


This Runtime Meta-Programming Pattern in Python is Interesting
Background
I’m currently working on a UI framework built on top of Pyodide, called Zenaura. Recently, I noticed that the builder interface — the main way users create UI elements — was a bit too complex and unappealing. While it does abstract the underlying, more cumbersome interface for interacting with Zenaura's virtual DOM "Node" data structure, it still wasn't satisfactory. I wanted to simplify things and provide users with a cleaner, more intuitive experience, all while laying the groundwork for potentially developing a compiler for a completely new syntax. Something like this:
div(attr1=val1, child1, child2, child3)
Problem Statement
The current builder interface is too low-level and user-unfriendly. Users shouldn’t have to interact with something like this:
builder = Builder(name__) if children: builder.with_children(*children) if attributes: builder.with_attributes(**attributes) if text: builder.with_text(text) # print("data", builder.node.children, builder.node.attributes) return builder.build()
Instead, they should be able to use a cleaner, more readable syntax like:
div(id="some-id", h1("text"), p("text"))
Looking at the MDN docs, there are 91 HTML tags, with the possibility of additions or deprecations. I initially considered dynamically generating the code to simplify this process, but while it works, it's not the most practical solution. The primary goal was to show docstrings whenever a user calls a function, but the dynamically generated approach introduces some challenges, such as a lack of auto-completion.
The Dynamic Approach
Here’s the dynamically generated code that I experimented with:
tag_config = { # root elements "html": "nestable", "main": "nestable", "body": "nestable", } tags_factory = { "nestable": lambda name__: f""" {name__} = partial(nestable, "{name__}") {name__}.__doc__ = nestable.__doc__ """, "textable": lambda name__: f""" {name__} = partial(textable, "{name__}") """, "self_closing": lambda name__: f""" {name__} = partial(self_closing, "{name__}") """, "nestable_no_attrs": lambda name__: f""" {name__} = partial(nestable_no_attrs, "{name__}") """ } for k, v in tag_config.items(): exec(tags_factory[v](k), globals())
This works well in terms of functionality but falls short on usability. The main drawback is the lack of autocomplete, since the code is injected at runtime. However, HTML tags themselves are relatively simple, so this isn't as much of a concern for now.
Benefits and Limitations
One of the significant advantages of this approach is flexibility. Supporting or deprecating a html element in Zenaura is as easy as adding or removing a key-value pair from the tag_config dictionary. It’s a straightforward way to adapt to changes in HTML tags over time.
Additionally, the only limitations is with auto-complete and showing doc strings to users, I think this is a tradeoff okay to make since html elements are pretty basic.
However, the trade-off comes in the form of usability: without auto-completion, users may face challenges when interacting with the interface. That said, I believe this is a good starting point for experimenting with new ways to handle tag elements in Zenaura.
The above is the detailed content of This Runtime Meta-Programming Pattern in Python is Interesting. For more information, please follow other related articles on the PHP Chinese website!

Hot AI Tools

Undresser.AI Undress
AI-powered app for creating realistic nude photos

AI Clothes Remover
Online AI tool for removing clothes from photos.

Undress AI Tool
Undress images for free

Clothoff.io
AI clothes remover

Video Face Swap
Swap faces in any video effortlessly with our completely free AI face swap tool!

Hot Article

Hot Tools

Notepad++7.3.1
Easy-to-use and free code editor

SublimeText3 Chinese version
Chinese version, very easy to use

Zend Studio 13.0.1
Powerful PHP integrated development environment

Dreamweaver CS6
Visual web development tools

SublimeText3 Mac version
God-level code editing software (SublimeText3)

Hot Topics










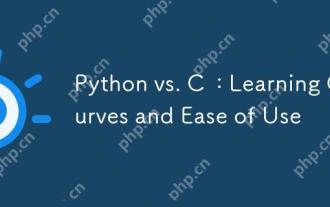
Python is easier to learn and use, while C is more powerful but complex. 1. Python syntax is concise and suitable for beginners. Dynamic typing and automatic memory management make it easy to use, but may cause runtime errors. 2.C provides low-level control and advanced features, suitable for high-performance applications, but has a high learning threshold and requires manual memory and type safety management.
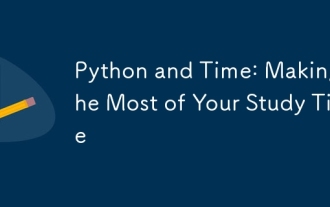
To maximize the efficiency of learning Python in a limited time, you can use Python's datetime, time, and schedule modules. 1. The datetime module is used to record and plan learning time. 2. The time module helps to set study and rest time. 3. The schedule module automatically arranges weekly learning tasks.
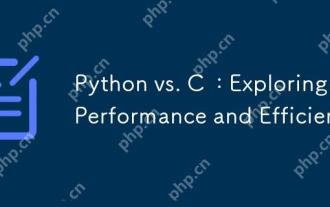
Python is better than C in development efficiency, but C is higher in execution performance. 1. Python's concise syntax and rich libraries improve development efficiency. 2.C's compilation-type characteristics and hardware control improve execution performance. When making a choice, you need to weigh the development speed and execution efficiency based on project needs.
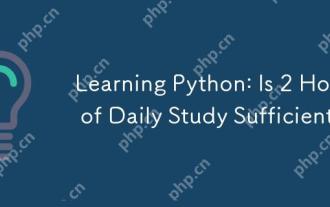
Is it enough to learn Python for two hours a day? It depends on your goals and learning methods. 1) Develop a clear learning plan, 2) Select appropriate learning resources and methods, 3) Practice and review and consolidate hands-on practice and review and consolidate, and you can gradually master the basic knowledge and advanced functions of Python during this period.
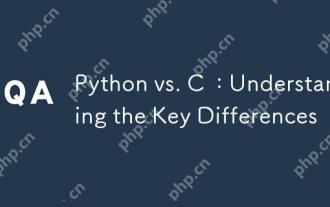
Python and C each have their own advantages, and the choice should be based on project requirements. 1) Python is suitable for rapid development and data processing due to its concise syntax and dynamic typing. 2)C is suitable for high performance and system programming due to its static typing and manual memory management.
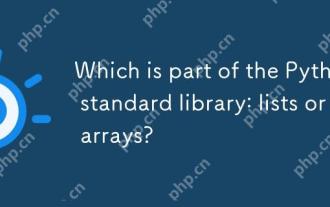
Pythonlistsarepartofthestandardlibrary,whilearraysarenot.Listsarebuilt-in,versatile,andusedforstoringcollections,whereasarraysareprovidedbythearraymoduleandlesscommonlyusedduetolimitedfunctionality.
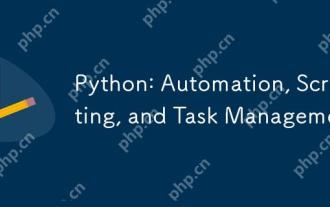
Python excels in automation, scripting, and task management. 1) Automation: File backup is realized through standard libraries such as os and shutil. 2) Script writing: Use the psutil library to monitor system resources. 3) Task management: Use the schedule library to schedule tasks. Python's ease of use and rich library support makes it the preferred tool in these areas.
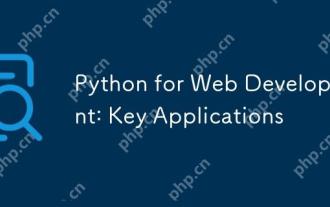
Key applications of Python in web development include the use of Django and Flask frameworks, API development, data analysis and visualization, machine learning and AI, and performance optimization. 1. Django and Flask framework: Django is suitable for rapid development of complex applications, and Flask is suitable for small or highly customized projects. 2. API development: Use Flask or DjangoRESTFramework to build RESTfulAPI. 3. Data analysis and visualization: Use Python to process data and display it through the web interface. 4. Machine Learning and AI: Python is used to build intelligent web applications. 5. Performance optimization: optimized through asynchronous programming, caching and code
