


Diving Deep into Data Engineering with Python: A Comprehensive Guide
In todays data driven world where lot of decisions are made off of data having a good understanding of data engineering concepts is critical. It generally involves logging data from online applications(web/mobile) into a data storage system, then setting up ETL(extract, transform, load) and finally building visualizations for in-depth data analysis to understand how the business or the app is doing. Python, with its versatility and extensive libraries, has become the go-to language for many data engineers.
This article will cover the key aspects of data engineering with Python, going over essential concepts, libraries, and some real-world applications.
1. Core Concepts in Data Engineering
-
Data Ingestion:
- The process of collecting data from various sources, such as databases, APIs, social media, and IoT devices.
-
Techniques:
- Batch processing (for large, static datasets)
- Stream processing (for real-time data)
- Change data capture (for incremental updates)
- Tools: Apache Kafka, Apache Flume, Debezium.
-
Data Transformation:
- The process of cleaning, transforming, and enriching raw data to make it suitable for analysis.
-
Techniques:
- Data cleaning (handling missing values, outliers, inconsistencies)
- Data integration (combining data from multiple sources)
- Data enrichment (adding external data)
- Tools: Apache Spark, Pandas, PySpark, Dataflow.
-
Data Storage:
- The process of storing transformed data in a suitable format for efficient retrieval and analysis.
-
Techniques:
- Data warehousing (for analytical workloads)
- Data lakes (for storing raw and processed data)
- NoSQL databases (for flexible data models)
- Tools: Amazon S3, Google Cloud Storage, Apache Hive, Cassandra.
-
Data Quality:
- Ensuring the accuracy, completeness, consistency, and timeliness of data.
- Techniques: Data profiling, data validation, data monitoring.
- Tools: Great Expectations, Apache Airflow.
-
Data Pipelines:
- Automated workflows that orchestrate the movement and transformation of data from source to destination.
- Techniques: ETL (Extract, Transform, Load), ELT (Extract, Load, Transform).
- Tools: Apache Airflow, Prefect, Luigi.
2. Essential Python Libraries for Data Engineering
-
Pandas:
- A powerful library for data manipulation and analysis. Offers data structures like Series and DataFrame, enabling efficient data cleaning, transformation, and aggregation.
- Example:
import pandas as pd # Load data from CSV df = pd.read_csv('data.csv') # Clean data df = df.dropna() df = df[df['age'] >= 18] # Transform data df['new_column'] = df['column1'] + df['column2'] # Save data to CSV df.to_csv('processed_data.csv', index=False)
Copy after loginCopy after login -
NumPy:
- Provides support for numerical computing, including arrays, matrices, and mathematical functions. Essential for performing complex mathematical operations on data.
- Example:
import numpy as np data = np.array([1, 2, 3, 4, 5]) mean = np.mean(data) std_dev = np.std(data)
Copy after login -
Scikit-learn:
- A comprehensive library for machine learning, including data preprocessing, model selection, and evaluation. Useful for building predictive models on engineered data.
- Example:
from sklearn.preprocessing import StandardScaler scaler = StandardScaler() scaled_data = scaler.fit_transform(data)
Copy after login -
PySpark:
- An interface for Apache Spark in Python. Enables distributed data processing and analysis on large datasets.
- Example:
from pyspark.sql import SparkSession spark = SparkSession.builder.appName("MyApp").getOrCreate() # Load data from Parquet df = spark.read.parquet("data.parquet") # Perform aggregation result = df.groupBy("category").sum("amount") # Save results result.write.csv("output.csv")
Copy after login -
Great Expectations:
- A library for data quality validation and documentation. Helps define and enforce expectations on data, ensuring data integrity throughout the data pipeline.
- Example:
from great_expectations.core import ExpectationSuite suite = ExpectationSuite("my_suite") suite.add_expectation("expect_column_values_to_be_between", column="age", min_value=0, max_value=120)
Copy after login -
Apache Airflow:
- A platform for programming workflows as directed acyclic graphs (DAGs). Enables scheduling, monitoring, and triggering data pipelines.
- Example:
import pandas as pd # Load data from CSV df = pd.read_csv('data.csv') # Clean data df = df.dropna() df = df[df['age'] >= 18] # Transform data df['new_column'] = df['column1'] + df['column2'] # Save data to CSV df.to_csv('processed_data.csv', index=False)
Copy after loginCopy after login
3. Real-World Applications
- Recommendation Systems: Building personalized recommendation systems for e-commerce platforms, streaming services, and social media.
- Fraud Detection: Developing models to identify fraudulent transactions in financial institutions and online platforms.
- Customer Churn Prediction: Predicting customer churn to improve customer retention strategies.
- Risk Assessment: Assessing credit risk, insurance risk, and other types of risk in financial and insurance industries.
- Supply Chain Optimization: Optimizing supply chains by analyzing historical data and predicting future demand.
- Internet of Things (IoT): Processing and analyzing data from IoT devices to gain insights into various domains, such as smart homes, smart cities, and industrial automation.
4. Best Practices
- Modularization: Break down complex data pipelines into smaller, reusable modules for better maintainability and testability.
- Testing: Write unit tests and integration tests to ensure the correctness and reliability of data pipelines.
- Documentation: Document data pipelines, including data sources, transformations, and expected outputs.
- Version Control: Use Git or other version control systems to track changes to code and data.
- Continuous Integration and Continuous Delivery (CI/CD): Automate the build, test, and deployment of data pipelines.
5. Future Trends
- Cloud Computing: Increasing adoption of cloud platforms like AWS, Azure, and GCP for data storage, processing, and analysis.
- Machine Learning Operations (MLOps): Integrating machine learning models into production data pipelines, ensuring model reliability and maintainability.
- Serverless Computing: Utilizing serverless functions for on-demand data processing and analysis.
- Data Observability: Gaining real-time insights into the health and performance of data pipelines.
- Edge Computing: Processing data closer to the source to reduce latency and improve real-time responsiveness.
Conclusion
Data engineering with Python is a dynamic and rapidly evolving field. By mastering the core concepts, libraries, and best practices outlined in this article, you can build robust and scalable data pipelines that unlock valuable insights from data. As the volume and complexity of data continue to grow, the role of data engineers will become even more crucial in driving data-driven decision-making across various industries.
Further Learning
- Online Courses: Platforms like Coursera, edX, and DataCamp offer numerous courses on data engineering with Python.
- Books: Explore books like "Data Engineering on AWS" and "Designing Data-Intensive Applications" for in-depth knowledge.
- Community: Engage with the data engineering community through online forums, meetups, and conferences.
Hope this provides a foundational understanding of data engineering with Python. By continuously learning and adapting to the latest advancements in the field, you can excel as a data engineer and contribute to the success of data-driven organizations.
note: AI-assisted content
The above is the detailed content of Diving Deep into Data Engineering with Python: A Comprehensive Guide. For more information, please follow other related articles on the PHP Chinese website!

Hot AI Tools

Undresser.AI Undress
AI-powered app for creating realistic nude photos

AI Clothes Remover
Online AI tool for removing clothes from photos.

Undress AI Tool
Undress images for free

Clothoff.io
AI clothes remover

Video Face Swap
Swap faces in any video effortlessly with our completely free AI face swap tool!

Hot Article

Hot Tools

Notepad++7.3.1
Easy-to-use and free code editor

SublimeText3 Chinese version
Chinese version, very easy to use

Zend Studio 13.0.1
Powerful PHP integrated development environment

Dreamweaver CS6
Visual web development tools

SublimeText3 Mac version
God-level code editing software (SublimeText3)

Hot Topics










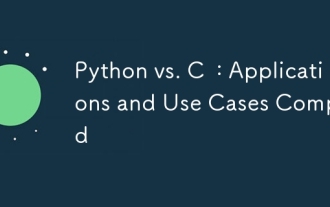
Python is suitable for data science, web development and automation tasks, while C is suitable for system programming, game development and embedded systems. Python is known for its simplicity and powerful ecosystem, while C is known for its high performance and underlying control capabilities.
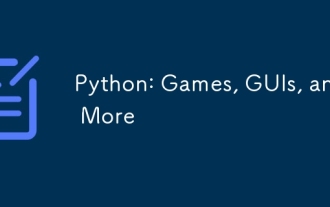
Python excels in gaming and GUI development. 1) Game development uses Pygame, providing drawing, audio and other functions, which are suitable for creating 2D games. 2) GUI development can choose Tkinter or PyQt. Tkinter is simple and easy to use, PyQt has rich functions and is suitable for professional development.
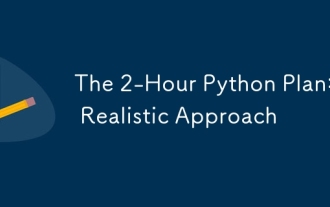
You can learn basic programming concepts and skills of Python within 2 hours. 1. Learn variables and data types, 2. Master control flow (conditional statements and loops), 3. Understand the definition and use of functions, 4. Quickly get started with Python programming through simple examples and code snippets.
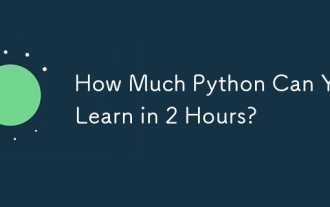
You can learn the basics of Python within two hours. 1. Learn variables and data types, 2. Master control structures such as if statements and loops, 3. Understand the definition and use of functions. These will help you start writing simple Python programs.
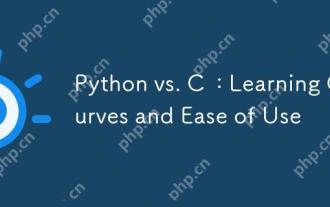
Python is easier to learn and use, while C is more powerful but complex. 1. Python syntax is concise and suitable for beginners. Dynamic typing and automatic memory management make it easy to use, but may cause runtime errors. 2.C provides low-level control and advanced features, suitable for high-performance applications, but has a high learning threshold and requires manual memory and type safety management.
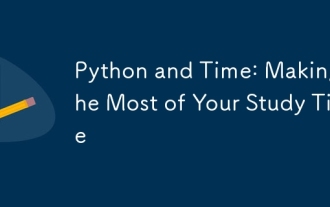
To maximize the efficiency of learning Python in a limited time, you can use Python's datetime, time, and schedule modules. 1. The datetime module is used to record and plan learning time. 2. The time module helps to set study and rest time. 3. The schedule module automatically arranges weekly learning tasks.
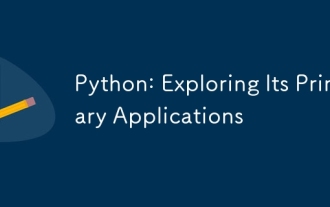
Python is widely used in the fields of web development, data science, machine learning, automation and scripting. 1) In web development, Django and Flask frameworks simplify the development process. 2) In the fields of data science and machine learning, NumPy, Pandas, Scikit-learn and TensorFlow libraries provide strong support. 3) In terms of automation and scripting, Python is suitable for tasks such as automated testing and system management.
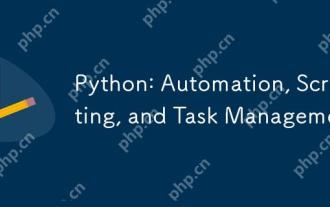
Python excels in automation, scripting, and task management. 1) Automation: File backup is realized through standard libraries such as os and shutil. 2) Script writing: Use the psutil library to monitor system resources. 3) Task management: Use the schedule library to schedule tasks. Python's ease of use and rich library support makes it the preferred tool in these areas.
