


Document Translation Service using Streamlit & AWS Translator
Introduction:
DocuTranslator, a document translation system, built in AWS and developed by Streamlit application framework. This application allows end user to translate the documents in their preferred language which they want to upload. It provides feasibility to translate in multiple languages as user wants, which really helps users to understand the content in their comfortable way.
Background:
The intent of this project is to provide a user friendly, simple application interface to fulfill the translation process as simple as users expect. In this system, nobody has to translate documents by entering into AWS Translate service, rather end user can directly access the application endpoint and get the requirements fulfilled.
High Level Architecture Diagram:
How Does This Work:
- End user is allowed to access an application through an application load balancer.
- Once application interface is opened, user will upload the required files to be translated and language to translate to.
- After submitting these details, file will be uploaded to mentioned source S3 bucket which triggers a lambda function to connect with AWS Translator service.
- Once translated document is ready, will be uploaded to destination S3 bucket.
- After that, end user can download the translated document from Streamlit application portal.
Technical Architecture:
Above architecture shows below key points -
- Application code has been containerized and stored to ECR repository.
- As per above design, an ECS cluster has been setup which instantiates two tasks that pulls application image from ECR repository.
- Both the tasks are launched on top of EC2 as a launch type. Both EC2s are launched in private subnet in us-east-1a and us-east-1b availability zones.
- A EFS file system is created to share application codes between two underlying EC2 instances. Two mountpoints are created in two availability zones (us-east-1a and us-east-1b).
- Two public subnets are configured in front of private subnets and a NAT gateway is set up in the public subnet in us-east-1a availability zone.
- An application load balancer has been configured in front of private subnets which distributes the traffic across two public subnets at port 80 of application load balancer security group(ALB SG).
- Two EC2 instances are configured in two different target group with same EC2 security group(Streamlit_SG) which accepts traffic at 16347 port from application load balancer.
- There is port mapping configured between port 16347 in EC2 instances and port 8501 at ECS container. Once traffic will hit at port 16347 of EC2 security group, will be redirected to 8501 port at ECS container level.
How Data is Getting Stored ?
Here, we have used EFS share path to share same application files between two underlying EC2 instances. We have created a mountpoint /streamlit_appfiles inside the EC2 instances and mounted with EFS share. This approach will help in sharing same content across two different servers. After that, our intent is to create a replicate same application content to container working directory which is /streamlit. For that we have used bind mounts so that whatever changes will be made on application code at EC2 level, will be replicated to container as well. We need to restrict bi-directional replication which says if anyone mistakenly changes code from inside the container, it should not replicate to EC2 host level, hence inside the container working directory has been created as a read only filesystem.
ECS Container Configuration and Volume:
Underlying EC2 Configuration:
Instance Type: t2.medium
Network type: Private Subnet
Container Configuration:
Image:
Network Mode: Default
Host Port: 16347
Container Port: 8501
Task CPU: 2 vCPU (2048 units)
Task Memory: 2.5 GB (2560 MiB)
Volume Configuration:
Volume Name: streamlit-volume
Source Path: /streamlit_appfiles
Container Path: /streamlit
Read Only Filesystem: YES
Task Definition Reference:
{ "taskDefinitionArn": "arn:aws:ecs:us-east-1:<account-id>:task-definition/Streamlit_TDF-1:5", "containerDefinitions": [ { "name": "streamlit", "image": "<account-id>.dkr.ecr.us-east-1.amazonaws.com/anirban:latest", "cpu": 0, "portMappings": [ { "name": "streamlit-8501-tcp", "containerPort": 8501, "hostPort": 16347, "protocol": "tcp", "appProtocol": "http" } ], "essential": true, "environment": [], "environmentFiles": [], "mountPoints": [ { "sourceVolume": "streamlit-volume", "containerPath": "/streamlit", "readOnly": true } ], "volumesFrom": [], "ulimits": [], "logConfiguration": { "logDriver": "awslogs", "options": { "awslogs-group": "/ecs/Streamlit_TDF-1", "mode": "non-blocking", "awslogs-create-group": "true", "max-buffer-size": "25m", "awslogs-region": "us-east-1", "awslogs-stream-prefix": "ecs" }, "secretOptions": [] }, "systemControls": [] } ], "family": "Streamlit_TDF-1", "taskRoleArn": "arn:aws:iam::<account-id>:role/ecsTaskExecutionRole", "executionRoleArn": "arn:aws:iam::<account-id>:role/ecsTaskExecutionRole", "revision": 5, "volumes": [ { "name": "streamlit-volume", "host": { "sourcePath": "/streamlit_appfiles" } } ], "status": "ACTIVE", "requiresAttributes": [ { "name": "com.amazonaws.ecs.capability.logging-driver.awslogs" }, { "name": "ecs.capability.execution-role-awslogs" }, { "name": "com.amazonaws.ecs.capability.ecr-auth" }, { "name": "com.amazonaws.ecs.capability.docker-remote-api.1.19" }, { "name": "com.amazonaws.ecs.capability.docker-remote-api.1.28" }, { "name": "com.amazonaws.ecs.capability.task-iam-role" }, { "name": "ecs.capability.execution-role-ecr-pull" }, { "name": "com.amazonaws.ecs.capability.docker-remote-api.1.18" }, { "name": "com.amazonaws.ecs.capability.docker-remote-api.1.29" } ], "placementConstraints": [], "compatibilities": [ "EC2" ], "requiresCompatibilities": [ "EC2" ], "cpu": "2048", "memory": "2560", "runtimePlatform": { "cpuArchitecture": "X86_64", "operatingSystemFamily": "LINUX" }, "registeredAt": "2024-11-09T05:59:47.534Z", "registeredBy": "arn:aws:iam::<account-id>:root", "tags": [] }
Developing Application Code and Creating Docker Image:
app.py
import streamlit as st import boto3 import os import time from pathlib import Path s3 = boto3.client('s3', region_name='us-east-1') tran = boto3.client('translate', region_name='us-east-1') lam = boto3.client('lambda', region_name='us-east-1') # Function to list S3 buckets def listbuckets(): list_bucket = s3.list_buckets() bucket_name = tuple([it["Name"] for it in list_bucket["Buckets"]]) return bucket_name # Upload object to S3 bucket def upload_to_s3bucket(file_path, selected_bucket, file_name): s3.upload_file(file_path, selected_bucket, file_name) def list_language(): response = tran.list_languages() list_of_langs = [i["LanguageName"] for i in response["Languages"]] return list_of_langs def wait_for_s3obj(dest_selected_bucket, file_name): while True: try: get_obj = s3.get_object(Bucket=dest_selected_bucket, Key=f'Translated-{file_name}.txt') obj_exist = 'true' if get_obj['Body'] else 'false' return obj_exist except s3.exceptions.ClientError as e: if e.response['Error']['Code'] == "404": print(f"File '{file_name}' not found. Checking again in 3 seconds...") time.sleep(3) def download(dest_selected_bucket, file_name, file_path): s3.download_file(dest_selected_bucket,f'Translated-{file_name}.txt', f'{file_path}/download/Translated-{file_name}.txt') with open(f"{file_path}/download/Translated-{file_name}.txt", "r") as file: st.download_button( label="Download", data=file, file_name=f"{file_name}.txt" ) def streamlit_application(): # Give a header st.header("Document Translator", divider=True) # Widgets to upload a file uploaded_files = st.file_uploader("Choose a PDF file", accept_multiple_files=True, type="pdf") # # upload a file file_name = uploaded_files[0].name.replace(' ', '_') if uploaded_files else None # Folder path file_path = '/tmp' # Select the bucket from drop down selected_bucket = st.selectbox("Choose the S3 Bucket to upload file :", listbuckets()) dest_selected_bucket = st.selectbox("Choose the S3 Bucket to download file :", listbuckets()) selected_language = st.selectbox("Choose the Language :", list_language()) # Create a button click = st.button("Upload", type="primary") if click == True: if file_name: with open(f'{file_path}/{file_name}', mode='wb') as w: w.write(uploaded_files[0].getvalue()) # Set the selected language to the environment variable of lambda function lambda_env1 = lam.update_function_configuration(FunctionName='TriggerFunctionFromS3', Environment={'Variables': {'UserInputLanguage': selected_language, 'DestinationBucket': dest_selected_bucket, 'TranslatedFileName': file_name}}) # Upload the file to S3 bucket: upload_to_s3bucket(f'{file_path}/{file_name}', selected_bucket, file_name) if s3.get_object(Bucket=selected_bucket, Key=file_name): st.success("File uploaded successfully", icon="✅") output = wait_for_s3obj(dest_selected_bucket, file_name) if output: download(dest_selected_bucket, file_name, file_path) else: st.error("File upload failed", icon="?") streamlit_application()
about.py
import streamlit as st ## Write the description of application st.header("About") about = ''' Welcome to the File Uploader Application! This application is designed to make uploading PDF documents simple and efficient. With just a few clicks, users can upload their documents securely to an Amazon S3 bucket for storage. Here’s a quick overview of what this app does: **Key Features:** - **Easy Upload:** Users can quickly upload PDF documents by selecting the file and clicking the 'Upload' button. - **Seamless Integration with AWS S3:** Once the document is uploaded, it is stored securely in a designated S3 bucket, ensuring reliable and scalable cloud storage. - **User-Friendly Interface:** Built using Streamlit, the interface is clean, intuitive, and accessible to all users, making the uploading process straightforward. **How it Works:** 1. **Select a PDF Document:** Users can browse and select any PDF document from their local system. 2. **Upload the Document:** Clicking the ‘Upload’ button triggers the process of securely uploading the selected document to an AWS S3 bucket. 3. **Success Notification:** After a successful upload, users will receive a confirmation message that their document has been stored in the cloud. This application offers a streamlined way to store documents on the cloud, reducing the hassle of manual file management. Whether you're an individual or a business, this tool helps you organize and store your files with ease and security. You can further customize this page by adding technical details, usage guidelines, or security measures as per your application's specifications.''' st.markdown(about)
navigation.py
import streamlit as st pg = st.navigation([ st.Page("app.py", title="DocuTranslator", icon="?"), st.Page("about.py", title="About", icon="?") ], position="sidebar") pg.run()
Dockerfile:
FROM python:3.9-slim WORKDIR /streamlit COPY requirements.txt /streamlit/requirements.txt RUN pip install --no-cache-dir -r requirements.txt RUN mkdir /tmp/download COPY . /streamlit EXPOSE 8501 CMD ["streamlit", "run", "navigation.py", "--server.port=8501", "--server.headless=true"]
Docker file will create an image by packaging all above application configuration files and then it was pushed to ECR repository. Docker Hub can also be used to store the image.
Load Balancing
In the architecture, application instances are supposed to be created in private subnet and load balancer is supposed to create to reduce incoming traffic load to private EC2 instances.
As there are two underlying EC2 hosts available to host containers, so load balancing is configured across two EC2 hosts to distribute incoming traffic. Two different target groups are created to place two EC2 instances in each with 50% weightage.
Load balancer accepts incoming traffic at port 80 and then passes to backend EC2 instances at port 16347 and that also passed to corresponding ECS container.
Lambda Function:
There is a lambda function configured to take source bucket as an input to download pdf file from there and extract the contents, then it translates the contents from current language to user provided target language and creates a text file to upload to destination S3 bucket.
{ "taskDefinitionArn": "arn:aws:ecs:us-east-1:<account-id>:task-definition/Streamlit_TDF-1:5", "containerDefinitions": [ { "name": "streamlit", "image": "<account-id>.dkr.ecr.us-east-1.amazonaws.com/anirban:latest", "cpu": 0, "portMappings": [ { "name": "streamlit-8501-tcp", "containerPort": 8501, "hostPort": 16347, "protocol": "tcp", "appProtocol": "http" } ], "essential": true, "environment": [], "environmentFiles": [], "mountPoints": [ { "sourceVolume": "streamlit-volume", "containerPath": "/streamlit", "readOnly": true } ], "volumesFrom": [], "ulimits": [], "logConfiguration": { "logDriver": "awslogs", "options": { "awslogs-group": "/ecs/Streamlit_TDF-1", "mode": "non-blocking", "awslogs-create-group": "true", "max-buffer-size": "25m", "awslogs-region": "us-east-1", "awslogs-stream-prefix": "ecs" }, "secretOptions": [] }, "systemControls": [] } ], "family": "Streamlit_TDF-1", "taskRoleArn": "arn:aws:iam::<account-id>:role/ecsTaskExecutionRole", "executionRoleArn": "arn:aws:iam::<account-id>:role/ecsTaskExecutionRole", "revision": 5, "volumes": [ { "name": "streamlit-volume", "host": { "sourcePath": "/streamlit_appfiles" } } ], "status": "ACTIVE", "requiresAttributes": [ { "name": "com.amazonaws.ecs.capability.logging-driver.awslogs" }, { "name": "ecs.capability.execution-role-awslogs" }, { "name": "com.amazonaws.ecs.capability.ecr-auth" }, { "name": "com.amazonaws.ecs.capability.docker-remote-api.1.19" }, { "name": "com.amazonaws.ecs.capability.docker-remote-api.1.28" }, { "name": "com.amazonaws.ecs.capability.task-iam-role" }, { "name": "ecs.capability.execution-role-ecr-pull" }, { "name": "com.amazonaws.ecs.capability.docker-remote-api.1.18" }, { "name": "com.amazonaws.ecs.capability.docker-remote-api.1.29" } ], "placementConstraints": [], "compatibilities": [ "EC2" ], "requiresCompatibilities": [ "EC2" ], "cpu": "2048", "memory": "2560", "runtimePlatform": { "cpuArchitecture": "X86_64", "operatingSystemFamily": "LINUX" }, "registeredAt": "2024-11-09T05:59:47.534Z", "registeredBy": "arn:aws:iam::<account-id>:root", "tags": [] }
Application Testing:
Open the application load balancer url "ALB-747339710.us-east-1.elb.amazonaws.com" to open the web application. Browse any pdf file, keep both source "fileuploadbucket-hwirio984092jjs" and destination bucket "translatedfileuploadbucket-kh939809kjkfjsekfl" as it is, because in the lambda code, it has been hard coded the target bucket is as mentioned above. Choose the language you want the document to be translated and click on upload. Once it's clicked, application program will start polling the destination S3 bucket to find out if the translated file is uploaded. If it find the exact file, then a new option "Download" will be visible to down load the file from destination S3 bucket.
Application Link: http://alb-747339710.us-east-1.elb.amazonaws.com/
Actual Content:
import streamlit as st import boto3 import os import time from pathlib import Path s3 = boto3.client('s3', region_name='us-east-1') tran = boto3.client('translate', region_name='us-east-1') lam = boto3.client('lambda', region_name='us-east-1') # Function to list S3 buckets def listbuckets(): list_bucket = s3.list_buckets() bucket_name = tuple([it["Name"] for it in list_bucket["Buckets"]]) return bucket_name # Upload object to S3 bucket def upload_to_s3bucket(file_path, selected_bucket, file_name): s3.upload_file(file_path, selected_bucket, file_name) def list_language(): response = tran.list_languages() list_of_langs = [i["LanguageName"] for i in response["Languages"]] return list_of_langs def wait_for_s3obj(dest_selected_bucket, file_name): while True: try: get_obj = s3.get_object(Bucket=dest_selected_bucket, Key=f'Translated-{file_name}.txt') obj_exist = 'true' if get_obj['Body'] else 'false' return obj_exist except s3.exceptions.ClientError as e: if e.response['Error']['Code'] == "404": print(f"File '{file_name}' not found. Checking again in 3 seconds...") time.sleep(3) def download(dest_selected_bucket, file_name, file_path): s3.download_file(dest_selected_bucket,f'Translated-{file_name}.txt', f'{file_path}/download/Translated-{file_name}.txt') with open(f"{file_path}/download/Translated-{file_name}.txt", "r") as file: st.download_button( label="Download", data=file, file_name=f"{file_name}.txt" ) def streamlit_application(): # Give a header st.header("Document Translator", divider=True) # Widgets to upload a file uploaded_files = st.file_uploader("Choose a PDF file", accept_multiple_files=True, type="pdf") # # upload a file file_name = uploaded_files[0].name.replace(' ', '_') if uploaded_files else None # Folder path file_path = '/tmp' # Select the bucket from drop down selected_bucket = st.selectbox("Choose the S3 Bucket to upload file :", listbuckets()) dest_selected_bucket = st.selectbox("Choose the S3 Bucket to download file :", listbuckets()) selected_language = st.selectbox("Choose the Language :", list_language()) # Create a button click = st.button("Upload", type="primary") if click == True: if file_name: with open(f'{file_path}/{file_name}', mode='wb') as w: w.write(uploaded_files[0].getvalue()) # Set the selected language to the environment variable of lambda function lambda_env1 = lam.update_function_configuration(FunctionName='TriggerFunctionFromS3', Environment={'Variables': {'UserInputLanguage': selected_language, 'DestinationBucket': dest_selected_bucket, 'TranslatedFileName': file_name}}) # Upload the file to S3 bucket: upload_to_s3bucket(f'{file_path}/{file_name}', selected_bucket, file_name) if s3.get_object(Bucket=selected_bucket, Key=file_name): st.success("File uploaded successfully", icon="✅") output = wait_for_s3obj(dest_selected_bucket, file_name) if output: download(dest_selected_bucket, file_name, file_path) else: st.error("File upload failed", icon="?") streamlit_application()
Translated Content (in Canadian French)
import streamlit as st ## Write the description of application st.header("About") about = ''' Welcome to the File Uploader Application! This application is designed to make uploading PDF documents simple and efficient. With just a few clicks, users can upload their documents securely to an Amazon S3 bucket for storage. Here’s a quick overview of what this app does: **Key Features:** - **Easy Upload:** Users can quickly upload PDF documents by selecting the file and clicking the 'Upload' button. - **Seamless Integration with AWS S3:** Once the document is uploaded, it is stored securely in a designated S3 bucket, ensuring reliable and scalable cloud storage. - **User-Friendly Interface:** Built using Streamlit, the interface is clean, intuitive, and accessible to all users, making the uploading process straightforward. **How it Works:** 1. **Select a PDF Document:** Users can browse and select any PDF document from their local system. 2. **Upload the Document:** Clicking the ‘Upload’ button triggers the process of securely uploading the selected document to an AWS S3 bucket. 3. **Success Notification:** After a successful upload, users will receive a confirmation message that their document has been stored in the cloud. This application offers a streamlined way to store documents on the cloud, reducing the hassle of manual file management. Whether you're an individual or a business, this tool helps you organize and store your files with ease and security. You can further customize this page by adding technical details, usage guidelines, or security measures as per your application's specifications.''' st.markdown(about)
Conclusion:
This article has shown us how document translation process can be as easy as we imagine where an end user has to click on some options to choose required information and get the desired output within few seconds without thinking about configuration. For now, we have included single feature to translate a pdf document, but later on, we will research more on this to have multi functionality in a single application with having some interesting features.
The above is the detailed content of Document Translation Service using Streamlit & AWS Translator. For more information, please follow other related articles on the PHP Chinese website!

Hot AI Tools

Undresser.AI Undress
AI-powered app for creating realistic nude photos

AI Clothes Remover
Online AI tool for removing clothes from photos.

Undress AI Tool
Undress images for free

Clothoff.io
AI clothes remover

Video Face Swap
Swap faces in any video effortlessly with our completely free AI face swap tool!

Hot Article

Hot Tools

Notepad++7.3.1
Easy-to-use and free code editor

SublimeText3 Chinese version
Chinese version, very easy to use

Zend Studio 13.0.1
Powerful PHP integrated development environment

Dreamweaver CS6
Visual web development tools

SublimeText3 Mac version
God-level code editing software (SublimeText3)

Hot Topics










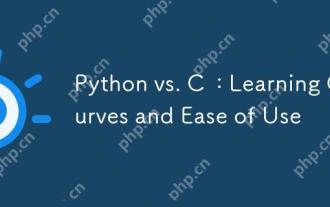
Python is easier to learn and use, while C is more powerful but complex. 1. Python syntax is concise and suitable for beginners. Dynamic typing and automatic memory management make it easy to use, but may cause runtime errors. 2.C provides low-level control and advanced features, suitable for high-performance applications, but has a high learning threshold and requires manual memory and type safety management.
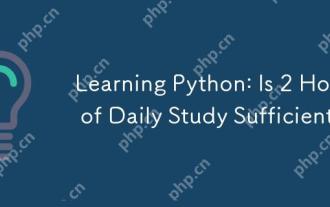
Is it enough to learn Python for two hours a day? It depends on your goals and learning methods. 1) Develop a clear learning plan, 2) Select appropriate learning resources and methods, 3) Practice and review and consolidate hands-on practice and review and consolidate, and you can gradually master the basic knowledge and advanced functions of Python during this period.
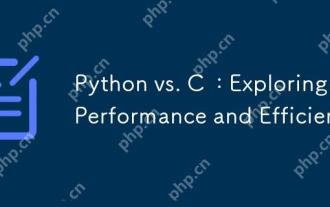
Python is better than C in development efficiency, but C is higher in execution performance. 1. Python's concise syntax and rich libraries improve development efficiency. 2.C's compilation-type characteristics and hardware control improve execution performance. When making a choice, you need to weigh the development speed and execution efficiency based on project needs.
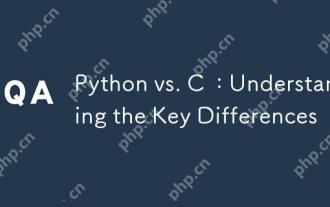
Python and C each have their own advantages, and the choice should be based on project requirements. 1) Python is suitable for rapid development and data processing due to its concise syntax and dynamic typing. 2)C is suitable for high performance and system programming due to its static typing and manual memory management.
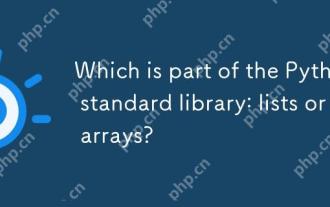
Pythonlistsarepartofthestandardlibrary,whilearraysarenot.Listsarebuilt-in,versatile,andusedforstoringcollections,whereasarraysareprovidedbythearraymoduleandlesscommonlyusedduetolimitedfunctionality.
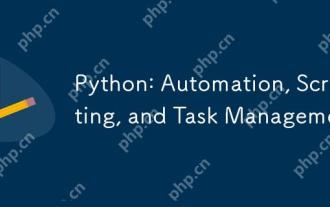
Python excels in automation, scripting, and task management. 1) Automation: File backup is realized through standard libraries such as os and shutil. 2) Script writing: Use the psutil library to monitor system resources. 3) Task management: Use the schedule library to schedule tasks. Python's ease of use and rich library support makes it the preferred tool in these areas.
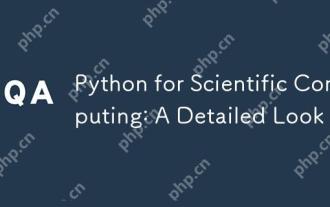
Python's applications in scientific computing include data analysis, machine learning, numerical simulation and visualization. 1.Numpy provides efficient multi-dimensional arrays and mathematical functions. 2. SciPy extends Numpy functionality and provides optimization and linear algebra tools. 3. Pandas is used for data processing and analysis. 4.Matplotlib is used to generate various graphs and visual results.
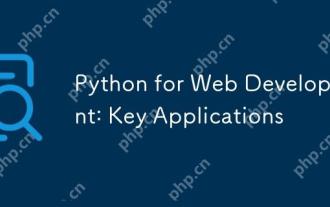
Key applications of Python in web development include the use of Django and Flask frameworks, API development, data analysis and visualization, machine learning and AI, and performance optimization. 1. Django and Flask framework: Django is suitable for rapid development of complex applications, and Flask is suitable for small or highly customized projects. 2. API development: Use Flask or DjangoRESTFramework to build RESTfulAPI. 3. Data analysis and visualization: Use Python to process data and display it through the web interface. 4. Machine Learning and AI: Python is used to build intelligent web applications. 5. Performance optimization: optimized through asynchronous programming, caching and code
