Analyzing APIs with PyInterceptor
PyInterceptor: A Python Function Call Interceptor for Non-Invasive Analysis
PyInterceptor is a Python library currently under development (available on GitHub) designed for non-invasive interception and analysis of function calls. Imagine needing detailed information—function names, parameters, return values, execution times—from a Python client interacting with a Python API, without modifying the existing code. PyInterceptor addresses this need.
This dev.to article introduces PyInterceptor's core concepts, use cases, and application.
Python Call Interception and Processing
Core Principles
Function call interception falls into two categories: blocking and non-blocking (Figure 1). Blocking interception captures call information and returns immediately, without executing the target function. This is beneficial for creating mocks or stubs during unit testing. Non-blocking interception gathers information, then executes the target function, capturing its return value before proceeding. PyInterceptor supports both modes.
Use Cases
Interception of Python API calls offers numerous applications:
- Automated Mock/Stub Creation: Utilize blocking mode to semi-automatically generate mocks and stubs for unit tests. PyInterceptor allows custom interceptor callables for returning simulated data.
- Structured Logging: Forward intercepted arguments and metadata to a logging system.
- Enhanced Debugging: Pinpoint bugs more efficiently by tracking function calls and their parameters.
- Call Statistics Generation: Collect performance metrics.
- And more…
Detailed Implementation
PyInterceptor operates by inserting a handler function that intercepts calls intended for the API. This handler captures metadata (arguments, timestamps, etc.), stores it in a CallInfo object, and manages call forwarding.
In blocking mode, the handler passes CallInfo to a user-defined interceptor callable. This callable processes the information (logging, statistics, etc.). The handler then returns the interceptor's result.
In non-blocking mode, the handler executes the target function, adds its return value to CallInfo, and then calls the interceptor. The actual target function's return value is returned to the caller, unlike in blocking mode.
Illustrative Code Example
This example demonstrates PyInterceptor's use with an arithmetic API and a Processor class, logging all method calls to a JSON file.
import json from pathlib import Path from typing import List from interceptor import intercept, get_methods, CallInfo class API: # ... (API methods remain unchanged) ... class Processor: # ... (Processor methods remain unchanged) ... class JSONLogger: # ... (JSONLogger class remains unchanged) ... if __name__ == '__main__': # ... (Main method remains unchanged) ...
The main method creates a JSONLogger instance (acting as the interceptor), intercepts the API and Processor methods using intercept()
, executes Processor methods, and saves the logs to "logs.json". The JSON output contains a detailed record of each function call.
Future Enhancements
Planned improvements for PyInterceptor include:
- Comprehensive API documentation and CI/CD pipeline.
- Python decorators for simplified class/method interception.
- Configuration options for managing memory usage (copying vs. referencing CallInfo data).
- Pre-built interceptor implementations for common use cases.
Your feedback is welcome! Please leave a comment if you found this article helpful or have suggestions for future development.
The above is the detailed content of Analyzing APIs with PyInterceptor. For more information, please follow other related articles on the PHP Chinese website!

Hot AI Tools

Undresser.AI Undress
AI-powered app for creating realistic nude photos

AI Clothes Remover
Online AI tool for removing clothes from photos.

Undress AI Tool
Undress images for free

Clothoff.io
AI clothes remover

Video Face Swap
Swap faces in any video effortlessly with our completely free AI face swap tool!

Hot Article

Hot Tools

Notepad++7.3.1
Easy-to-use and free code editor

SublimeText3 Chinese version
Chinese version, very easy to use

Zend Studio 13.0.1
Powerful PHP integrated development environment

Dreamweaver CS6
Visual web development tools

SublimeText3 Mac version
God-level code editing software (SublimeText3)

Hot Topics










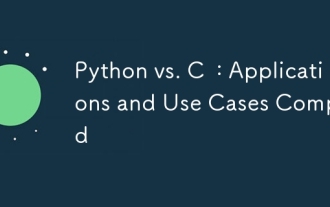
Python is suitable for data science, web development and automation tasks, while C is suitable for system programming, game development and embedded systems. Python is known for its simplicity and powerful ecosystem, while C is known for its high performance and underlying control capabilities.
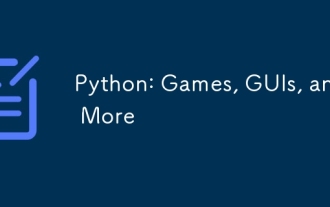
Python excels in gaming and GUI development. 1) Game development uses Pygame, providing drawing, audio and other functions, which are suitable for creating 2D games. 2) GUI development can choose Tkinter or PyQt. Tkinter is simple and easy to use, PyQt has rich functions and is suitable for professional development.
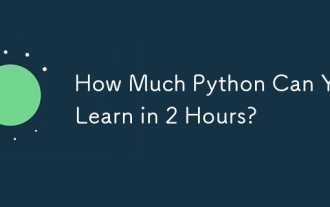
You can learn the basics of Python within two hours. 1. Learn variables and data types, 2. Master control structures such as if statements and loops, 3. Understand the definition and use of functions. These will help you start writing simple Python programs.
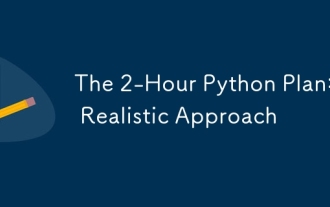
You can learn basic programming concepts and skills of Python within 2 hours. 1. Learn variables and data types, 2. Master control flow (conditional statements and loops), 3. Understand the definition and use of functions, 4. Quickly get started with Python programming through simple examples and code snippets.
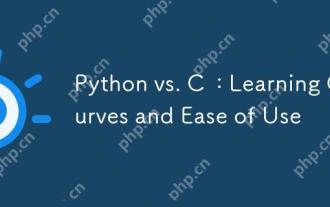
Python is easier to learn and use, while C is more powerful but complex. 1. Python syntax is concise and suitable for beginners. Dynamic typing and automatic memory management make it easy to use, but may cause runtime errors. 2.C provides low-level control and advanced features, suitable for high-performance applications, but has a high learning threshold and requires manual memory and type safety management.
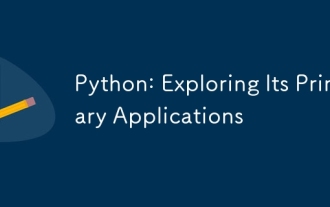
Python is widely used in the fields of web development, data science, machine learning, automation and scripting. 1) In web development, Django and Flask frameworks simplify the development process. 2) In the fields of data science and machine learning, NumPy, Pandas, Scikit-learn and TensorFlow libraries provide strong support. 3) In terms of automation and scripting, Python is suitable for tasks such as automated testing and system management.
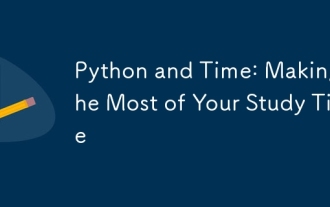
To maximize the efficiency of learning Python in a limited time, you can use Python's datetime, time, and schedule modules. 1. The datetime module is used to record and plan learning time. 2. The time module helps to set study and rest time. 3. The schedule module automatically arranges weekly learning tasks.
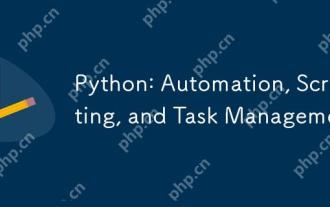
Python excels in automation, scripting, and task management. 1) Automation: File backup is realized through standard libraries such as os and shutil. 2) Script writing: Use the psutil library to monitor system resources. 3) Task management: Use the schedule library to schedule tasks. Python's ease of use and rich library support makes it the preferred tool in these areas.
