


Understanding RAG Workflow: Retrieval-Augmented Generation in Python
The Retrieval-Augmented Generation (RAG) workflow is an advanced approach in natural language processing (NLP) that combines retrieval and generation capabilities. It is particularly useful for tasks where the model needs to generate responses based on both its training data and external knowledge sources. This article will delve into how RAG works, its use cases, and how to implement it in Python.
What is Retrieval-Augmented Generation (RAG)?
RAG is a hybrid approach that marries two components:
- Retriever: Fetches relevant documents or information from an external knowledge base.
- Generator: Generates coherent and contextually relevant responses based on the retrieved documents.
By combining these components, RAG workflows enable models to generate more accurate, context-aware, and up-to-date outputs compared to standalone generative models.
How RAG Workflow Works
- Input Query: The user provides a query, such as a question or a prompt.
- Document Retrieval: The retriever searches for relevant documents in an external database, such as Elasticsearch, a vector database, or even a simple text corpus.
- Contextual Embedding: Retrieved documents are passed to the generator along with the original query to provide additional context.
- Response Generation: The generator uses the query and retrieved documents to produce a final response.
Key Use Cases of RAG
- Question Answering: Providing precise answers using both internal and external knowledge bases.
- Chatbots: Enhancing conversational AI systems with up-to-date or domain-specific knowledge.
- Customer Support: Resolving queries by fetching and generating answers from a large corpus of FAQs or manuals.
- Research Assistance: Summarizing and answering questions based on scientific papers or other research materials.
Implementing RAG in Python
Here’s a step-by-step implementation of a basic RAG workflow using Python:
- Install Necessary Libraries:
pip install transformers langchain faiss-cpu sentence-transformers
- Set Up the Retriever: Use a vector database like FAISS for efficient retrieval.
from sentence_transformers import SentenceTransformer import faiss # Initialize embedding model model = SentenceTransformer('all-MiniLM-L6-v2') # Example documents documents = [ "Python is a versatile programming language.", "Transformers are powerful models for NLP tasks.", "FAISS is used for vector similarity search." ] # Generate embeddings doc_embeddings = model.encode(documents) # Create FAISS index dimension = doc_embeddings.shape[1] index = faiss.IndexFlatL2(dimension) index.add(doc_embeddings)
- Define the Generator: Use a pre-trained language model from Hugging Face.
from transformers import pipeline # Initialize text generation pipeline generator = pipeline('text-generation', model='gpt2')
- Integrate Retriever and Generator: Combine the retriever and generator to form the RAG workflow.
def rag_pipeline(query): # Retrieve similar documents query_embedding = model.encode([query]) distances, indices = index.search(query_embedding, k=2) retrieved_docs = [documents[i] for i in indices[0]] # Generate response using retrieved documents context = "\n".join(retrieved_docs) prompt = f"Context: {context}\nQuery: {query}\nAnswer:" response = generator(prompt, max_length=50, num_return_sequences=1) return response[0]['generated_text'] # Example query query = "What is FAISS?" print(rag_pipeline(query))
- Test the Workflow: Run the script and provide various queries to test the system’s performance.
Benefits of RAG Workflow
- Improved Accuracy: Leverages external knowledge for generating contextually accurate responses.
- Flexibility: Adapts to various domains by changing the knowledge base.
- Scalability: Works efficiently with large datasets using scalable retrieval mechanisms like FAISS.
Conclusion
The Retrieval-Augmented Generation (RAG) workflow represents a significant advancement in NLP by integrating retrieval and generation. It is highly versatile and finds applications in domains ranging from customer support to research. By implementing RAG in Python, as demonstrated above, you can create powerful, context-aware AI systems tailored to your specific needs.
Feel free to experiment with different retrieval systems or fine-tune the generator to better suit your applications. The possibilities are vast with RAG workflows!
The above is the detailed content of Understanding RAG Workflow: Retrieval-Augmented Generation in Python. For more information, please follow other related articles on the PHP Chinese website!

Hot AI Tools

Undresser.AI Undress
AI-powered app for creating realistic nude photos

AI Clothes Remover
Online AI tool for removing clothes from photos.

Undress AI Tool
Undress images for free

Clothoff.io
AI clothes remover

Video Face Swap
Swap faces in any video effortlessly with our completely free AI face swap tool!

Hot Article

Hot Tools

Notepad++7.3.1
Easy-to-use and free code editor

SublimeText3 Chinese version
Chinese version, very easy to use

Zend Studio 13.0.1
Powerful PHP integrated development environment

Dreamweaver CS6
Visual web development tools

SublimeText3 Mac version
God-level code editing software (SublimeText3)

Hot Topics










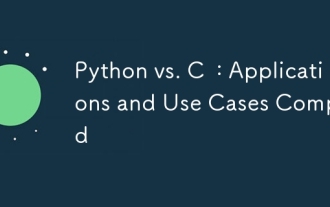
Python is suitable for data science, web development and automation tasks, while C is suitable for system programming, game development and embedded systems. Python is known for its simplicity and powerful ecosystem, while C is known for its high performance and underlying control capabilities.
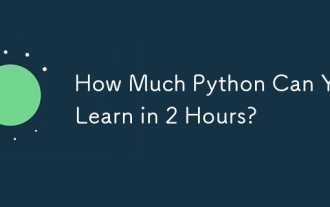
You can learn the basics of Python within two hours. 1. Learn variables and data types, 2. Master control structures such as if statements and loops, 3. Understand the definition and use of functions. These will help you start writing simple Python programs.
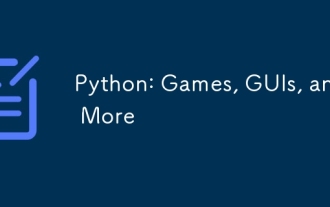
Python excels in gaming and GUI development. 1) Game development uses Pygame, providing drawing, audio and other functions, which are suitable for creating 2D games. 2) GUI development can choose Tkinter or PyQt. Tkinter is simple and easy to use, PyQt has rich functions and is suitable for professional development.
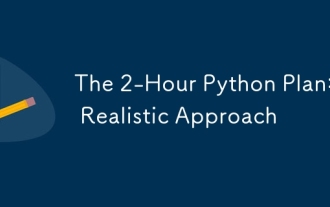
You can learn basic programming concepts and skills of Python within 2 hours. 1. Learn variables and data types, 2. Master control flow (conditional statements and loops), 3. Understand the definition and use of functions, 4. Quickly get started with Python programming through simple examples and code snippets.
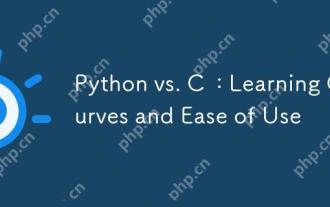
Python is easier to learn and use, while C is more powerful but complex. 1. Python syntax is concise and suitable for beginners. Dynamic typing and automatic memory management make it easy to use, but may cause runtime errors. 2.C provides low-level control and advanced features, suitable for high-performance applications, but has a high learning threshold and requires manual memory and type safety management.
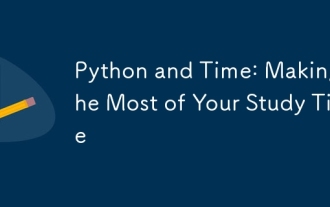
To maximize the efficiency of learning Python in a limited time, you can use Python's datetime, time, and schedule modules. 1. The datetime module is used to record and plan learning time. 2. The time module helps to set study and rest time. 3. The schedule module automatically arranges weekly learning tasks.
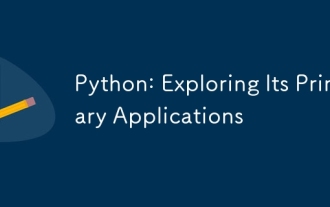
Python is widely used in the fields of web development, data science, machine learning, automation and scripting. 1) In web development, Django and Flask frameworks simplify the development process. 2) In the fields of data science and machine learning, NumPy, Pandas, Scikit-learn and TensorFlow libraries provide strong support. 3) In terms of automation and scripting, Python is suitable for tasks such as automated testing and system management.
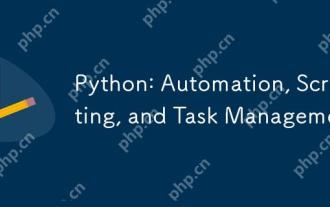
Python excels in automation, scripting, and task management. 1) Automation: File backup is realized through standard libraries such as os and shutil. 2) Script writing: Use the psutil library to monitor system resources. 3) Task management: Use the schedule library to schedule tasks. Python's ease of use and rich library support makes it the preferred tool in these areas.
