Distributed Programming: From Basics to Advanced Concepts
Introduction
Distributed programming is a cornerstone of modern software development, focusing on systems spanning multiple networked computers (nodes). These systems collaborate seamlessly to achieve complex tasks, sharing resources, data, and processing power through sophisticated message-passing.
Distributed systems underpin many everyday technologies, including cloud platforms, social media, cryptocurrencies, and global finance. Distributing computation and storage offers scalability, fault tolerance, and efficient resource use. However, it also presents challenges like network latency, partial failures, data consistency issues, and intricate coordination.
The strength of distributed programming lies in its ability to handle massive workloads exceeding the capacity of single machines. Horizontal scaling (adding more machines) provides virtually unlimited processing power. This, coupled with redundancy and fault tolerance, makes distributed systems ideal for mission-critical, high-availability applications.
This article explores key concepts, design patterns, and practical implementations in distributed computing. We'll cover communication protocols and consensus algorithms, providing real-world examples, from simple distributed caches to complex microservices. Mastering these principles is vital for contemporary software development.
Basic Concepts
Before tackling advanced topics, understanding fundamental distributed system concepts is crucial. These concepts form the basis for building reliable, scalable applications. We'll examine core inter-component communication mechanisms and interaction patterns.
Message Passing
Message passing is the foundation of distributed systems. Nodes communicate by exchanging messages. Here's a Python example using the socket
library:
import socket def create_server(): # ... (Server code as in original example) ... def create_client(): # ... (Client code as in original example) ... # Run the client create_client()
Remote Procedure Calls (RPC)
RPC enables programs to execute procedures on remote machines. Here's a Python example using XML-RPC:
from xmlrpc.server import SimpleXMLRPCServer from xmlrpc.client import ServerProxy # Server def start_rpc_server(): # ... (Server code as in original example) ... # Client def call_remote_factorial(): # ... (Client code as in original example) ... # Run the client (uncomment to execute) # call_remote_factorial()
Advanced Concepts
Building on the basics, let's delve into more advanced distributed programming concepts. These address complex challenges like maintaining system-wide consistency, managing distributed state, handling concurrency, and building resilient architectures. These are vital for enterprise-grade, scalable systems.
Distributed Consensus
Distributed consensus ensures multiple computers agree on a single value or action despite failures and network issues.
Key Aspects:
- Agreement: All healthy nodes agree on the same value.
- Integrity: Only proposed values are agreed upon.
- Termination: The algorithm eventually completes, with all healthy nodes deciding.
Challenges:
- Asynchronous Communication: Message delays or loss complicate determining node health.
- Node Failures: Node crashes disrupt the consensus process.
- Network Partitions: Network divisions isolate node groups, hindering communication.
Importance:
- Data Consistency: Ensures database replica consistency.
- Fault Tolerance: Systems operate even with node failures.
- Decentralization: Creates robust systems without single points of failure.
- Blockchain: Underpins blockchain's secure transactions.
Algorithms:
- Raft: Simple and understandable, widely used.
- Paxos: More complex but powerful.
- Zab: Used in Apache ZooKeeper.
(Simplified Raft Implementation - Conceptual)
- Leader Election: A leader node is elected.
- Log Replication: The leader replicates log entries (e.g., transactions) to followers.
- Consensus: Followers acknowledge and commit entries.
- State Machine Replication: Each node applies entries to its state machine, ensuring consistency.
(Raft Node Class - Conceptual)
import socket def create_server(): # ... (Server code as in original example) ... def create_client(): # ... (Client code as in original example) ... # Run the client create_client()
Distributed Cache, Distributed Task Queue, Distributed Lock, and Event-Driven Architecture
(The code examples for Distributed Cache using Redis, Distributed Task Queue using Celery, Distributed Lock using Redis, and Event-Driven Architecture using RabbitMQ remain largely the same as in the original input, with minor stylistic adjustments for consistency.)
Conclusion
Distributed programming presents significant challenges but offers powerful solutions for building scalable systems. The examples illustrate various patterns and techniques, from basic message passing to advanced consensus and event-driven architectures.
Remember that distributed systems increase complexity. Use them when the benefits (scalability, reliability, performance) outweigh the added complexity and operational overhead. Consider network failures, partial failures, and eventual consistency during design.
This article provides a foundational overview. Distributed programming is a vast field; continue learning and experimenting to find optimal solutions for your specific needs.
References
(The reference section remains the same as in the original input.)
The above is the detailed content of Distributed Programming: From Basics to Advanced Concepts. For more information, please follow other related articles on the PHP Chinese website!

Hot AI Tools

Undresser.AI Undress
AI-powered app for creating realistic nude photos

AI Clothes Remover
Online AI tool for removing clothes from photos.

Undress AI Tool
Undress images for free

Clothoff.io
AI clothes remover

Video Face Swap
Swap faces in any video effortlessly with our completely free AI face swap tool!

Hot Article

Hot Tools

Notepad++7.3.1
Easy-to-use and free code editor

SublimeText3 Chinese version
Chinese version, very easy to use

Zend Studio 13.0.1
Powerful PHP integrated development environment

Dreamweaver CS6
Visual web development tools

SublimeText3 Mac version
God-level code editing software (SublimeText3)

Hot Topics










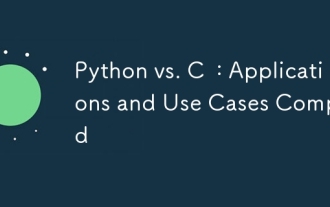
Python is suitable for data science, web development and automation tasks, while C is suitable for system programming, game development and embedded systems. Python is known for its simplicity and powerful ecosystem, while C is known for its high performance and underlying control capabilities.
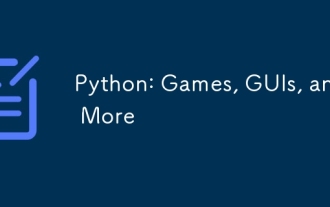
Python excels in gaming and GUI development. 1) Game development uses Pygame, providing drawing, audio and other functions, which are suitable for creating 2D games. 2) GUI development can choose Tkinter or PyQt. Tkinter is simple and easy to use, PyQt has rich functions and is suitable for professional development.
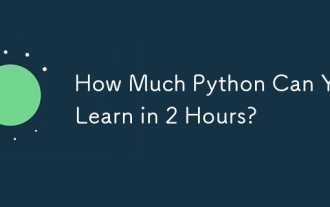
You can learn the basics of Python within two hours. 1. Learn variables and data types, 2. Master control structures such as if statements and loops, 3. Understand the definition and use of functions. These will help you start writing simple Python programs.
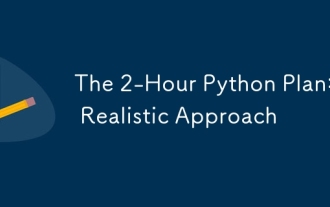
You can learn basic programming concepts and skills of Python within 2 hours. 1. Learn variables and data types, 2. Master control flow (conditional statements and loops), 3. Understand the definition and use of functions, 4. Quickly get started with Python programming through simple examples and code snippets.
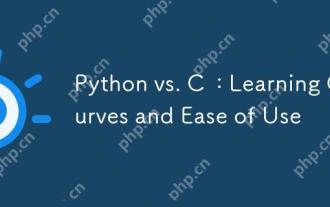
Python is easier to learn and use, while C is more powerful but complex. 1. Python syntax is concise and suitable for beginners. Dynamic typing and automatic memory management make it easy to use, but may cause runtime errors. 2.C provides low-level control and advanced features, suitable for high-performance applications, but has a high learning threshold and requires manual memory and type safety management.
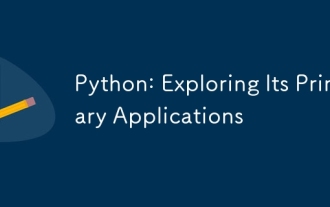
Python is widely used in the fields of web development, data science, machine learning, automation and scripting. 1) In web development, Django and Flask frameworks simplify the development process. 2) In the fields of data science and machine learning, NumPy, Pandas, Scikit-learn and TensorFlow libraries provide strong support. 3) In terms of automation and scripting, Python is suitable for tasks such as automated testing and system management.
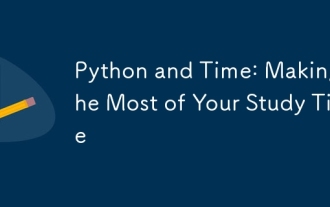
To maximize the efficiency of learning Python in a limited time, you can use Python's datetime, time, and schedule modules. 1. The datetime module is used to record and plan learning time. 2. The time module helps to set study and rest time. 3. The schedule module automatically arranges weekly learning tasks.
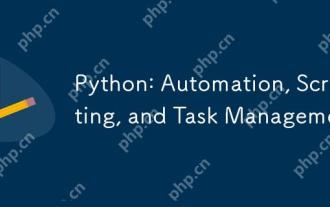
Python excels in automation, scripting, and task management. 1) Automation: File backup is realized through standard libraries such as os and shutil. 2) Script writing: Use the psutil library to monitor system resources. 3) Task management: Use the schedule library to schedule tasks. Python's ease of use and rich library support makes it the preferred tool in these areas.
