Improving memory efficiency in a working interpreter
Lifetimes are a fascinating feature of Rust and the human experience. This is a technical blog, so let’s focus on the former. I was admittedly a slow adopter for leveraging lifetimes to safely borrow data in Rust. In the treewalk implementation of Memphis, my Python interpreter written in Rust, I hardly leverage lifetimes (by cloning incessantly) and I repeatedly elude the borrow checker (by using interior mutability, also incessantly) whenever possible.
My fellow Rustaceans, I am here to today to tell you this ends now. Read my lips……no more shortcuts.
Okay okay, let’s be real. What is a shortcut versus what’s the right way is a matter of priorities and perspective. We’ve all made mistakes, and I’m here to take accountability for mine.
I began writing an interpreter six weeks after I first installed rustc because I have no chill. With that haranguing and posturing out of the way, let’s begin today’s lecture on how we can use lifetimes as our lifeline to improve my bloated interpreter codebase.
Identifying and avoiding cloned data
A Rust lifetime is a mechanism which provides a compile-time guarantee that any references do not outlive the objects to which they refer. They allow us to avoid the “dangling pointer” problem from C and C .
This is assuming you leverage them at all! Cloning is a convenient workaround when you want to avoid the complexities associated with managing lifetimes, though the downside is increased memory usage and a slight delay related to each time data is copied.
Using lifetimes also forces you to think more idiomatically about owners and borrowing in Rust, which I was eager to do.
I chose my first candidate as the tokens from a Python input file. My original implementation, which relied heavily on ChatGPT guidance while I was sitting on Amtrak, used this flow:
- we pass our Python text to a Builder
- the Builder creates a Lexer, which tokenizes the input stream
- the Builder then creates a Parser, which clones the token stream to hold its own copy
- the Builder is used to create an Interpreter, which repeatedly asks the Parser for its next parsed statement and evaluates it until we reach the end of the token stream
The convenient aspect of cloning the token stream is that the Lexer was free to be dropped after step 3. By updating my architecture to have the Lexer own the tokens and the Parser just borrow them, the Lexer would now be required to stay alive much longer. Rust lifetimes would guarantee this for us: as long as the Parser existed holding a reference to a borrowed token, the compiler would guarantee that the Lexer which own those tokens still existed, ensuring a valid reference.
Like all code always, this ended up being a bigger change than I expected. Let’s see why!
The new parser
Before updating the Parser to borrow the tokens from the Lexer, it looked like this. The two fields of interest for today’s discussion are tokens and current_token. We have no idea how large the Vec
pub struct Parser { state: Container<State>, tokens: Vec<Token>, current_token: Token, position: usize, line_number: usize, delimiter_depth: usize, } impl Parser { pub fn new(tokens: Vec<Token>, state: Container<State>) -> Self { let current_token = tokens.first().cloned().unwrap_or(Token::Eof); Parser { state, tokens, current_token, position: 0, line_number: 1, delimiter_depth: 0, } } }
After borrowing the tokens from the Lexer, it looks fairly similar, but now we see a LIFETIME! By connecting tokens to the lifetime 'a, the Rust compiler will not allow the owner of the tokens (which is our Lexer) and the tokens themselves to be dropped while our Parser still references them. This feels safe and fancy!
static EOF: Token = Token::Eof; /// A recursive-descent parser which attempts to encode the full Python grammar. pub struct Parser<'a> { state: Container<State>, tokens: &'a [Token], current_token: &'a Token, position: usize, line_number: usize, delimiter_depth: usize, } impl<'a> Parser<'a> { pub fn new(tokens: &'a [Token], state: Container<State>) -> Self { let current_token = tokens.first().unwrap_or(&EOF); Parser { state, tokens, current_token, position: 0, line_number: 1, delimiter_depth: 0, } } }
Another small difference you may notice is this line:
static EOF: Token = Token::Eof;
This is a small optimization that I began considering once my Parser was moving in the direction of “memory-efficient.” Rather than instantiating a new Token::Eof each time the Parser needs to check if it is at the end of the text stream, the new model allowed me to instantiate only a single token and reference &EOF repeatedly.
Again, this is a small optimization, but it speaks to the larger mindset of each piece of data existing only once in memory and every consumer just referencing it when needed, which Rust both encourages you to do and snugly holds your hand along the way.
Speaking of optimization, I really should have benchmarked the memory usage before and after. Since I did not, I have nothing more to say on the matter.
As I alluded to earlier, tying the lifetime of my Lexer and Parser together a large impact on my Builder pattern. Let’s see what that looks like!
The new Builder: MemphisContext
In the flow I described above, remember how I mentioned that the Lexer could be dropped as soon as the Parser created its own copy of the tokens? This had unintentionally influenced the design of my Builder, which was intended to be the component which supports orchestrating Lexer, Parser, and Interpreter interactions, whether you begin with a Python text stream or a path to a Python file.
As you can see below, there are a few other non-ideal aspects to this design:
- needing to call a dangerous downcast method to get the Interpreter.
- why did I think it was okay to return a Parser to every unit test just to then pass it right back into interpreter.run(&mut parser)?!
fn downcast<T: InterpreterEntrypoint + 'static>(input: T) -> Interpreter { let any_ref: &dyn Any = &input as &dyn Any; any_ref.downcast_ref::<Interpreter>().unwrap().clone() } fn init(text: &str) -> (Parser, Interpreter) { let (parser, interpreter) = Builder::new().text(text).build(); (parser, downcast(interpreter)) } #[test] fn function_definition() { let input = r#" def add(x, y): return x + y a = add(2, 3) "#; let (mut parser, mut interpreter) = init(input); match interpreter.run(&mut parser) { Err(e) => panic!("Interpreter error: {:?}", e), Ok(_) => { assert_eq!( interpreter.state.read("a"), Some(ExprResult::Integer(5.store())) ); } } }
Below is the new MemphisContext interface. This mechanism manages the Lexer lifetime internally (to keep our references alive long enough to keep our Parser happy!) and only exposes what is needed to run this test.
pub struct Parser { state: Container<State>, tokens: Vec<Token>, current_token: Token, position: usize, line_number: usize, delimiter_depth: usize, } impl Parser { pub fn new(tokens: Vec<Token>, state: Container<State>) -> Self { let current_token = tokens.first().cloned().unwrap_or(Token::Eof); Parser { state, tokens, current_token, position: 0, line_number: 1, delimiter_depth: 0, } } }
context.run_and_return_interpreter() is still a bit clunky and speaks to another design problem I may tackle down the road: when you run the interpreter, do you want to return only the final return value or something which lets you access arbitrary values from the symbol table? This method opts for the latter approach. I actually think there’s a case to do both, and will keep tweaking my API to allow for this as we go.
Incidentally, this change improved my ability to evaluate an arbitrary piece of Python code. If you’ll recall from my WebAssembly saga, I had to rely on my crosscheck TreewalkAdapter to do that at the time. Now, our Wasm interface is much cleaner.
static EOF: Token = Token::Eof; /// A recursive-descent parser which attempts to encode the full Python grammar. pub struct Parser<'a> { state: Container<State>, tokens: &'a [Token], current_token: &'a Token, position: usize, line_number: usize, delimiter_depth: usize, } impl<'a> Parser<'a> { pub fn new(tokens: &'a [Token], state: Container<State>) -> Self { let current_token = tokens.first().unwrap_or(&EOF); Parser { state, tokens, current_token, position: 0, line_number: 1, delimiter_depth: 0, } } }
The interface context.evaluate_oneshot() returns the expression result rather than a full symbol table. I wonder if there’s a better way to ensure any of the “oneshot” methods can only operate on a context once, ensuring that no consumers use them in a stateful context. I’ll keep simmering on that!
Was this worth it?
Memphis is first-and-foremost a learning exercise, so this was absolutely worth it!
In addition to sharing the tokens between the Lexer and the Parser, I created an interface to evaluate Python code with significantly less boilerplate. While sharing data introduced additional complexity, these changes bring clear benefits: reduced memory usage, improved safety guarantees through stricter lifetime management, and a streamlined API that’s easier to maintain and extend.
I’m choosing to believe this was the right approach, mostly to maintain my self-esteem. Ultimately, I aim to write code that clearly reflects the principles of software and computer engineering. We can now open the Memphis source, point to the single owner of the tokens, and sleep soundly at night!
Subscribe & Save [on nothing]
If you’d like to get more posts like this directly to your inbox, you can subscribe here!
Elsewhere
In addition to mentoring software engineers, I also write about my experience navigating self-employment and late-diagnosed autism. Less code and the same number of jokes.
- Lake-Effect Coffee, Chapter 1 - From Scratch dot org
The above is the detailed content of Improving memory efficiency in a working interpreter. For more information, please follow other related articles on the PHP Chinese website!

Hot AI Tools

Undresser.AI Undress
AI-powered app for creating realistic nude photos

AI Clothes Remover
Online AI tool for removing clothes from photos.

Undress AI Tool
Undress images for free

Clothoff.io
AI clothes remover

Video Face Swap
Swap faces in any video effortlessly with our completely free AI face swap tool!

Hot Article

Hot Tools

Notepad++7.3.1
Easy-to-use and free code editor

SublimeText3 Chinese version
Chinese version, very easy to use

Zend Studio 13.0.1
Powerful PHP integrated development environment

Dreamweaver CS6
Visual web development tools

SublimeText3 Mac version
God-level code editing software (SublimeText3)

Hot Topics










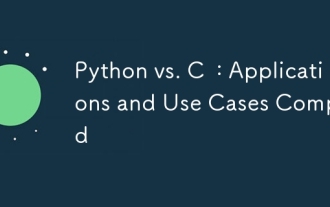
Python is suitable for data science, web development and automation tasks, while C is suitable for system programming, game development and embedded systems. Python is known for its simplicity and powerful ecosystem, while C is known for its high performance and underlying control capabilities.
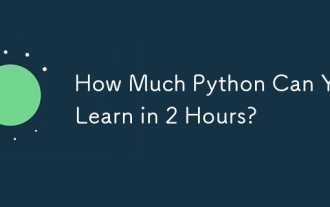
You can learn the basics of Python within two hours. 1. Learn variables and data types, 2. Master control structures such as if statements and loops, 3. Understand the definition and use of functions. These will help you start writing simple Python programs.
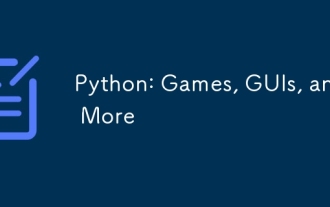
Python excels in gaming and GUI development. 1) Game development uses Pygame, providing drawing, audio and other functions, which are suitable for creating 2D games. 2) GUI development can choose Tkinter or PyQt. Tkinter is simple and easy to use, PyQt has rich functions and is suitable for professional development.
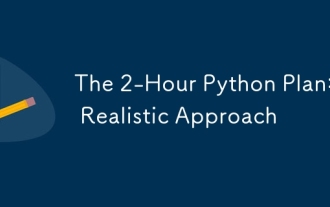
You can learn basic programming concepts and skills of Python within 2 hours. 1. Learn variables and data types, 2. Master control flow (conditional statements and loops), 3. Understand the definition and use of functions, 4. Quickly get started with Python programming through simple examples and code snippets.
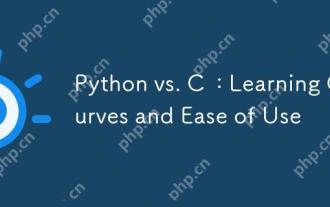
Python is easier to learn and use, while C is more powerful but complex. 1. Python syntax is concise and suitable for beginners. Dynamic typing and automatic memory management make it easy to use, but may cause runtime errors. 2.C provides low-level control and advanced features, suitable for high-performance applications, but has a high learning threshold and requires manual memory and type safety management.
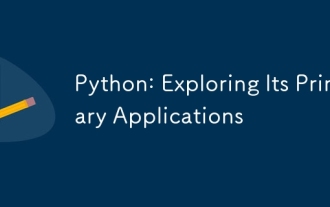
Python is widely used in the fields of web development, data science, machine learning, automation and scripting. 1) In web development, Django and Flask frameworks simplify the development process. 2) In the fields of data science and machine learning, NumPy, Pandas, Scikit-learn and TensorFlow libraries provide strong support. 3) In terms of automation and scripting, Python is suitable for tasks such as automated testing and system management.
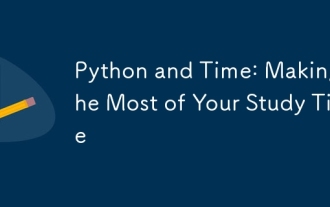
To maximize the efficiency of learning Python in a limited time, you can use Python's datetime, time, and schedule modules. 1. The datetime module is used to record and plan learning time. 2. The time module helps to set study and rest time. 3. The schedule module automatically arranges weekly learning tasks.
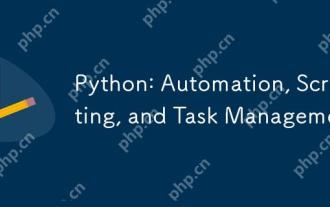
Python excels in automation, scripting, and task management. 1) Automation: File backup is realized through standard libraries such as os and shutil. 2) Script writing: Use the psutil library to monitor system resources. 3) Task management: Use the schedule library to schedule tasks. Python's ease of use and rich library support makes it the preferred tool in these areas.
