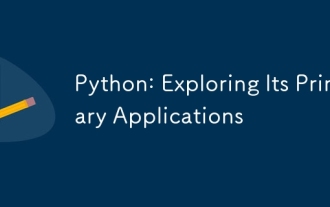
Python is widely used in the fields of web development, data science, machine learning, automation and scripting. 1) In web development, Django and Flask frameworks simplify the development process. 2) In the fields of data science and machine learning, NumPy, Pandas, Scikit-learn and TensorFlow libraries provide strong support. 3) In terms of automation and scripting, Python is suitable for tasks such as automated testing and system management.
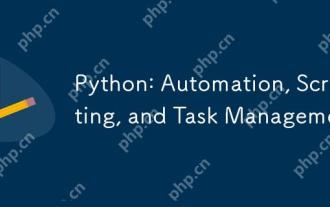
Python excels in automation, scripting, and task management. 1) Automation: File backup is realized through standard libraries such as os and shutil. 2) Script writing: Use the psutil library to monitor system resources. 3) Task management: Use the schedule library to schedule tasks. Python's ease of use and rich library support makes it the preferred tool in these areas.
